ObjectAug: Object-level Data Augmentation for Semantic Image Segmentation
2021 INTERNATIONAL JOINT CONFERENCE ON NEURAL NETWORKS (IJCNN)(2021)
Abstract
Effective training of deep neural networks (DNNs) usually requires labeling a large dataset, which is time and labor intensive. Recently, various data augmentation strategies like regional dropout and mix strategies have been proposed, which are effective as the augmented dataset can guide the model to attend on less discriminative parts. However, these strategies operate only at the image level, where the objects and the background are coupled. Thus, the boundaries are not well augmented due to the fixed semantic scenario. In this paper, we propose ObjectAug to perform object-level augmentation for semantic image segmentation. Our method first decouples the image into individual objects and the background using semantic labels. Second, each object is augmented individually with commonly used augmentation methods (e.g., scaling, shifting, and rotation). Third, the pixel artifacts brought by object augmentation are further restored using image inpainting. Finally, the augmented objects and background are assembled as an augmented image. In this way, the boundaries can be fully explored in the various semantic scenarios. In addition, ObjectAug can support category-aware augmentation that gives various possibilities to objects in each category, and can be easily combined with existing image-level augmentation methods to further boost the performance. Comprehensive experiments are conducted on both natural image and medical image datasets. Experiment results demonstrate that our ObjectAug can effectively improve segmentation performance.
MoreTranslated text
Key words
Object-level, Data Augmentation, Image Segmentation
AI Read Science
Must-Reading Tree
Example
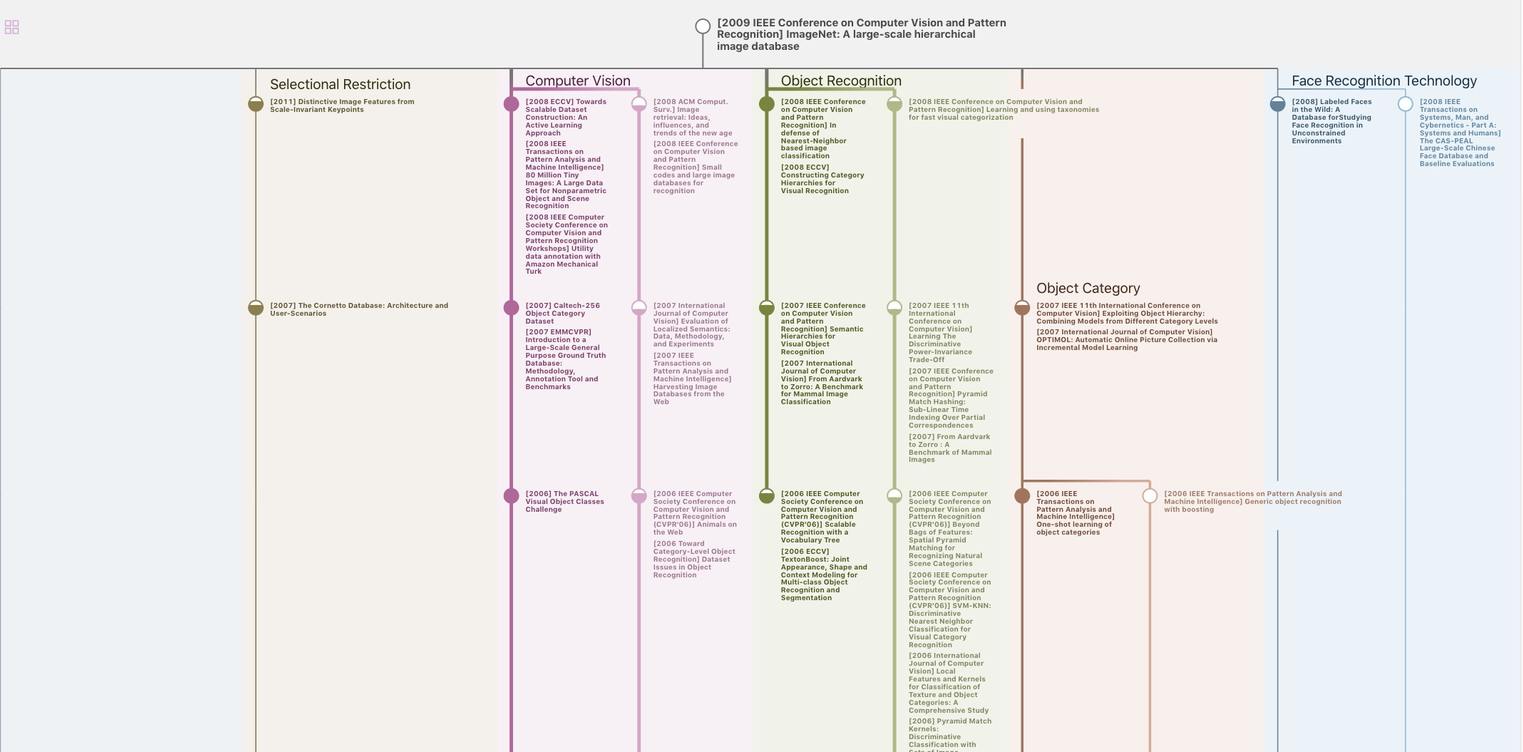
Generate MRT to find the research sequence of this paper
Chat Paper
Summary is being generated by the instructions you defined