A Novel Knowledge Base Question Answering Model Based on Knowledge Representation and Recurrent Convolutional Neural Network
2020 International Conference on Service Science (ICSS)(2020)
Abstract
The goal of the question-answering (QA) system is to understand the questions from users and return their accurate answers. In the medical field, the question-answering system amis to understand patients' questions and return the correct answers. The existed knowledge base question-answering (KB-QA) systems mainly rely on hand-crafted features and ignore structure information of knowledge base which accordingly lead to the answers with low accuracy. In this paper, a novel KB-QA model is put forward based on knowledge representation and recurrent convolutional neural network. This model has three parts, candidate answers generation, entity relationships extraction and knowledge representation learning based on knowledge base. In addition, an algorithm is also developed to compute the scores of linking candidate answers and knowledge base. Experimental results show that the presented model achieves better performance compared with the baseline systems.
MoreTranslated text
Key words
knowledge base question-answering model,recurrent convolutional neural network,knowledge representation
AI Read Science
Must-Reading Tree
Example
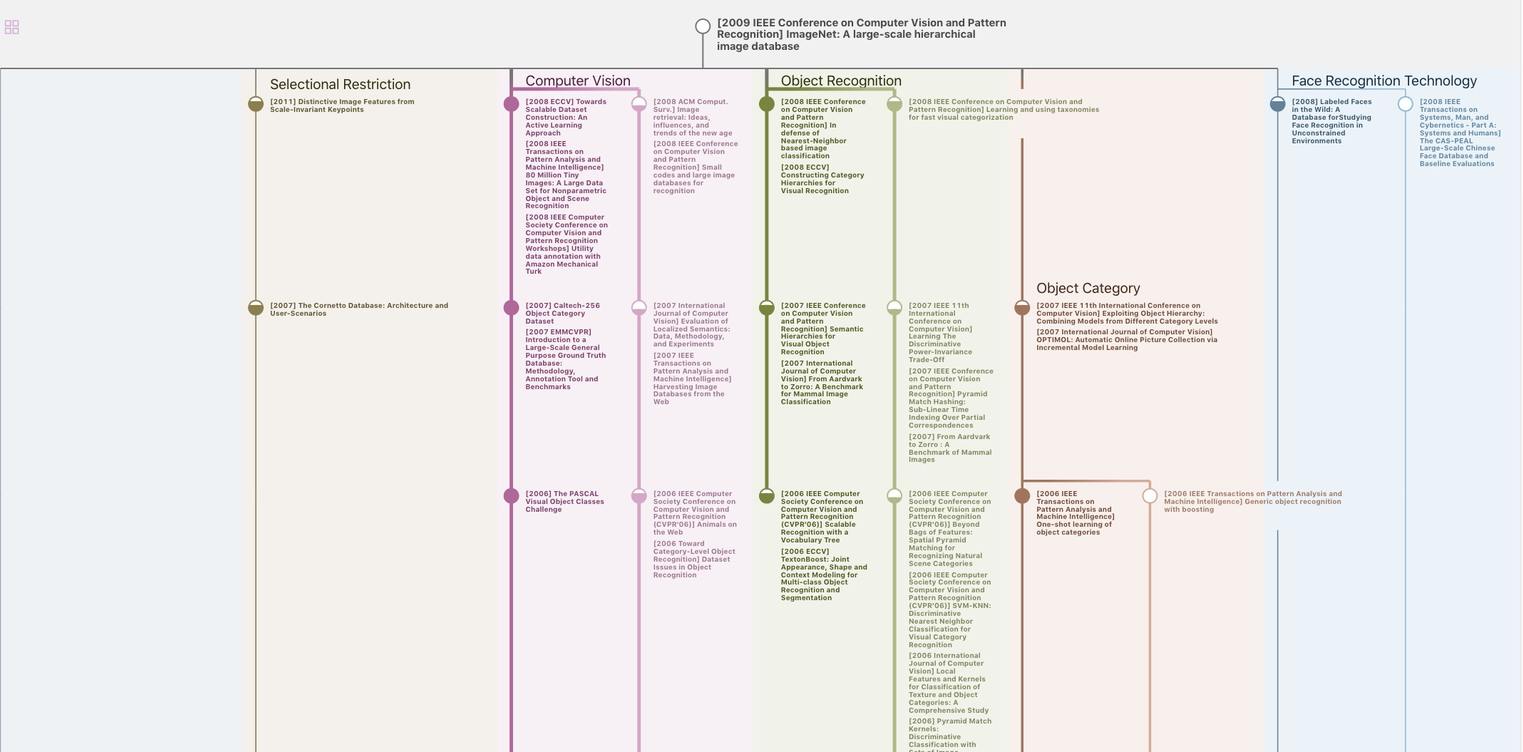
Generate MRT to find the research sequence of this paper
Chat Paper
Summary is being generated by the instructions you defined