Koopman operator dynamical models: Learning, analysis and control
Annual Reviews in Control(2021)
摘要
The Koopman operator allows for handling nonlinear systems through a globally linear representation. In general, the operator is infinite-dimensional – necessitating finite approximations – for which there is no overarching framework. Although there are principled ways of learning such finite approximations, they are in many instances overlooked in favor of, often ill-posed and unstructured methods. Also, Koopman operator theory has long-standing connections to known system-theoretic and dynamical system notions that are not universally recognized. Given the former and latter realities, this work aims to bridge the gap between various concepts regarding both theory and tractable realizations. Firstly, we review data-driven representations (both unstructured and structured) for Koopman operator dynamical models, categorizing various existing methodologies and highlighting their differences. Furthermore, we provide concise insight into the paradigm’s relation to system-theoretic notions and analyze the prospect of using the paradigm for modeling control systems. Additionally, we outline the current challenges and comment on future perspectives.
更多查看译文
关键词
Koopman operator,Dynamical models,Representation learning,System analysis,Data-based control
AI 理解论文
溯源树
样例
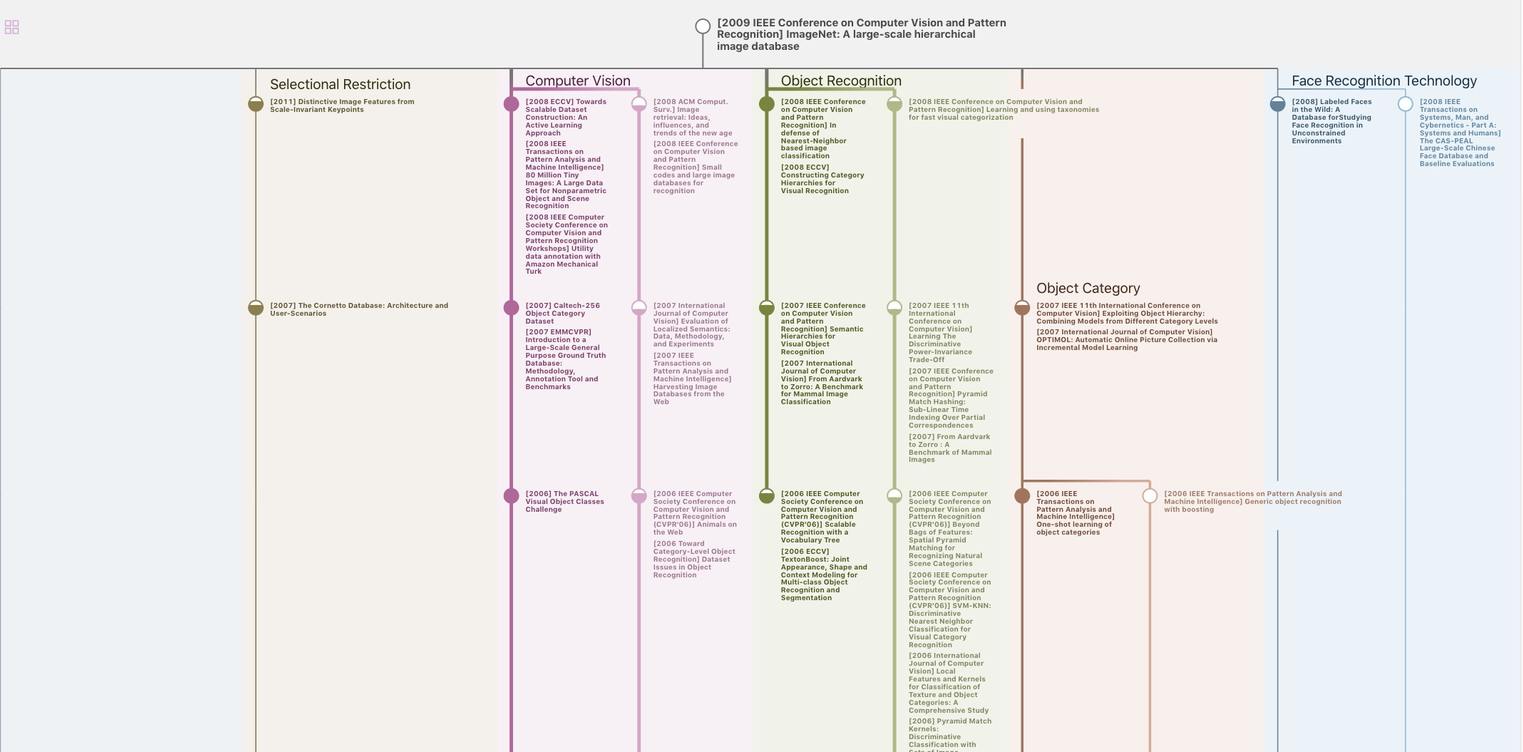
生成溯源树,研究论文发展脉络
Chat Paper
正在生成论文摘要