Accelerating Dynamic Time Warping-Based Keyword Search Using Recurrent Neural Networks
2020 28TH SIGNAL PROCESSING AND COMMUNICATIONS APPLICATIONS CONFERENCE (SIU)(2020)
摘要
Keyword Search (KWS) is the task of retrieving terms of interest within speech. The main approach to KWS, using continuous speech recognition systems fails to perform well on limited resource languages. Dynamic time warping (DTW)-based similarity search techniques provide a useful alternative in these circumstances. However, the main drawback of DTW-based approaches is that their search time is relatively longer. Recurrent neural network-based representation learning techniques have been receiving increasing interest in the literature and it is possible to conduct high speed KWS with this method. In this paper, we investigate the application of representation learning-based systems as a pre-filtering operation to DTW-based search in order to increase the speed. Experiments on Turkish speech data of telephone conversations provided by the IARPA Babel program show that the pre-filtering can increase the speed of search by 35% with a negligible drop in the DTW-based search performance.
更多查看译文
关键词
keyword search, dynamic time warping, pre-filtering, recurrent autoencoders, recurrent neural networks
AI 理解论文
溯源树
样例
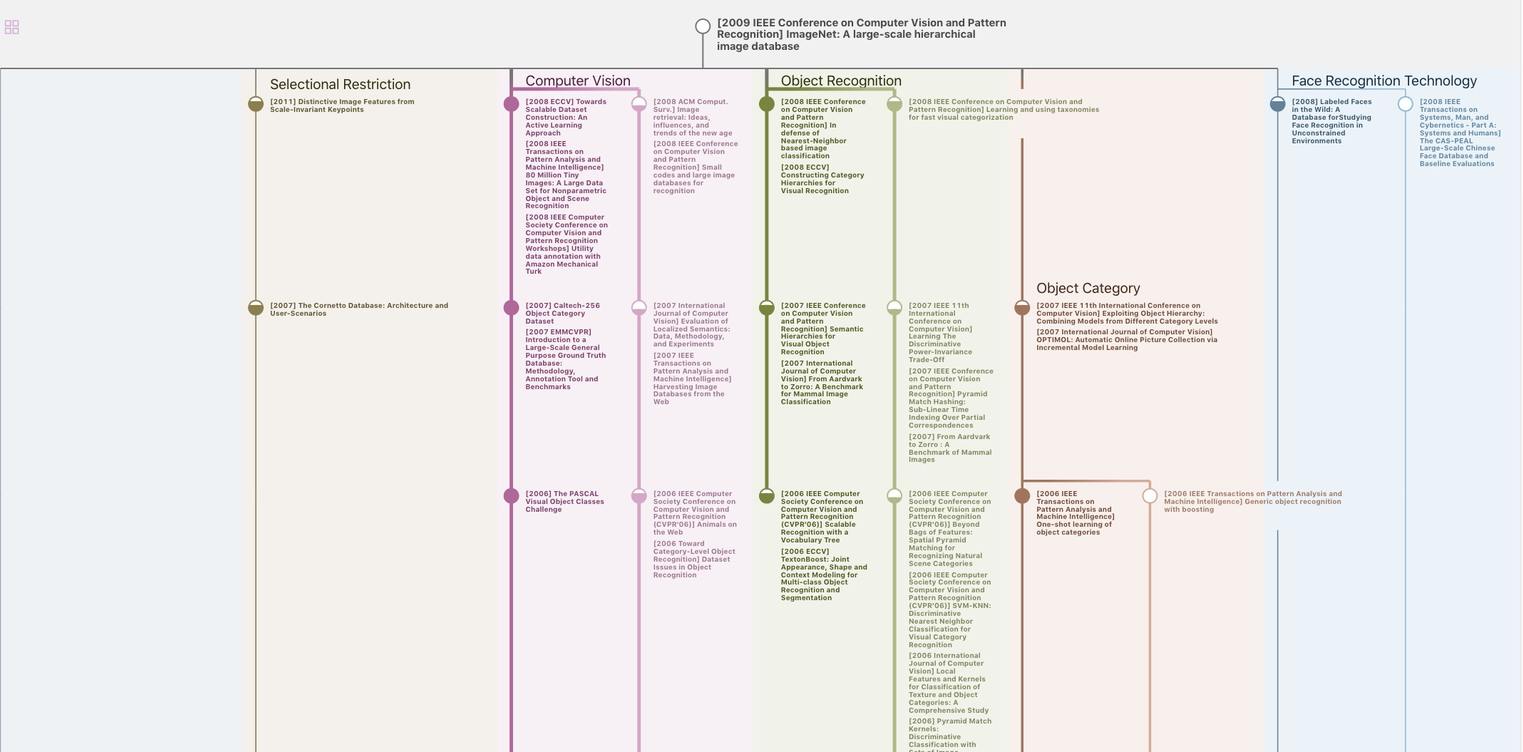
生成溯源树,研究论文发展脉络
Chat Paper
正在生成论文摘要