Deepamo: A Multi-Slice, Multi-View Anthropomorphic Model Observer For Visual Detection Tasks Performed On Volume Images
JOURNAL OF MEDICAL IMAGING(2021)
摘要
Purpose: We propose a deep learning-based anthropomorphic model observer (DeepAMO) for image quality evaluation of multi-orientation, multi-slice image sets with respect to a clinically realistic 3D defect detection task.Approach: The DeepAMO is developed based on a hypothetical model of the decision process of a human reader performing a detection task using a 3D volume. The DeepAMO is comprised of three sequential stages: defect segmentation, defect confirmation (DC), and rating value inference. The input to the DeepAMO is a composite image, typical of that used to view 3D volumes in clinical practice. The output is a rating value designed to reproduce a human observer's defect detection performance. In stages 2 and 3, we propose: (1) a projection-based DC block that confirms defect presence in two 2D orthogonal orientations and (2) a calibration method that "learns" the mapping from the features of stage 2 to the distribution of observer ratings from the human observer rating data (thus modeling inter- or intraobserver variability) using a mixture density network. We implemented and evaluated the DeepAMO in the context of Tc-99m-DMSA SPECT imaging. A human observer study was conducted, with two medical imaging physics graduate students serving as observers. A 5 x 2-fold cross-validation experiment was conducted to test the statistical equivalence in defect detection performance between the DeepAMO and the human observer. We also compared the performance of the DeepAMO to an unoptimized implementation of a scanning linear discriminant observer (SLDO).Results: The results show that the DeepAMO's and human observer's performances on unseen images were statistically equivalent with a margin of difference (Delta AUC) of 0.0426 at p < 0.05, using 288 training images. A limited implementation of an SLDO had a substantially higher AUC (0.99) compared to the DeepAMO and human observer.Conclusion: The results show that the DeepAMO has the potential to reproduce the absolute performance, and not just the relative ranking of human observers on a clinically realistic defect detection task, and that building conceptual components of the human reading process into deep learning-based models can allow training of these models in settings where limited training images are available. (C) 2021 Society of Photo-Optical Instrumentation Engineers (SPIE).
更多查看译文
关键词
model observer, deep learning, task-based image quality assessment
AI 理解论文
溯源树
样例
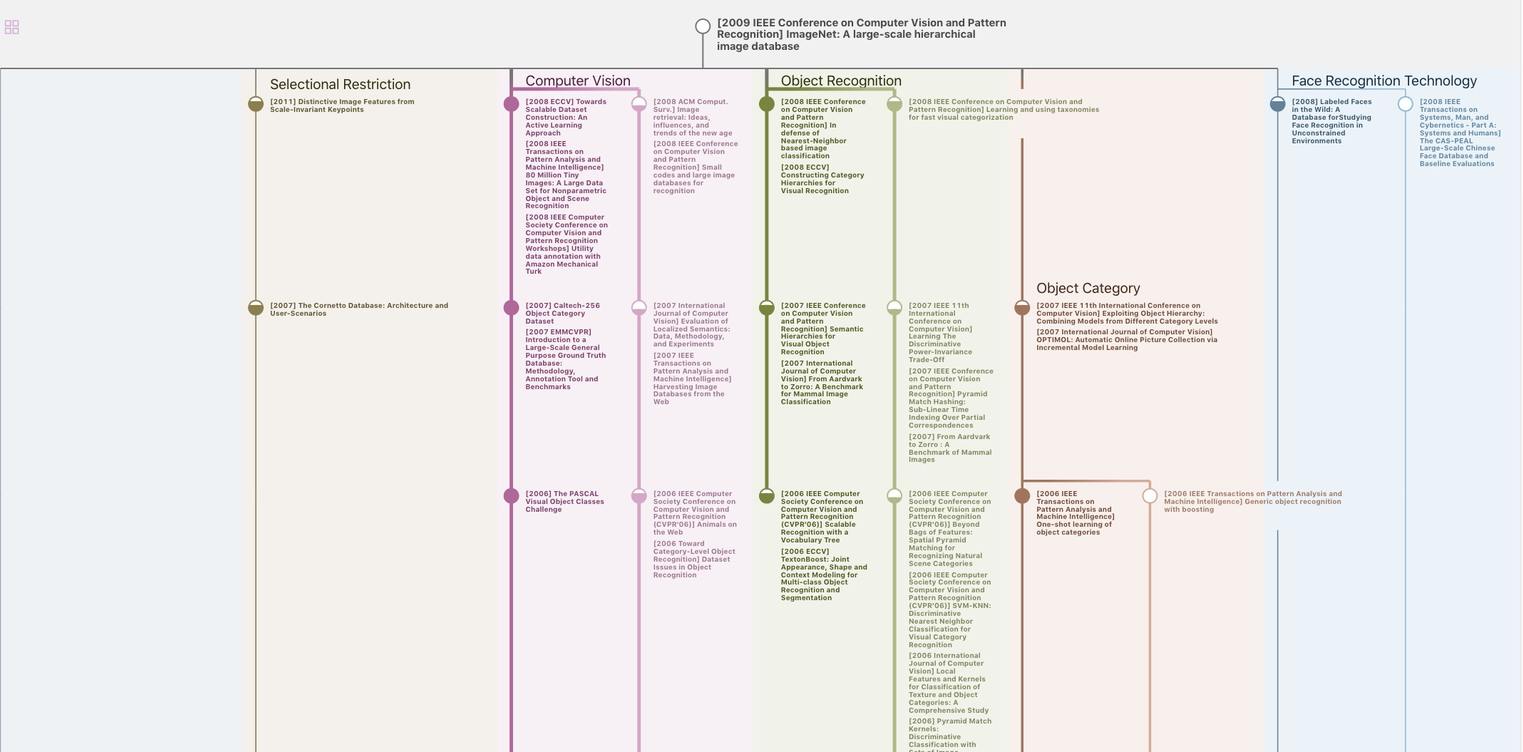
生成溯源树,研究论文发展脉络
Chat Paper
正在生成论文摘要