Revisiting Contrastive Learning for Few-Shot Classification
arxiv(2021)
摘要
Instance discrimination based contrastive learning has emerged as a leading approach for self-supervised learning of visual representations. Yet, its generalization to novel tasks remains elusive when compared to representations learned with supervision, especially in the few-shot setting. We demonstrate how one can incorporate supervision in the instance discrimination based contrastive self-supervised learning framework to learn representations that generalize better to novel tasks. We call our approach CIDS (Contrastive Instance Discrimination with Supervision). CIDS performs favorably compared to existing algorithms on popular few-shot benchmarks like Mini-ImageNet or Tiered-ImageNet. We also propose a novel model selection algorithm that can be used in conjunction with a universal embedding trained using CIDS to outperform state-of-the-art algorithms on the challenging Meta-Dataset benchmark.
更多查看译文
关键词
contrastive learning,classification,few-shot
AI 理解论文
溯源树
样例
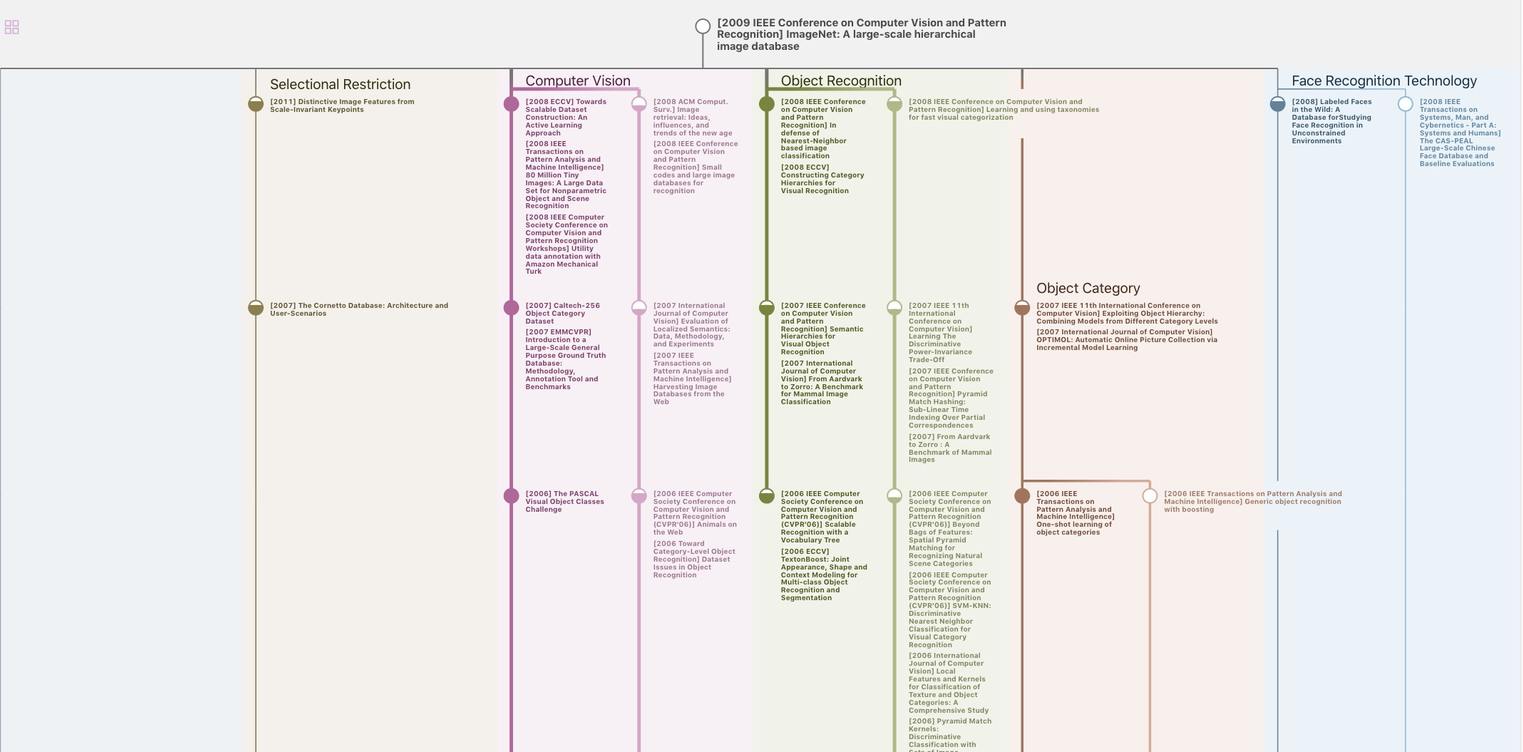
生成溯源树,研究论文发展脉络
Chat Paper
正在生成论文摘要