Leveraging Shared and Divergent Facial Expression Behavior Between Genders in Deception Detection
2020 15th IEEE International Conference on Automatic Face and Gesture Recognition (FG 2020)(2020)
摘要
While facial expression behavior has been understood as mostly universal between the genders, recent research has highlighted important differences, including the expression of smiles and surprise. Despite such gender differences, studies involving facial expression often have limited sample sizes such that splitting the data set in half to train separate male and female models has been untenable. In order to leverage gender divergent complexity in facial expression models while also using a full dataset to train shared behaviors, we developed GAHL: the Gender-Augmented Hyper-Linear model. GAHL selectively increases non-linear model complexity with regards to gender divergent features. Using both simulated data and data from a study of facial expressions during deception (N=80, >6 hours), we demonstrate that when the facial expression data set size is in the range of N<; 7 5, GAHL outperforms several mainstream machine learning models including logistic regression, decision tree, and SVM with polynomial and radial basis function kernels.
更多查看译文
关键词
facial expression models,GAHL,nonlinear model complexity,machine learning,divergent facial expression,deception detection,facial expression behavior,gender differences,male separation,female separation,shared facial expression,gender augmented hyper-linear,gender divergent complexity,gender divergent features
AI 理解论文
溯源树
样例
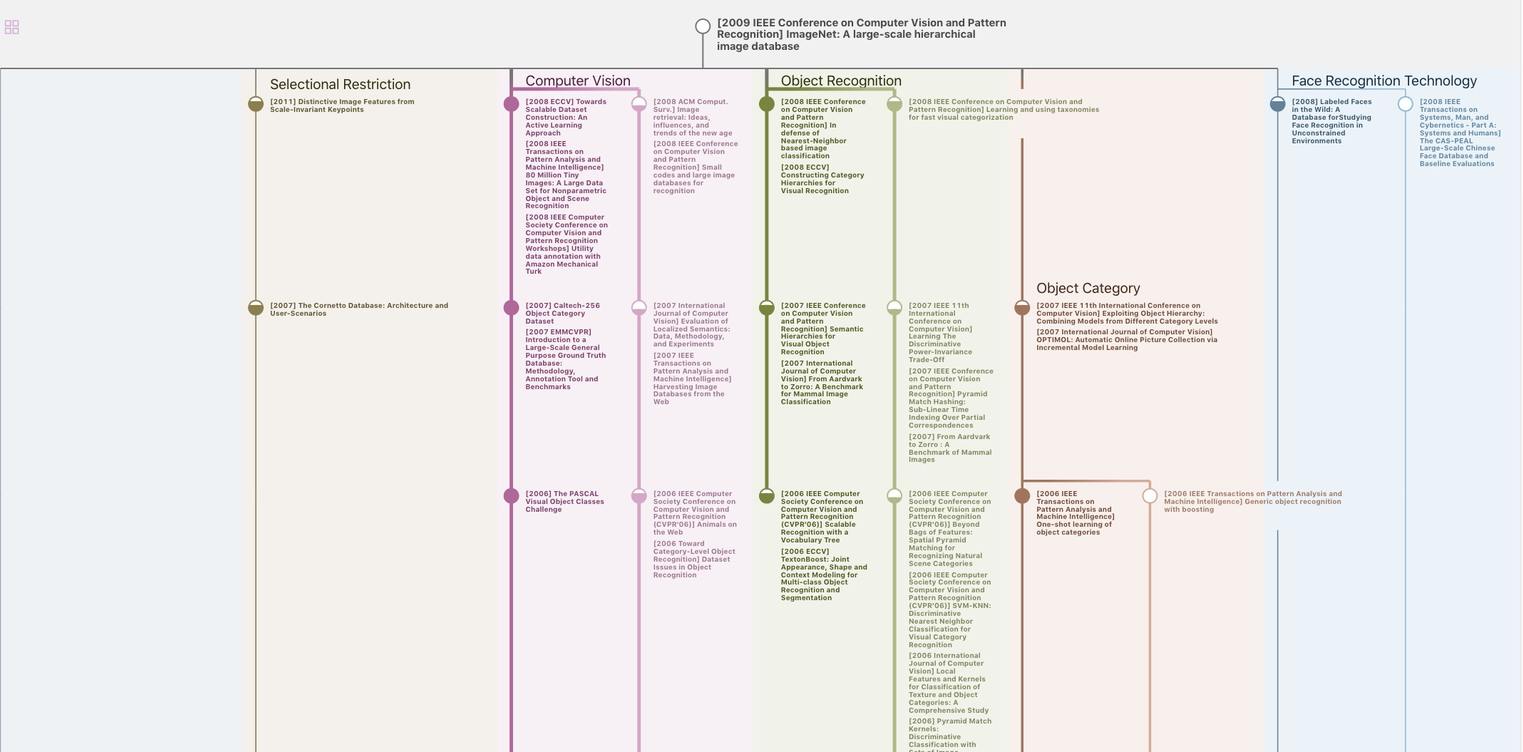
生成溯源树,研究论文发展脉络
Chat Paper
正在生成论文摘要