A Phenotypic Risk Score For Predicting Mortality In Sickle Cell Disease
BRITISH JOURNAL OF HAEMATOLOGY(2021)
摘要
Risk assessment for patients with sickle cell disease (SCD) remains challenging as it depends on an individual physician's experience and ability to integrate a variety of test results. We aimed to provide a new risk score that combines clinical, laboratory, and imaging data. In a prospective cohort of 600 adult patients with SCD, we assessed the relationship of 70 baseline covariates to all-cause mortality. Random survival forest and regularised Cox regression machine learning (ML) methods were used to select top predictors. Multivariable models and a risk score were developed and internally validated. Over a median follow-up of 4 center dot 3 years, 131 deaths were recorded. Multivariable models were developed using nine independent predictors of mortality: tricuspid regurgitant velocity, estimated right atrial pressure, mitral E velocity, left ventricular septal thickness, body mass index, blood urea nitrogen, alkaline phosphatase, heart rate and age. Our prognostic risk score had superior performance with a bias-corrected C-statistic of 0 center dot 763. Our model stratified patients into four groups with significantly different 4-year mortality rates (3%, 11%, 35% and 75% respectively). Using readily available variables from patients with SCD, we applied ML techniques to develop and validate a mortality risk scoring method that reflects the summation of cardiopulmonary, renal and liver end-organ damage.Trial Registration: ClinicalTrials.gov Identifier: NCT#00011648.
更多查看译文
关键词
sickle cell anaemia, risk assessment, machine learning
AI 理解论文
溯源树
样例
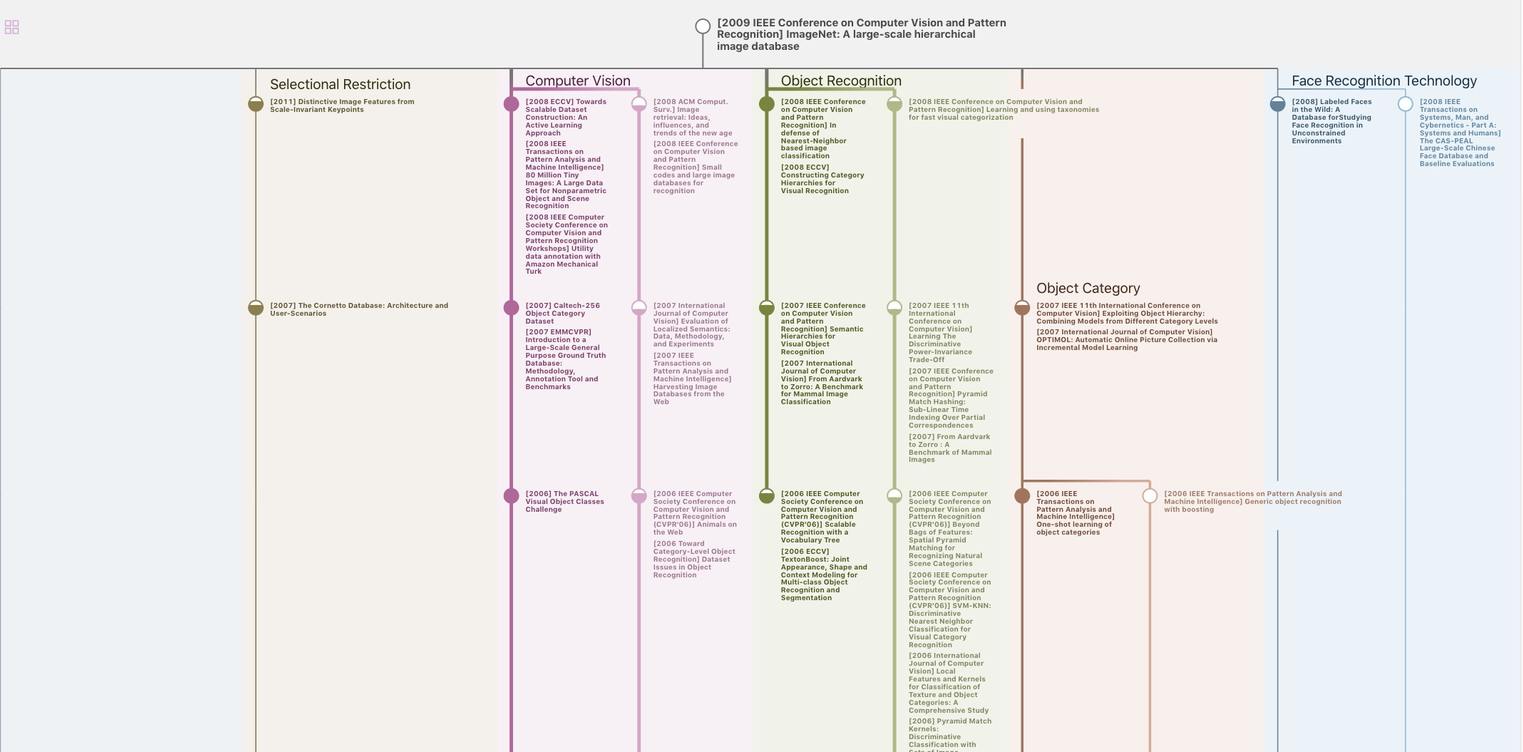
生成溯源树,研究论文发展脉络
Chat Paper
正在生成论文摘要