Geometric attentional dynamic graph convolutional neural networks for point cloud analysis.
Neurocomputing(2021)
摘要
This paper proposes geometric attentional dynamic graph convolutional neural networks for point cloud analysis. The core operation is a geometric attentional edge convolution module which extends classic CNN to extract both extrinsic and intrinsic properties of point clouds for a rich representation learning of point features. The relations in geometric space regarding the extrinsic geometric topological prior are modeled as geometric attention and incorporated in the EdgeConv of DGCNN which captures the intrinsic feature likelihood of point clouds. Therefore, the proposed geometric attentional edge convolution is able to learn point cloud representations from both intrinsic and extrinsic properties. To form a hierarchical architecture to capture rich information of point clouds from these two kinds of underlying properties, two graphs are dynamic constructed in the geometric and feature space respectively layer by layer. Extensive experiments on several benchmarks for various point cloud analysis tasks, including shape classification, share retrieval, normal estimation, shape part segmentation, have verified the effectiveness of the proposed method.
更多查看译文
关键词
Point cloud analysis,Dynamic graph neural networks,Geometric attention,3D deep learning
AI 理解论文
溯源树
样例
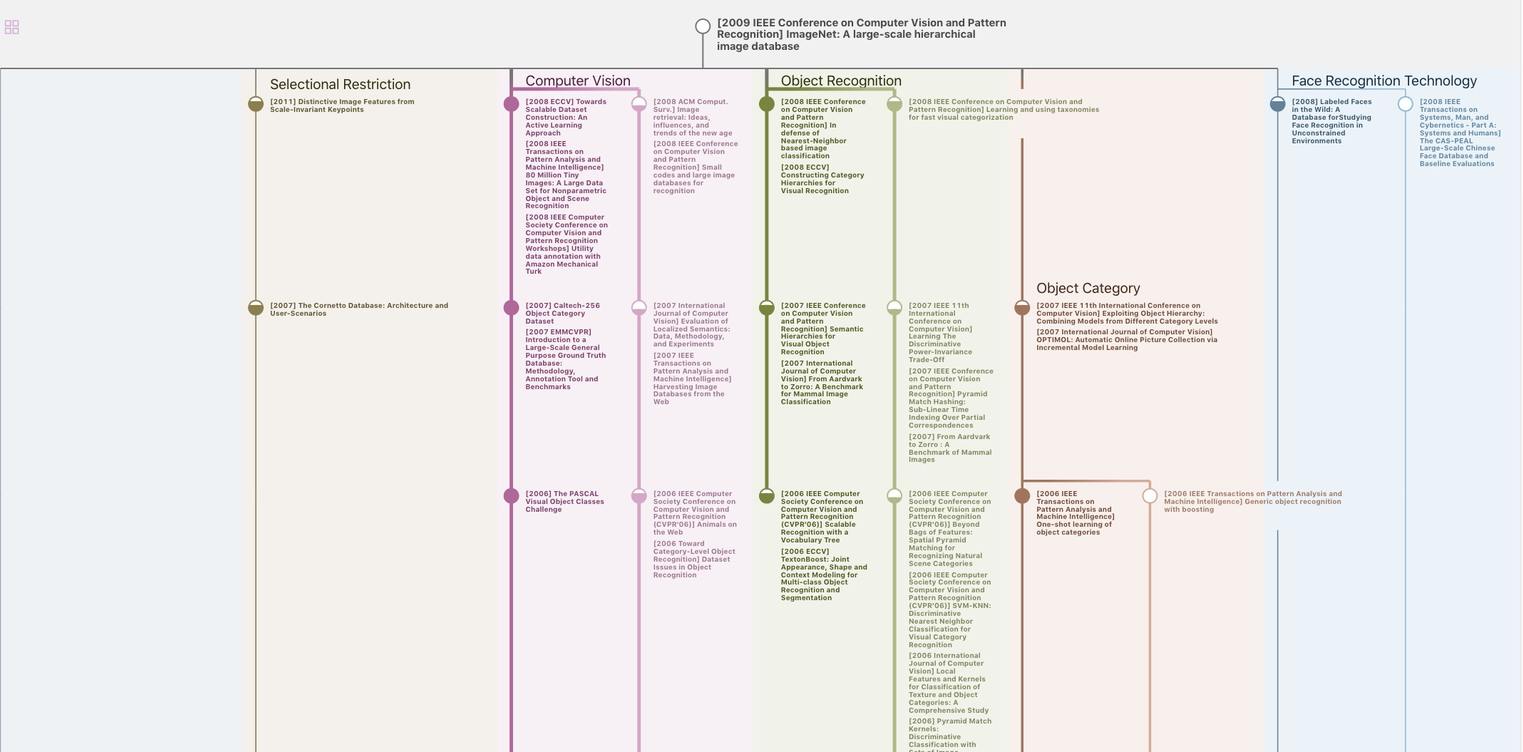
生成溯源树,研究论文发展脉络
Chat Paper
正在生成论文摘要