Joint-Label Learning By Dual Augmentation For Time Series Classification
THIRTY-FIFTH AAAI CONFERENCE ON ARTIFICIAL INTELLIGENCE, THIRTY-THIRD CONFERENCE ON INNOVATIVE APPLICATIONS OF ARTIFICIAL INTELLIGENCE AND THE ELEVENTH SYMPOSIUM ON EDUCATIONAL ADVANCES IN ARTIFICIAL INTELLIGENCE(2021)
摘要
Recently, deep neural networks (DNNs) have achieved excellent performance on time series classification. However, DNNs require large amounts of labeled data for supervised training. Although data augmentation can alleviate this problem, the standard approach assigns the same label to all augmented samples from the same source. This leads to the expansion of the data distribution such that the classification boundaries may be even harder to determine. In this paper, we propose Joint-label learning by Dual Augmentation (JobDA), which can enrich the training samples without expanding the distribution of the original data. Instead, we apply simple transformations to the time series and give these modified time series new labels, so that the model has to distinguish between these and the original data, as well as separating the original classes. This approach sharpens the boundaries around the original time series, and results in superior classification performance. We use Time Series Warping for our transformations: We shrink and stretch different regions of the original time series, like a fun-house mirror. Experiments conducted on extensive time-series datasets show that JobDA can improve the model performance on small datasets. More-over, we verify that JobDA has better generalization ability compared with conventional data augmentation, and the visualization analysis further demonstrates that JobDA can learn more compact clusters.
更多查看译文
AI 理解论文
溯源树
样例
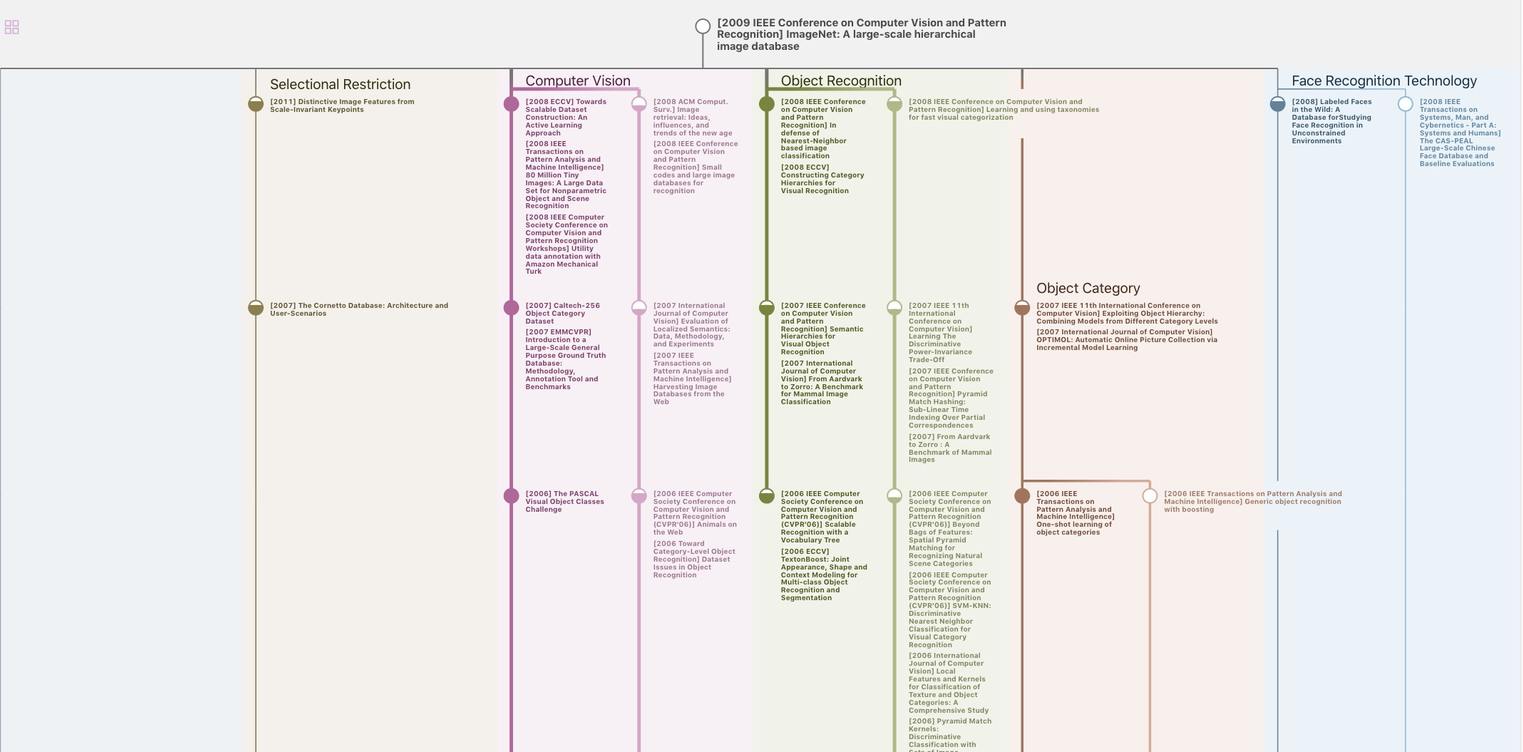
生成溯源树,研究论文发展脉络
Chat Paper
正在生成论文摘要