Federated Learning Improves Site Performance In Multicenter Deep Learning Without Data Sharing
JOURNAL OF THE AMERICAN MEDICAL INFORMATICS ASSOCIATION(2021)
摘要
Objective: To demonstrate enabling multi-institutional training without centralizing or sharing the underlying physical data via federated learning (FL).Materials and Methods: Deep learning models were trained at each participating institution using local clinical data, and an additional model was trained using FL across all of the institutions.Results: We found that the FL model exhibited superior performance and generalizability to the models trained at single institutions, with an overall performance level that was significantly better than that of any of the institutional models alone when evaluated on held-out test sets from each institution and an outside challenge dataset.Discussion: The power of FL was successfully demonstrated across 3 academic institutions while avoiding the privacy risk associated with the transfer and pooling of patient data.Conclusion: Federated learning is an effective methodology that merits further study to enable accelerated development of models across institutions, enabling greater generalizability in clinical use.
更多查看译文
关键词
deep learning, federated learning, privacy, generalizability, prostate
AI 理解论文
溯源树
样例
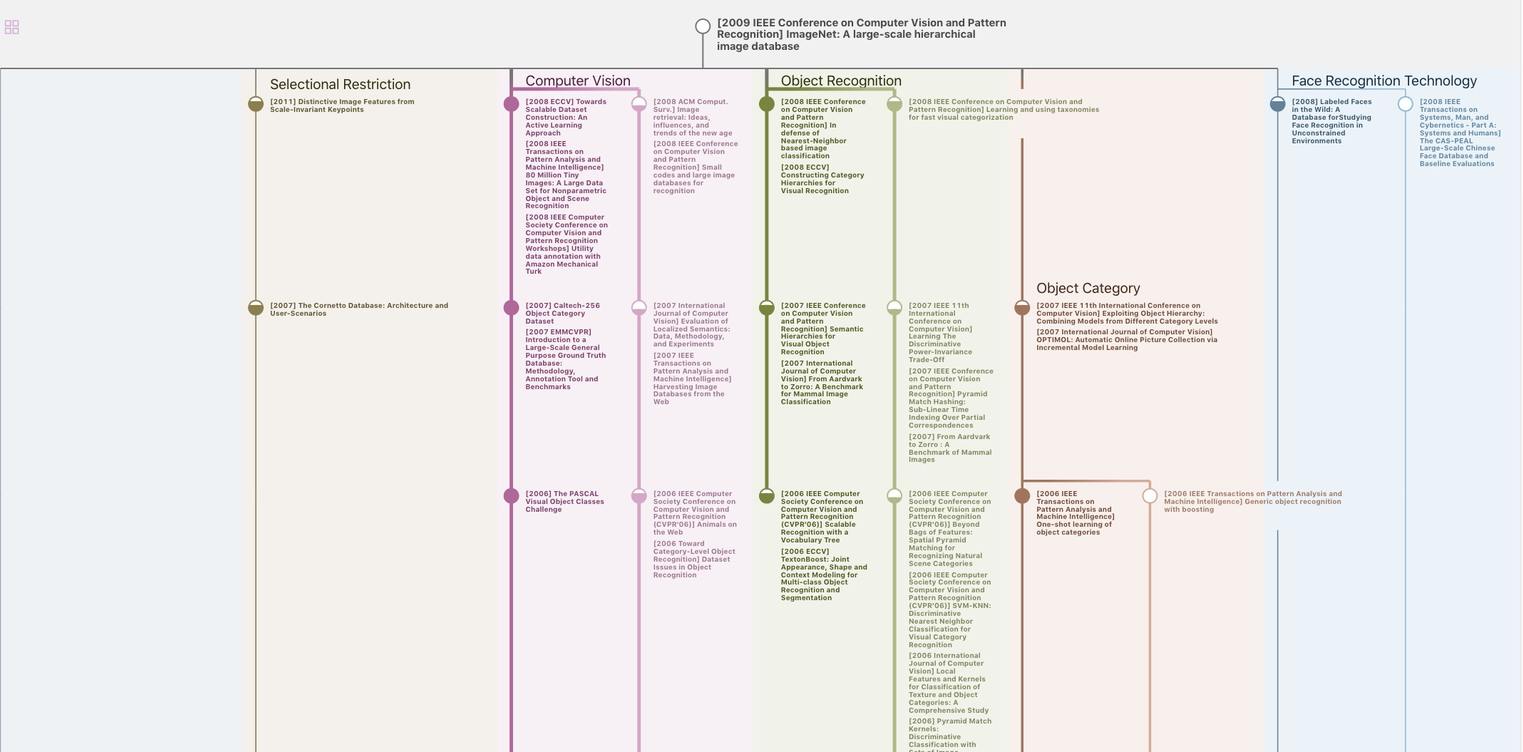
生成溯源树,研究论文发展脉络
Chat Paper
正在生成论文摘要