Facilitating Knowledge Sharing from Domain Experts to Data Scientists for Building NLP Models
IUI(2021)
Abstract
ABSTRACT Data scientists face a steep learning curve in understanding a new domain for which they want to build machine learning (ML) models. While input from domain experts could offer valuable help, such input is often limited, expensive, and generally not in a form readily consumable by a model development pipeline. In this paper, we propose Ziva, a framework to guide domain experts in sharing essential domain knowledge to data scientists for building NLP models. With Ziva, experts are able to distill and share their domain knowledge using domain concept extractors and five types of label justification over a representative data sample. The design of Ziva is informed by preliminary interviews with data scientists, in order to understand current practices of domain knowledge acquisition process for ML development projects. To assess our design, we run a mix-method case-study to evaluate how Ziva can facilitate interaction between domain experts and data scientists. Our results highlight that (1) domain experts are able to use Ziva to provide rich domain knowledge, while maintaining low mental load and stress levels; and (2) data scientists find Ziva’s output helpful for learning essential information about the domain, offering scalability of information, and lowering the burden on domain experts to share knowledge. We conclude this work by experimenting with building NLP models using the Ziva output for our case study.
MoreTranslated text
Key words
Human-in-the-loop machine learning, CSCW, Multi-disciplinary collaboration
AI Read Science
Must-Reading Tree
Example
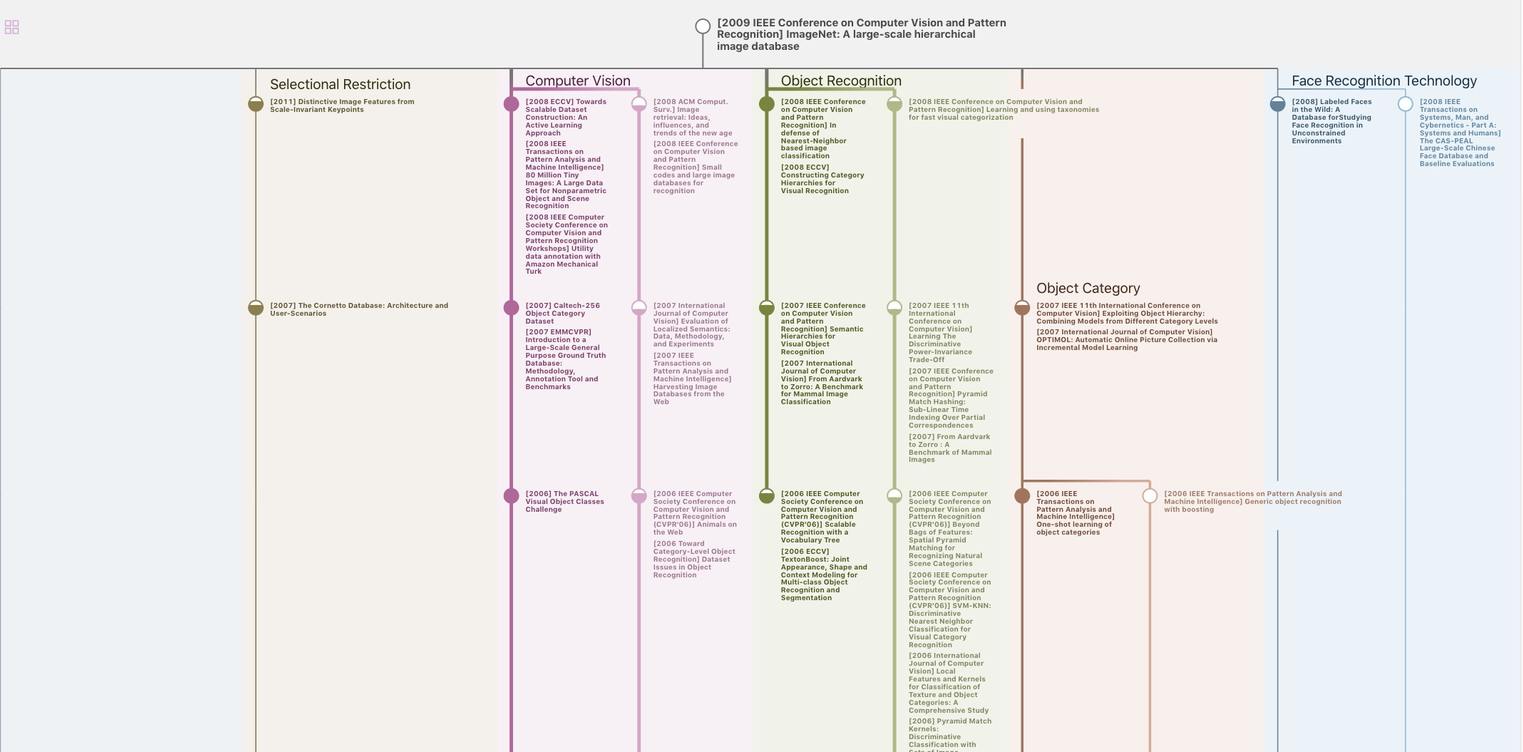
Generate MRT to find the research sequence of this paper
Chat Paper
Summary is being generated by the instructions you defined