Domain-Dependent Speaker Diarization for the Third DIHARD Challenge
arxiv(2021)
摘要
This report presents the system developed by the ABSP Laboratory team for the third DIHARD speech diarization challenge. Our main contribution in this work is to develop a simple and efficient solution for acoustic domain dependent speech diarization. We explore speaker embeddings for \emph{acoustic domain identification} (ADI) task. Our study reveals that i-vector based method achieves considerably better performance than x-vector based approach in the third DIHARD challenge dataset. Next, we integrate the ADI module with the diarization framework. The performance substantially improved over that of the baseline when we optimized the thresholds for agglomerative hierarchical clustering and the parameters for dimensionality reduction during scoring for individual acoustic domains. We achieved a relative improvement of $9.63\%$ and $10.64\%$ in DER for core and full conditions, respectively, for Track 1 of the DIHARD III evaluation set.
更多查看译文
关键词
challenge,domain-dependent
AI 理解论文
溯源树
样例
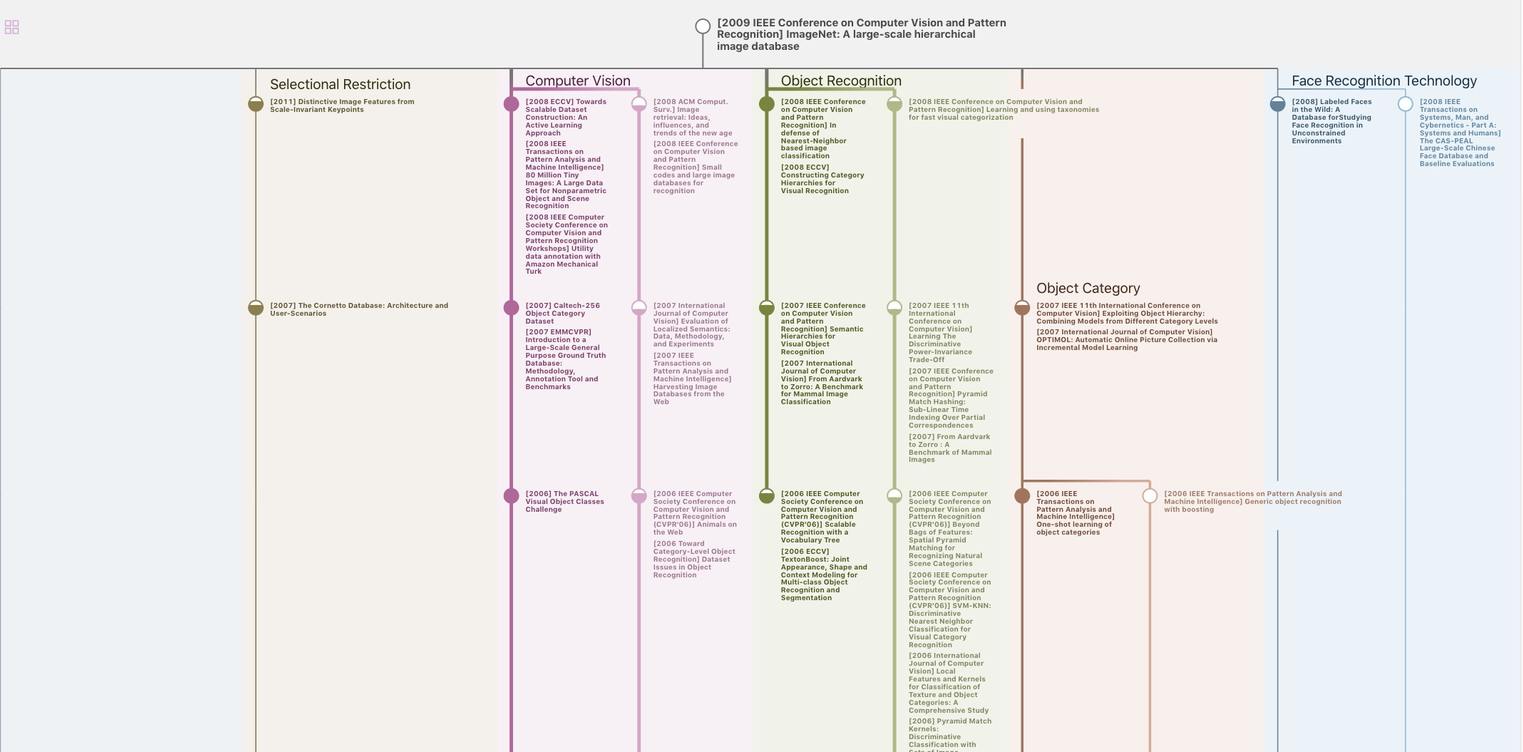
生成溯源树,研究论文发展脉络
Chat Paper
正在生成论文摘要