Investigating the accuracy of cross-learning time series forecasting methods
International Journal of Forecasting(2021)
摘要
The M4 competition identified innovative forecasting methods, advancing the theory and practice of forecasting. One of the most promising innovations of M4 was the utilization of cross-learning approaches that allow models to learn from multiple series how to accurately predict individual ones. In this paper, we investigate the potential of cross-learning by developing various neural network models that adopt such an approach, and we compare their accuracy to that of traditional models that are trained in a series-by-series fashion. Our empirical evaluation, which is based on the M4 monthly data, confirms that cross-learning is a promising alternative to traditional forecasting, at least when appropriate strategies for extracting information from large, diverse time series data sets are considered. Ways of combining traditional with cross-learning methods are also examined in order to initiate further research in the field.
更多查看译文
关键词
Time series,Cross-learning,Features,Neural networks,M4 competition
AI 理解论文
溯源树
样例
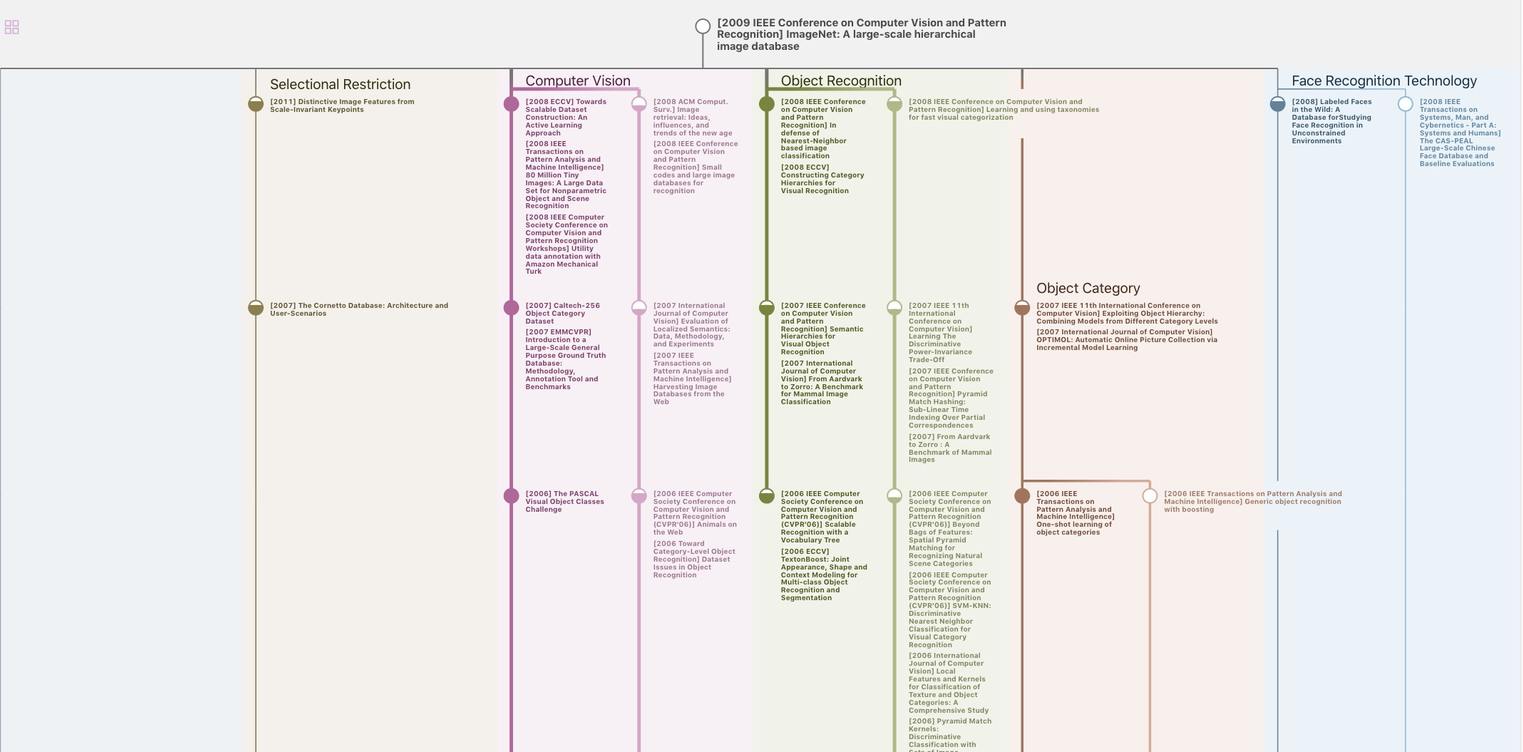
生成溯源树,研究论文发展脉络
Chat Paper
正在生成论文摘要