Personalized Human Activity Recognition Based On Integrated Wearable Sensor And Transfer Learning
SENSORS(2021)
摘要
Human activity recognition (HAR) based on the wearable device has attracted more attention from researchers with sensor technology development in recent years. However, personalized HAR requires high accuracy of recognition, while maintaining the model's generalization capability is a major challenge in this field. This paper designed a compact wireless wearable sensor node, which combines an air pressure sensor and inertial measurement unit (IMU) to provide multi-modal information for HAR model training. To solve personalized recognition of user activities, we propose a new transfer learning algorithm, which is a joint probability domain adaptive method with improved pseudo-labels (IPL-JPDA). This method adds the improved pseudo-label strategy to the JPDA algorithm to avoid cumulative errors due to inaccurate initial pseudo-labels. In order to verify our equipment and method, we use the newly designed sensor node to collect seven daily activities of 7 subjects. Nine different HAR models are trained by traditional machine learning and transfer learning methods. The experimental results show that the multi-modal data improve the accuracy of the HAR system. The IPL-JPDA algorithm proposed in this paper has the best performance among five HAR models, and the average recognition accuracy of different subjects is 93.2%.
更多查看译文
关键词
human activity recognition (HAR), wearable device, air pressure sensor, inertial measurement unit (IMU), transfer learning
AI 理解论文
溯源树
样例
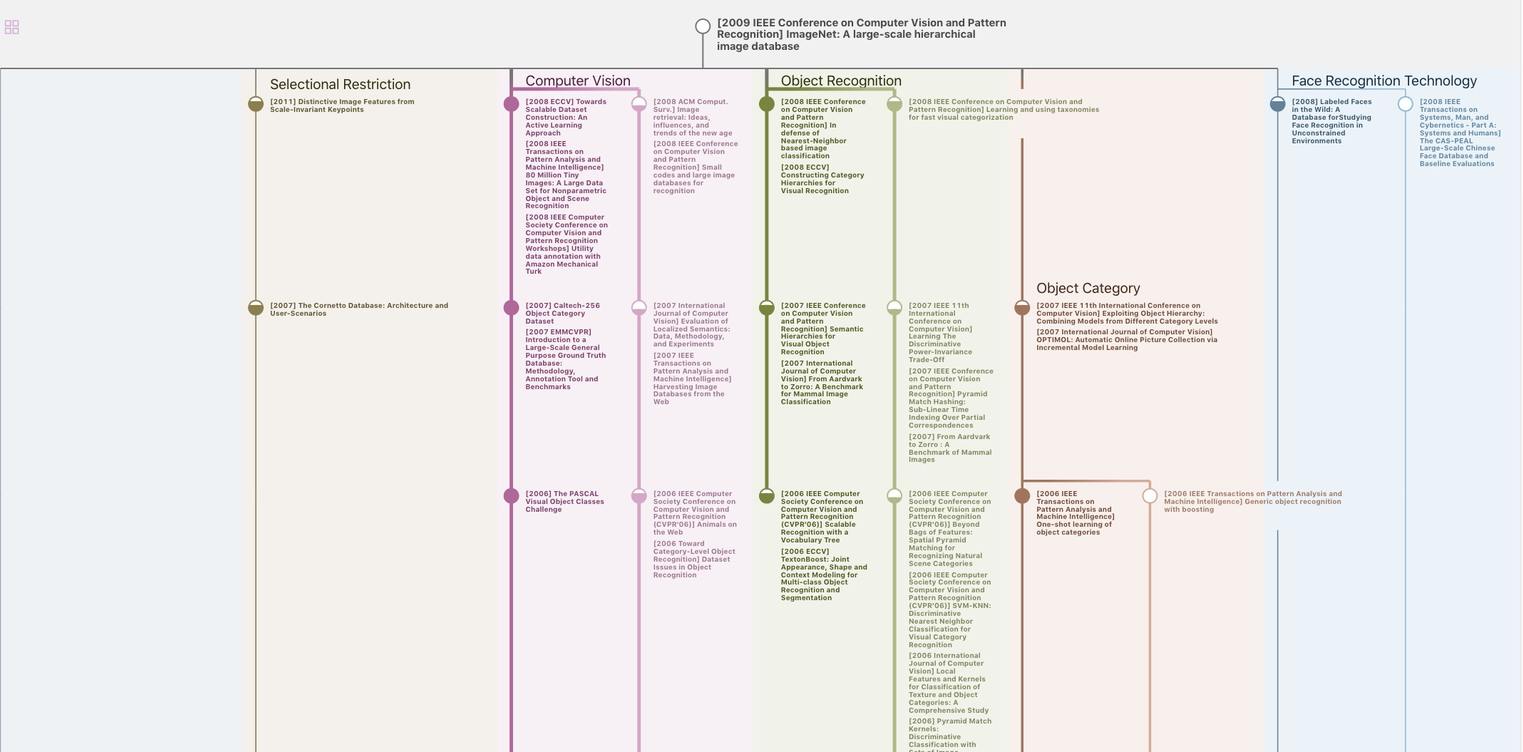
生成溯源树,研究论文发展脉络
Chat Paper
正在生成论文摘要