Optimal robust mean and location estimation via convex programs with respect to any pseudo-norms
Probability Theory and Related Fields(2022)
摘要
We consider the problem of robust mean and location estimation with respect to any pseudo-norm of the form x∈ℝ^d↦ x _S = sup _v∈ S < v,x > where S is any symmetric subset of ℝ^d . We show that the deviation-optimal minimax sub-Gaussian rate for confidence 1-δ is max( ℓ ^*(Σ ^1/2S)/√(N), sup _v∈ SΣ ^1/2v _2√(log (1/δ )/N)) where ℓ ^*(Σ ^1/2S) is the Gaussian mean width of Σ ^1/2S and Σ the covariance of the data. This improves the entropic minimax lower bound from Lugosi and Mendelson (Probab Theory Relat Fields 175(3–4):957–973, 2019) and closes the gap characterized by Sudakov’s inequality between the entropy and the Gaussian mean width for this problem. This shows that the right statistical complexity measure for the mean estimation problem is the Gaussian mean width. We also show that this rate can be achieved by a solution to a convex optimization problem in the adversarial and L_2 heavy-tailed setup by considering minimum of some Fenchel–Legendre transforms constructed using the median-of-means principle. We finally show that this rate may also be achieved in situations where there is not even a first moment but a location parameter exists.
更多查看译文
关键词
Robustness,Entropy,Gaussian mean widths,Heavy-tailed data,Location parameter,Median-of-means,Fenchel–Legendre transform
AI 理解论文
溯源树
样例
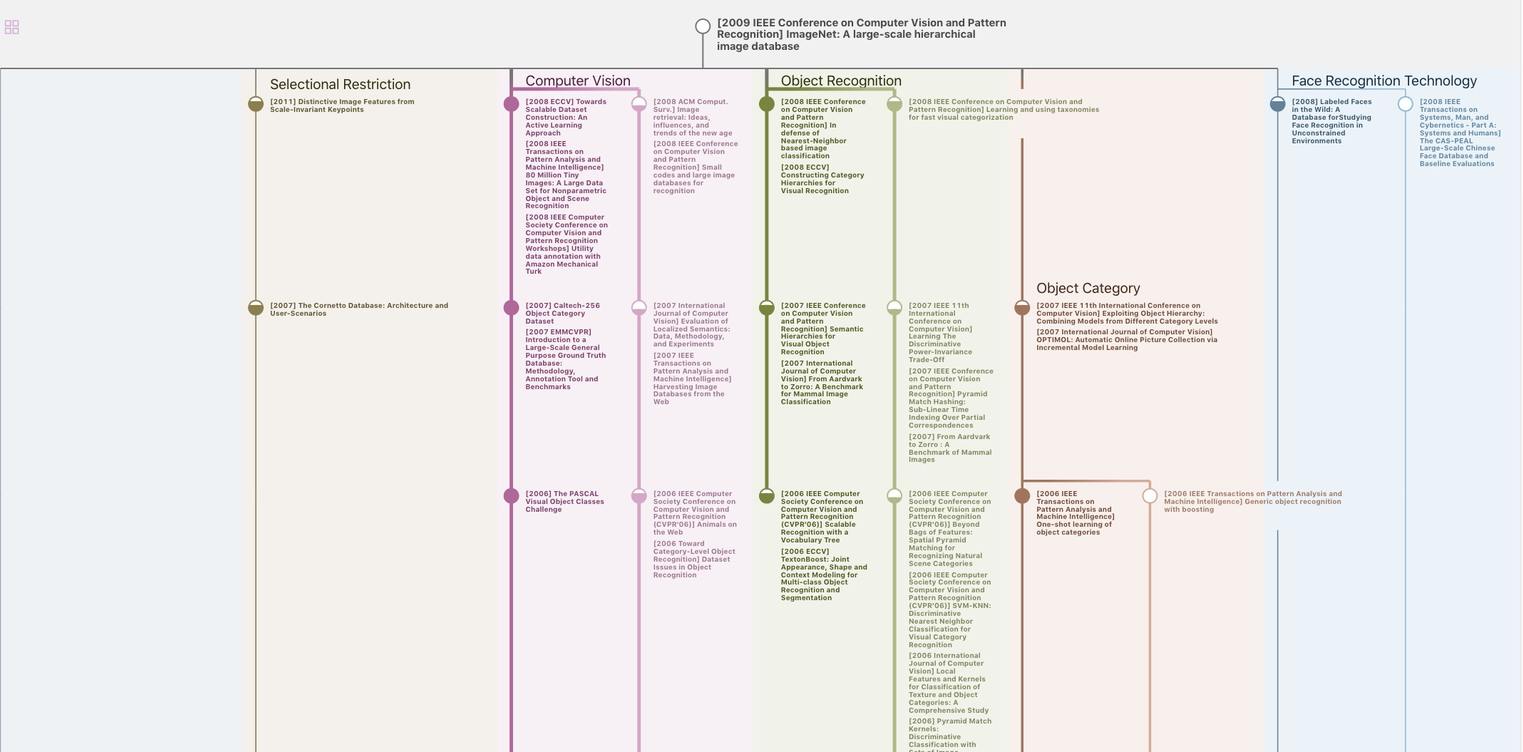
生成溯源树,研究论文发展脉络
Chat Paper
正在生成论文摘要