BeFair: Addressing Fairness in the Banking Sector
arxiv(2021)
摘要
Algorithmic bias mitigation has been one of the most difficult conundrums for the data science community and Machine Learning (ML) experts. Over several years, there have appeared enormous efforts in the field of fairness in ML. Despite the progress toward identifying biases and designing fair algorithms, translating them into the industry remains a major challenge. In this paper, we present the initial results of an industrial open innovation project in the banking sector: we propose a general roadmap for fairness in ML and the implementation of a toolkit called BeFair that helps to identify and mitigate bias. Results show that training a model without explicit constraints may lead to bias exacerbation in the predictions.
更多查看译文
关键词
machine learning, banking, fairness, bias, discrimination
AI 理解论文
溯源树
样例
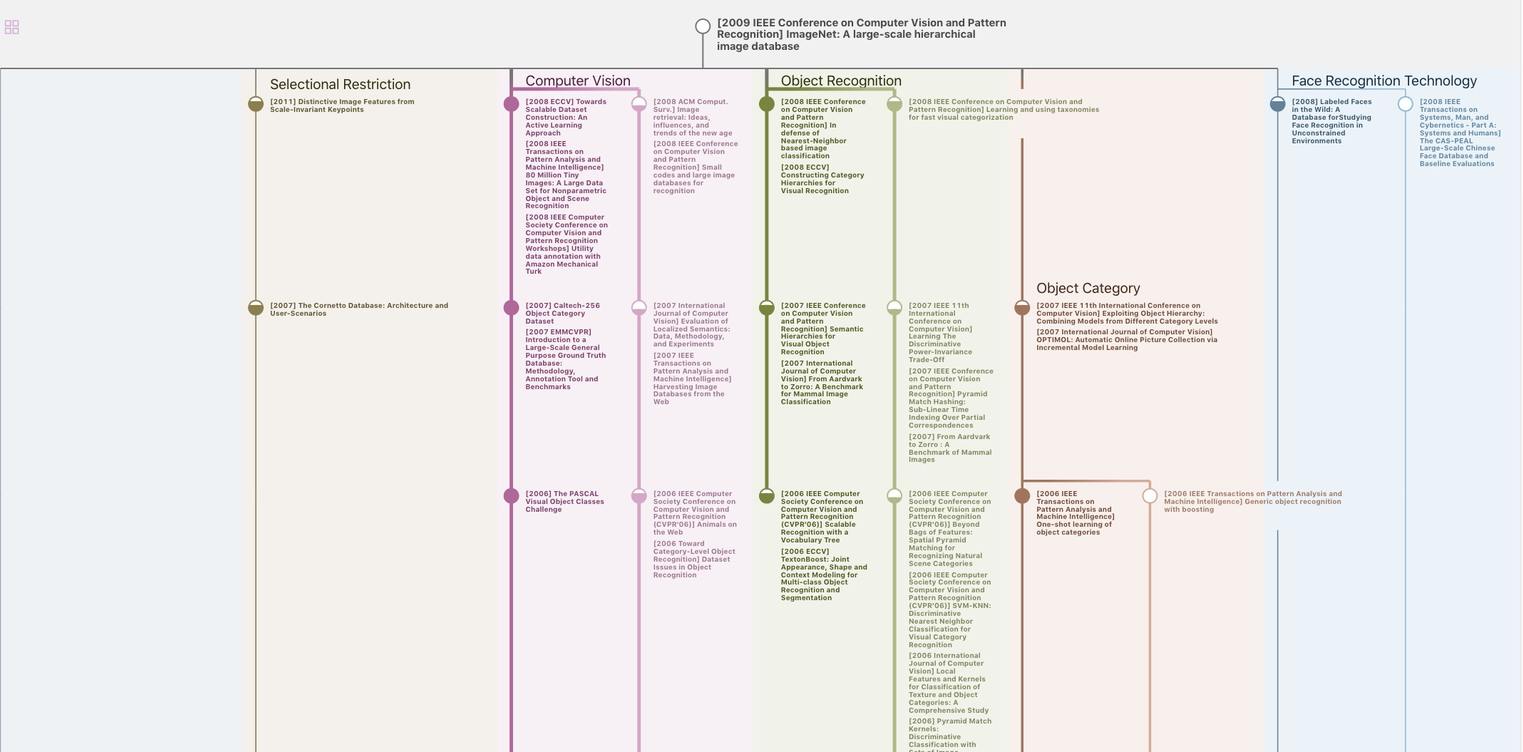
生成溯源树,研究论文发展脉络
Chat Paper
正在生成论文摘要