Visual Comfort Aware-Reinforcement Learning For Depth Adjustment Of Stereoscopic 3d Images
THIRTY-FIFTH AAAI CONFERENCE ON ARTIFICIAL INTELLIGENCE, THIRTY-THIRD CONFERENCE ON INNOVATIVE APPLICATIONS OF ARTIFICIAL INTELLIGENCE AND THE ELEVENTH SYMPOSIUM ON EDUCATIONAL ADVANCES IN ARTIFICIAL INTELLIGENCE(2021)
摘要
Depth adjustment aims to enhance the visual experience of stereoscopic 3D (S3D) images, which accompanied with improving visual comfort and depth perception. For a human expert, the depth adjustment procedure is a sequence of iterative decision making The human expert iteratively adjusts the depth until he is satisfied with the both levels of visual comfort and the perceived depth. In this work, we present a novel deep reinforcement learning (DRL)-based approach for depth adjustment named VCA-RL (Visual Comfort Aware Reinforcement Learning) to explicitly model human sequential decision making in depth editing operations. We formulate the depth adjustment process as a Markov decision process where actions are defined as camera movement operations to control the distance between the left and right cameras. Our agent is trained based on the guidance of an objective visual comfort assessment metric to learn the optimal sequence of camera movement actions in terms of perceptual aspects in stereoscopic viewing. With extensive experiments and user studies, we show the effectiveness of our VCA-RL model on three different S3D databases.
更多查看译文
关键词
stereoscopic 3d images,depth adjustment,aware-reinforcement
AI 理解论文
溯源树
样例
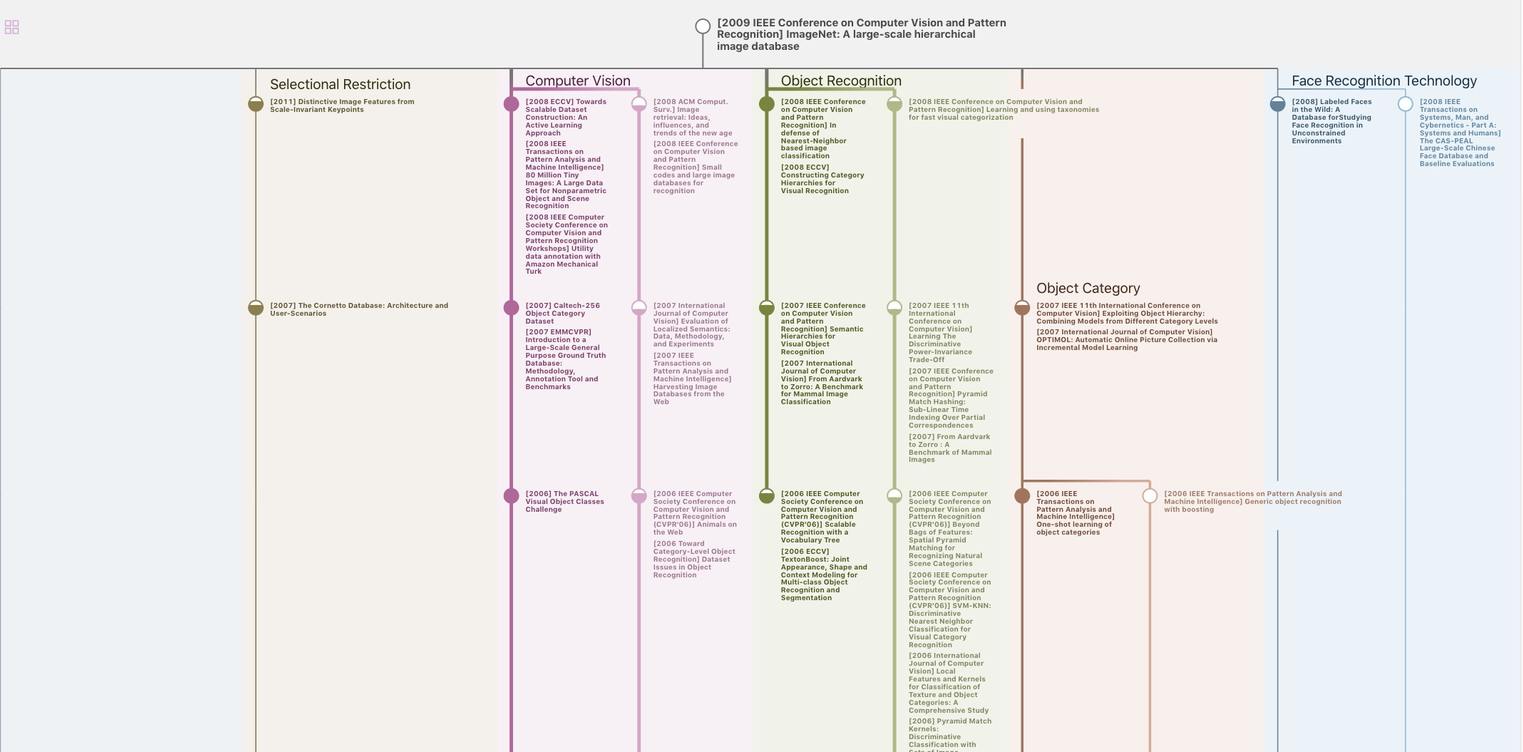
生成溯源树,研究论文发展脉络
Chat Paper
正在生成论文摘要