Prediction of Total Drug Clearance in Humans Using Animal Data: Proposal of a Multimodal Learning Method Based on Deep Learning
Journal of Pharmaceutical Sciences(2021)
Abstract
Research into pharmacokinetics plays an important role in the development process of new drugs. Accurately predicting human pharmacokinetic parameters from preclinical data can increase the success rate of clinical trials. Since clearance (CL) which indicates the capacity of the entire body to process a drug is one of the most important parameters, many methods have been developed. However, there are still rooms to be improved for practical use in drug discovery research; “improving CL prediction accuracy” and “understanding the chemical structure of compounds in terms of pharmacokinetics”. To improve those, this research proposes a multimodal learning method based on deep learning that takes not only the chemical structure of a drug but also rat CL as inputs. Good results were obtained compared with the conventional animal scale-up method; the geometric mean fold error was 2.68 and the proportion of compounds with prediction errors of 2-fold or less was 48.5%. Furthermore, it was found to be possible to infer the partial structure useful for CL prediction by a structure contributing factor inference method. The validity of these results of structural interpretation of metabolic stability was confirmed by chemists.
MoreTranslated text
Key words
total drug clearance,multimodal learning method,animal data,deep learning
AI Read Science
Must-Reading Tree
Example
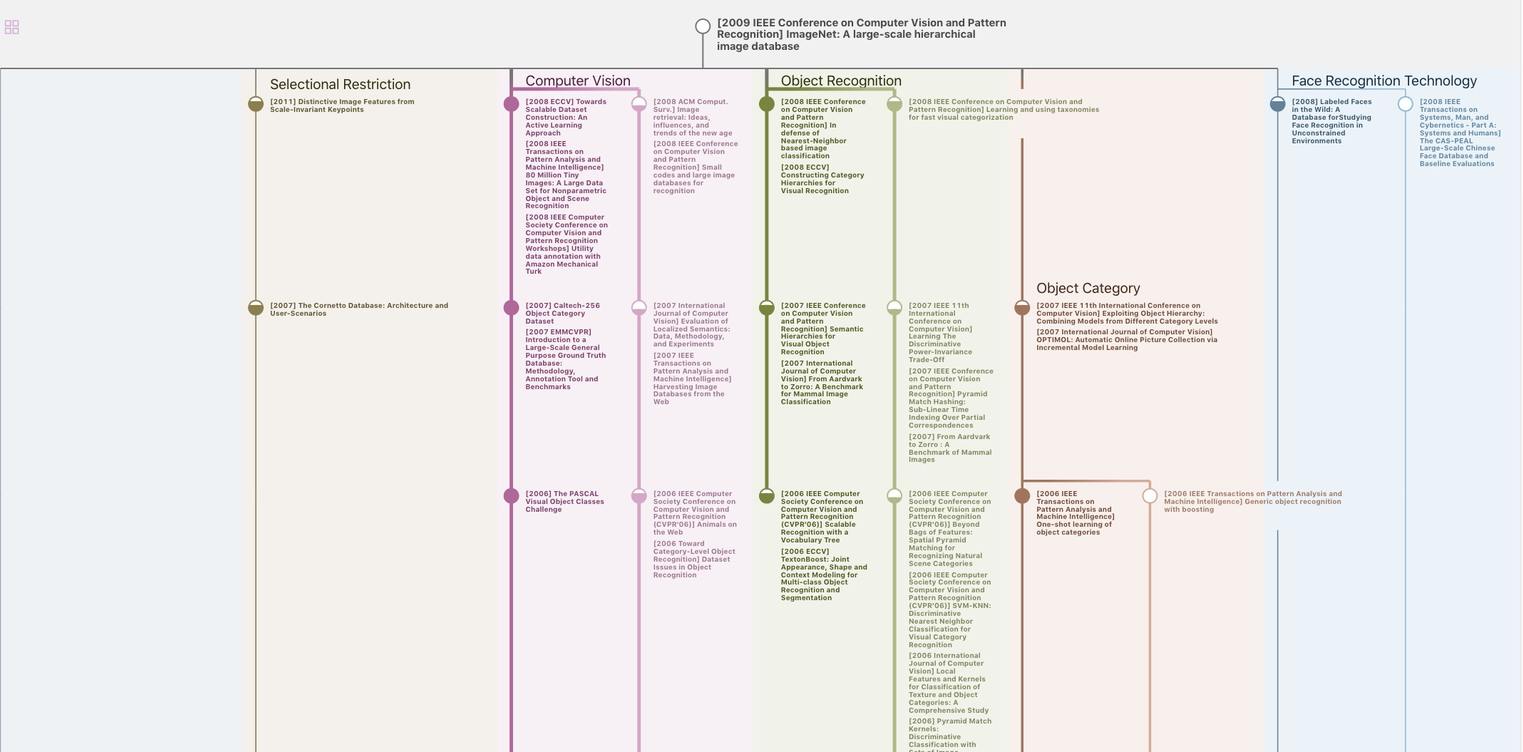
Generate MRT to find the research sequence of this paper
Chat Paper
Summary is being generated by the instructions you defined