MutagenPred-GCNNs: A Graph Convolutional Neural Network-Based Classification Model for Mutagenicity Prediction with Data-Driven Molecular Fingerprints
INTERDISCIPLINARY SCIENCES-COMPUTATIONAL LIFE SCIENCES(2021)
摘要
An important task in the early stage of drug discovery is the identification of mutagenic compounds. Mutagenicity prediction models that can interpret relationships between toxicological endpoints and compound structures are especially favorable. In this research, we used an advanced graph convolutional neural network (GCNN) architecture to identify the molecular representation and develop predictive models based on these representations. The predictive model based on features extracted by GCNNs can not only predict the mutagenicity of compounds but also identify the structure alerts in compounds. In fivefold cross-validation and external validation, the highest area under the curve was 0.8782 and 0.8382, respectively; the highest accuracy ( Q ) was 80.98% and 76.63%, respectively; the highest sensitivity was 83.27% and 78.92%, respectively; and the highest specificity was 78.83% and 76.32%, respectively. Additionally, our model also identified some toxicophores, such as aromatic nitro, three-membered heterocycles, quinones, and nitrogen and sulfur mustard. These results indicate that GCNNs could learn the features of mutagens effectively. In summary, we developed a mutagenicity classification model with high predictive performance and interpretability based on a data-driven molecular representation trained through GCNNs.
更多查看译文
关键词
Graph convolutional networks, Mutagenicity prediction, Deep learning
AI 理解论文
溯源树
样例
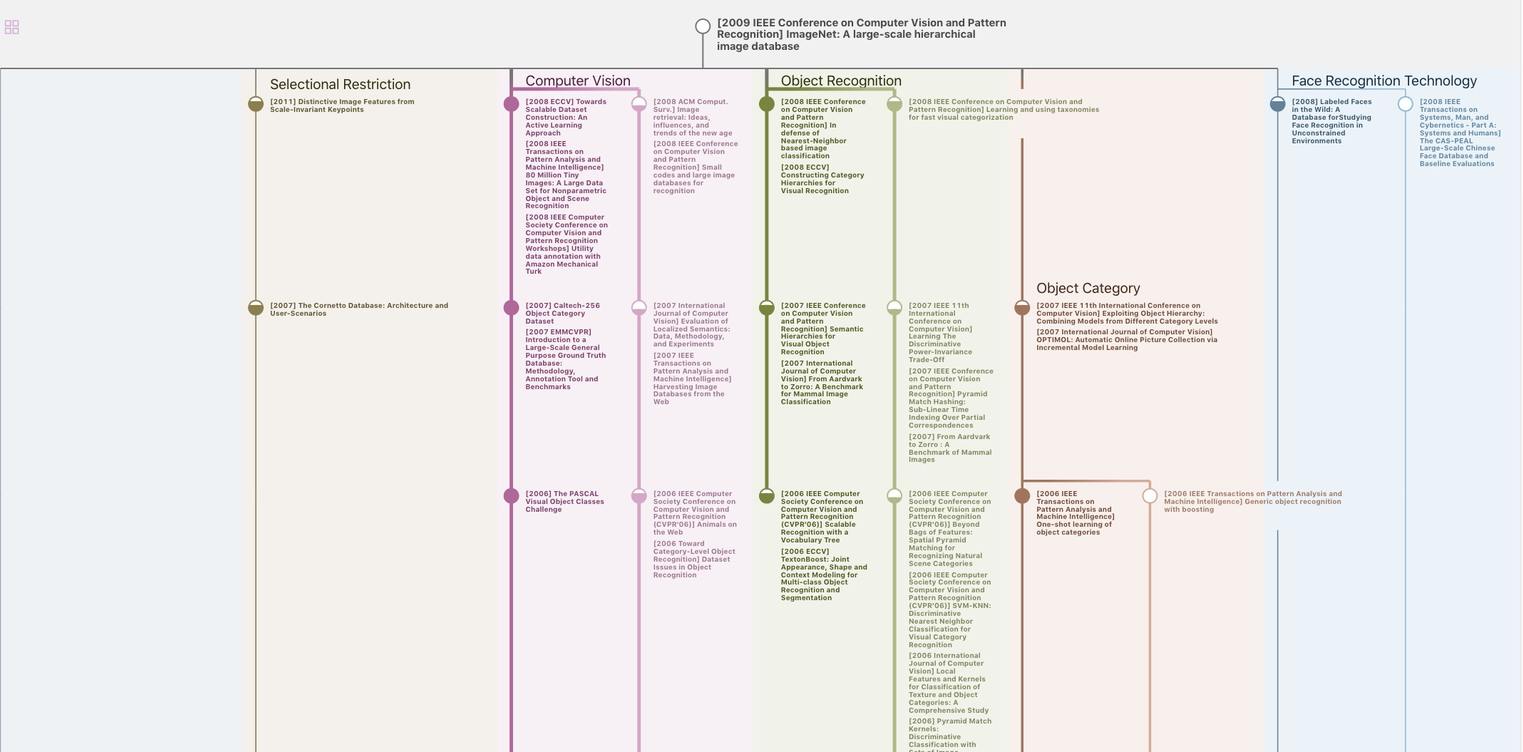
生成溯源树,研究论文发展脉络
Chat Paper
正在生成论文摘要