A Bayesian Approach For Subset Selection In Contextual Bandits
THIRTY-FIFTH AAAI CONFERENCE ON ARTIFICIAL INTELLIGENCE, THIRTY-THIRD CONFERENCE ON INNOVATIVE APPLICATIONS OF ARTIFICIAL INTELLIGENCE AND THE ELEVENTH SYMPOSIUM ON EDUCATIONAL ADVANCES IN ARTIFICIAL INTELLIGENCE(2021)
摘要
Subset selection in Contextual Bandits (CB) is an important task in various applications such as advertisement recommendation. In CB, arms are attached with contexts and thus correlated in the context space. Proper exploration for subset selection in CB should carefully consider the contexts. Previous works mainly concentrate on the best one arm identification in linear bandit problems, where the expected rewards are linearly dependent on the contexts. However, these methods highly rely on linearity, and cannot be easily extended to more general cases. We propose a novel Bayesian approach for subset selection in general CB where the reward functions can be nonlinear. Our method provides a principled way to employ contextual information and efficiently explore the arms. For cases with relatively smooth posteriors, we give theoretical results that are comparable to previous works. For general cases, we provide a calculable approximate variant. Empirical results show the effectiveness of our method on both linear bandits and general CB.
更多查看译文
AI 理解论文
溯源树
样例
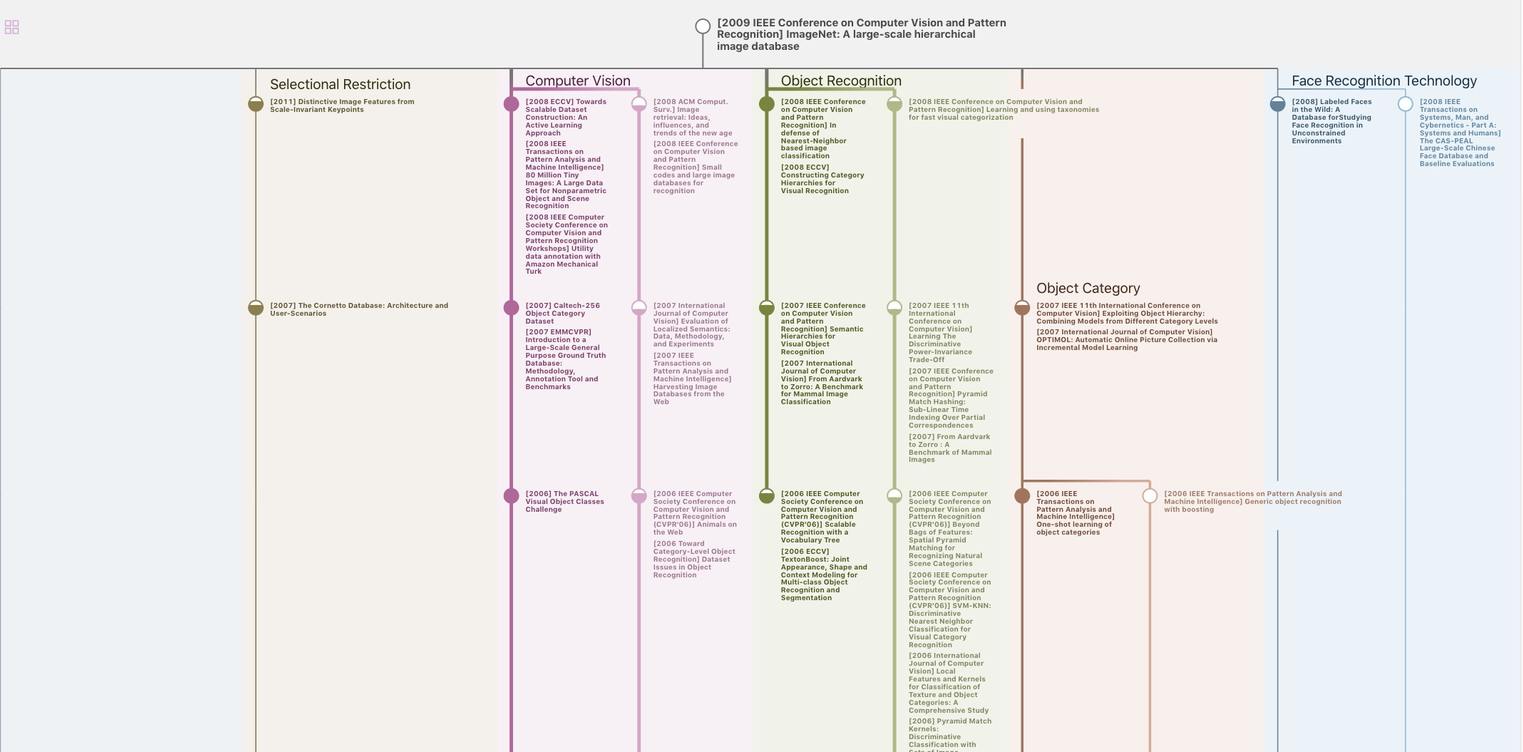
生成溯源树,研究论文发展脉络
Chat Paper
正在生成论文摘要