Model Partition Defense against GAN Attacks on Collaborative Learning via Mobile Edge Computing
GLOBECOM 2020 - 2020 IEEE Global Communications Conference(2020)
Abstract
With growing concerns about privacy issues of machine learning, collaborative learning (CL) is developed to offer on-device training. However, adversarial behaviors of model inversion (MI) are undermining privacy of training data. Specifically, adversaries act as ordinary participants in CL and reproduce private data of a class in training data by training generative adversarial networks (GAN) on the fly, unknowingly. To this end, we design a novel model partition defense, PAMPAS, over user devices and trustworthy edge server to resist GAN attack, and formulate a new optimization problem, TENSOR, to optimize training time. To address the challenges that come with PAMPAS, we propose an algorithm TESLA that yields the optimal solution. Experiment and simulation results manifest that PAMPAS effectively defend GAN attack and TESLA reduces training time by 50% compared with other solutions.
MoreTranslated text
Key words
federated learning,GAN attack,model partition defense,mobile edge network
AI Read Science
Must-Reading Tree
Example
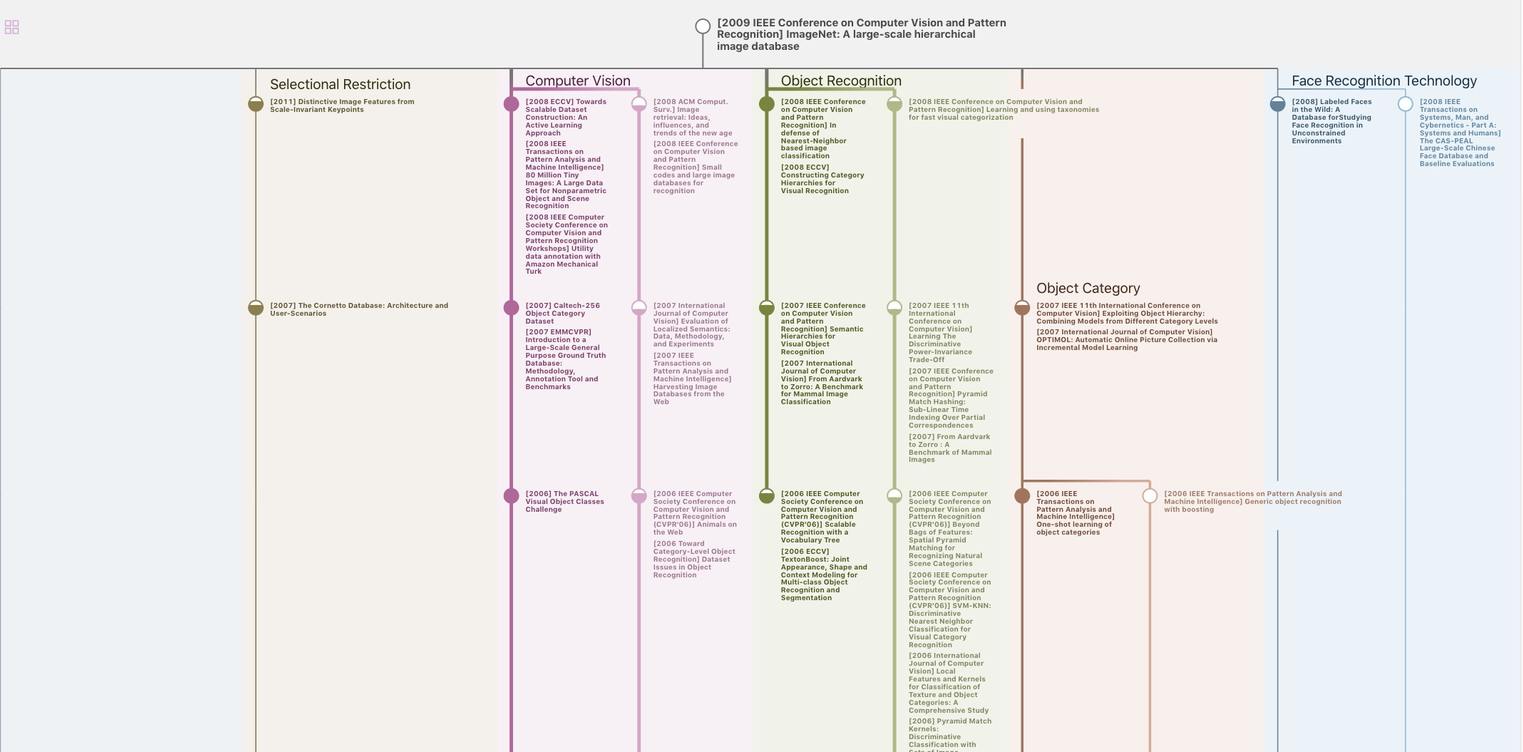
Generate MRT to find the research sequence of this paper
Chat Paper
Summary is being generated by the instructions you defined