Learning Continuous High-Dimensional Models Using Mutual Information And Copula Bayesian Networks
THIRTY-FIFTH AAAI CONFERENCE ON ARTIFICIAL INTELLIGENCE, THIRTY-THIRD CONFERENCE ON INNOVATIVE APPLICATIONS OF ARTIFICIAL INTELLIGENCE AND THE ELEVENTH SYMPOSIUM ON EDUCATIONAL ADVANCES IN ARTIFICIAL INTELLIGENCE(2021)
摘要
We propose a new framework to learn non-parametric graphical models from continuous observational data. Our method is based on concepts from information theory in order to discover independences and causality between variables: the conditional and multivariate mutual information (such as (Verny et al. 2017) for discrete models). To estimate these quantities, we propose non-parametric estimators relying on the Bernstein copula and that are constructed by exploiting the relation between the mutual information and the copula entropy (Ma and Sun 2011; Belalia et al. 2017). To our knowledge, this relation is only documented for the bivariate case and, for the need of our algorithms, is here extended to the conditional and multivariate mutual information. This framework leads to a new algorithm to learn continuous non-parametric Bayesian networks. Moreover, we use this estimator to speed up the BIC algorithm proposed in (Elidan 2010) by taking advantage of the decomposition of the likelihood function in a sum of mutual information (Koller and Friedman 2009). Finally, our method is compared in terms of performances and complexity with other state of the art techniques to learn Copula Bayesian Networks and shows superior results. In particular, it needs less data to recover the original structure and generalizes better on data that are not sampled from Gaussian distributions.
更多查看译文
关键词
copula bayesian networks,mutual information,models,learning,high-dimensional
AI 理解论文
溯源树
样例
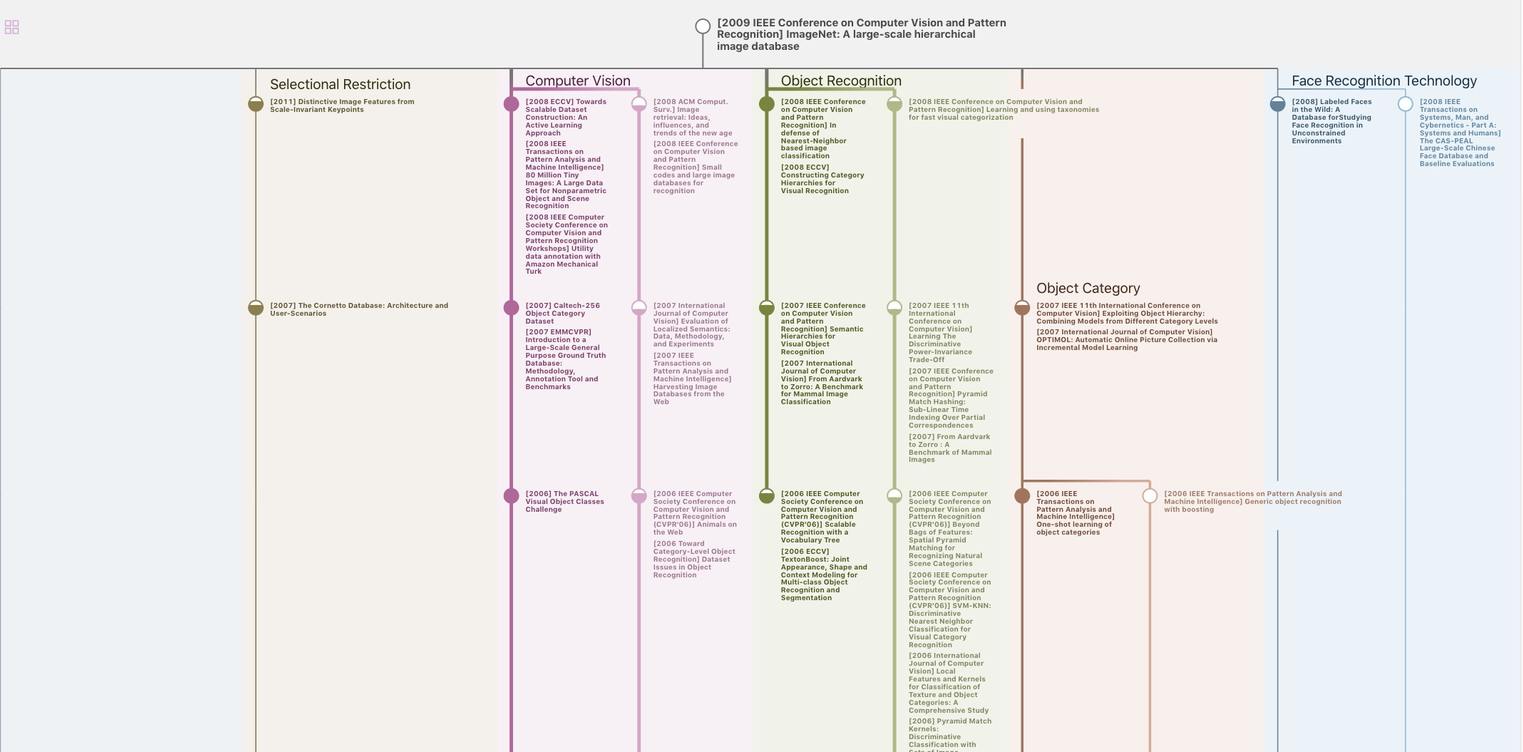
生成溯源树,研究论文发展脉络
Chat Paper
正在生成论文摘要