Feature Selection For Zero-Shot Gesture Recognition
2020 15TH IEEE INTERNATIONAL CONFERENCE ON AUTOMATIC FACE AND GESTURE RECOGNITION (FG 2020)(2020)
摘要
Existing classification techniques assign a predetermined categorical label to each sample and can not recognize the new categories that might appear after the training stage. This limitation has led to the advent of new paradigms in machine learning such as zero-shot learning (ZSL). ZSL aims to recognize unseen categories by having a high-level description of them. While deep learning has pushed the limits of ZSL for object recognition, ZSL for temporal problems such as unfamiliar gesture recognition (ZSGL) remain unexplored. Previous attempts to address ZSGL were focused on the creation of gesture attributes, attribute-based datasets, and algorithmic improvements, and there is little or no research concerned with feature selection for ZSGL problems. It is indisputable that deep learning has obviated the need for feature engineering for the problems with large datasets. However, when the data is scarce, it is critical to leverage the domain information to create discriminative input features. The main goal of this work is to study the effect of three different feature extraction techniques (raw features, engineered features, and deep learning features) on the performance of ZSGL. Next, we propose a new approach for ZSGL that jointly minimizes the reconstruction loss, semantic and classification losses. Our methodology yields an unseen class accuracy of (38%) which parallels the accuracies obtained through stateof-the-art approaches.
更多查看译文
关键词
gesture recognition,zero shot learning,attribute learning,and spontaneous gestures
AI 理解论文
溯源树
样例
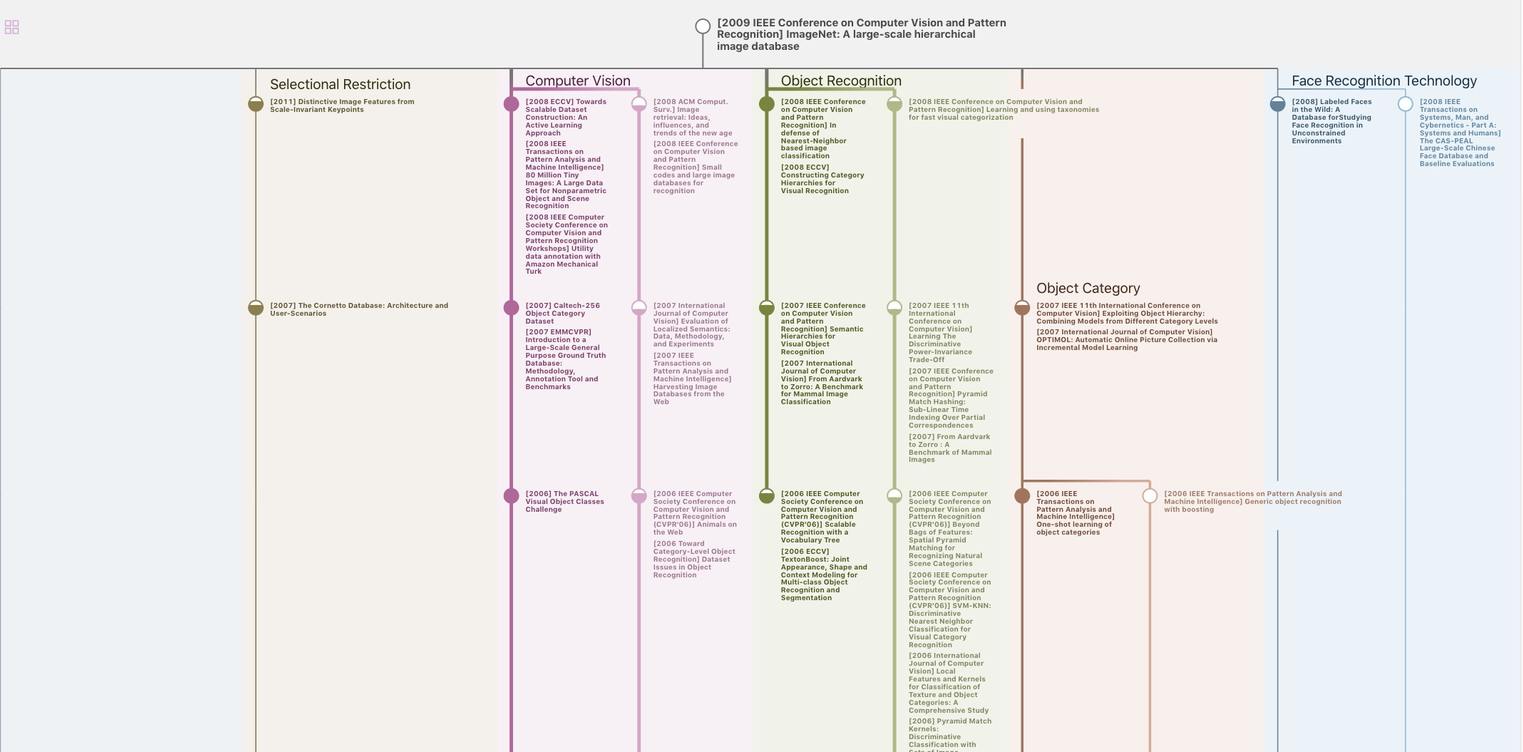
生成溯源树,研究论文发展脉络
Chat Paper
正在生成论文摘要