Diagnosing Alzheimer's Disease Based on Multiclass MRI Scans using Transfer Learning Techniques
CURRENT MEDICAL IMAGING(2021)
摘要
Aims: To prevent Alzheimer's disease (AD) from progressing to dementia, early predic-tion and classification of AD are important and they play a crucial role in medical image analysis. Background: In this study, we employed a transfer learning technique to classify magnetic reso-nance (MR) images using a pre-trained convolutional neural network (CNN). Objective: To address the early diagnosis of AD, we employed a computer-assisted technique, spe-cifically the deep learning (DL) model, to detect AD. Methods: In particular, we classified Alzheimer's disease (AD), mild cognitive impairment (MCI), and normal control (NC) subjects using whole slide two-dimensional (2D) images. To illustrate this approach, we made use of state-of-the-art CNN base models, i.e., the residual networks Res -Net-101, ResNet-50, and ResNet-18, and compared their effectiveness in identifying AD. To evalu-ate this approach, an AD Neuroimaging Initiative (ADNI) dataset was utilized. We also showed uniqueness by using MR images selected only from the central slice containing left and right hippo-campus regions to evaluate the models. Results: All three models used randomly split data in the ratio of 70:30 for training and testing. Among the three, ResNet-101 showed 98.37% accuracy, better than the other two ResNet models, and performed well in multiclass classification. The promising results emphasize the benefit of us-ing transfer learning, specifically when the dataset is low. Conclusion: From this study, we know that transfer learning helps to overcome DL problems main-ly when the data available is insufficient to train a model from scratch. This approach is highly ad-vantageous in medical image analysis to diagnose diseases like AD.
更多查看译文
关键词
Alzheimer's disease, CNN, deep learning, MR images, residual networks, transfer learning
AI 理解论文
溯源树
样例
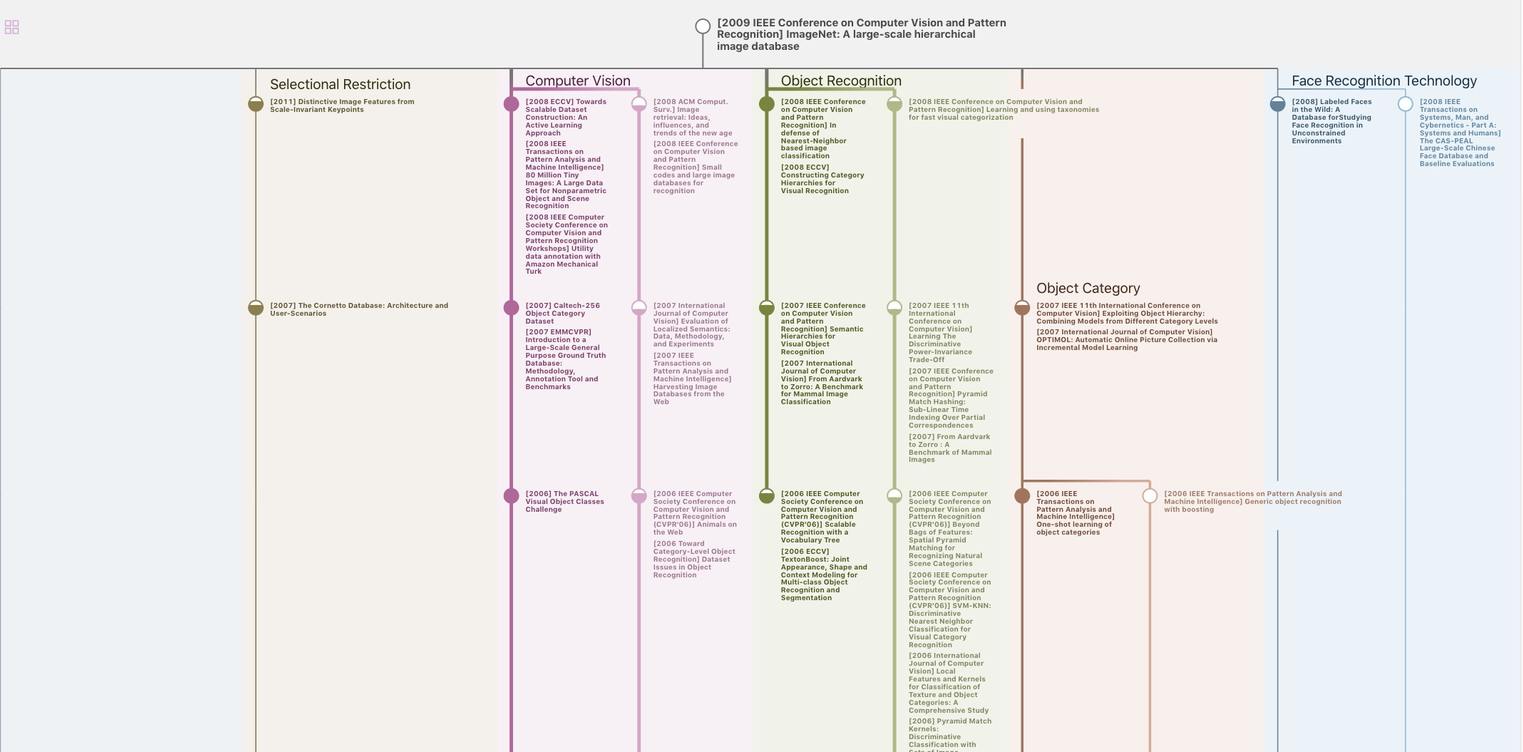
生成溯源树,研究论文发展脉络
Chat Paper
正在生成论文摘要