A Weighted Federated Averaging Framework to Reduce the Negative Influence from the Dishonest Users.
SpaCCS(2020)
摘要
Federated learning becomes popular for it can train an excellent performance global model without exposing clients’ privacy. However, most FL applications failed to consider there exists fake local trained models returned from attackers or dishonest users. Not only would the fake parameters be harmful to the convergence of the global model but also be wasting of other users’ computational resources. In this paper, we propose a framework to grade the users’ credit score based on the performances of the returned local models on the testing dataset. We also consider historical data using the exponential moving average to give a relatively higher weight for the most recent testing results. The experiments show that our system can efficiently and effectively find out the fake local models and then speed up the convergence of the global model.
更多查看译文
关键词
weighted federated averaging framework,negative influence
AI 理解论文
溯源树
样例
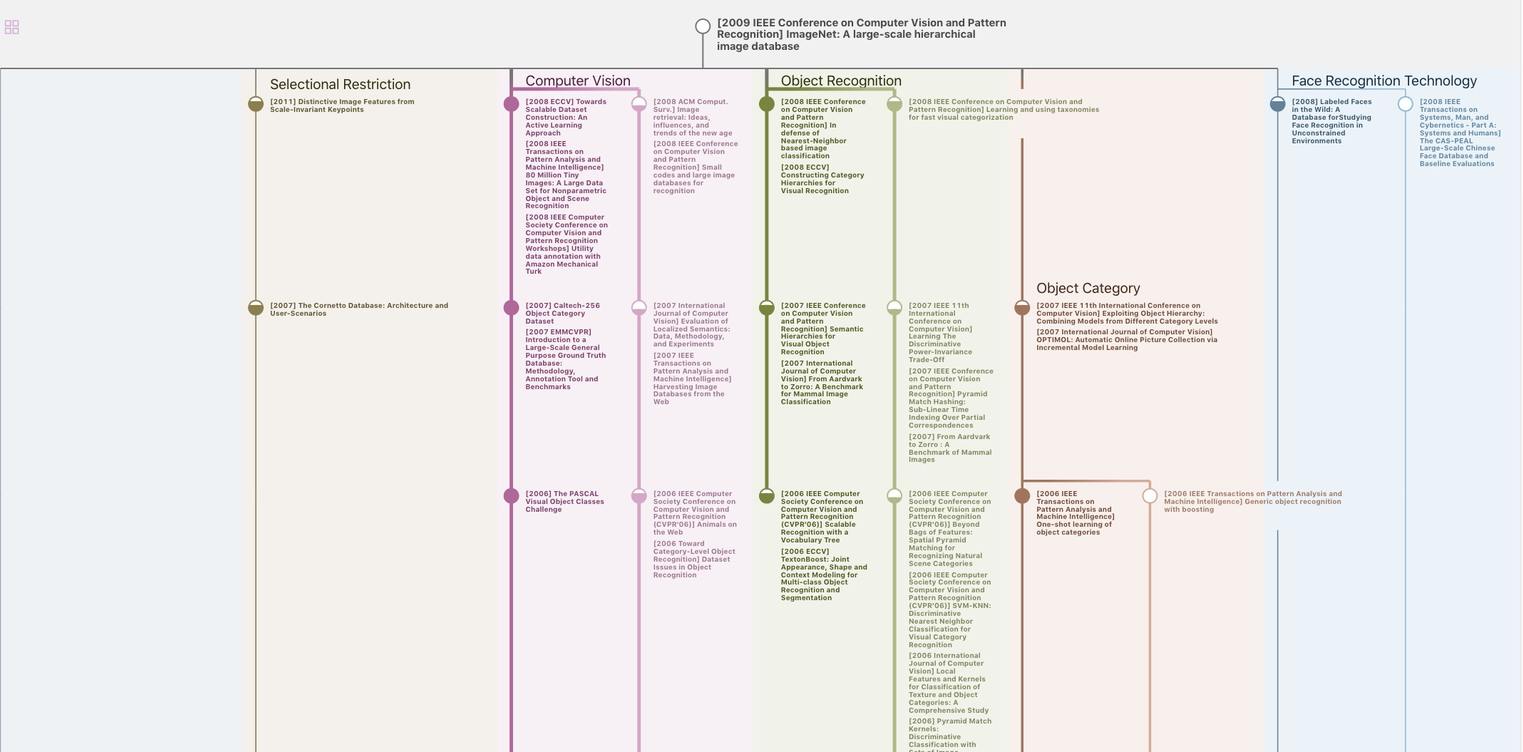
生成溯源树,研究论文发展脉络
Chat Paper
正在生成论文摘要