Dynamic Bayesian adjustment of anticipatory covariates in retrospective data: application to the effect of education on divorce risk.
JOURNAL OF APPLIED STATISTICS(2020)
摘要
We address a problem in inference from retrospective studies where the value of a variable is measured at the date of the survey but is used as covariate to events that have occurred long before the survey. This causes problem because the value of the current-date (anticipatory) covariate does not follow the temporal order of events. We propose a dynamic Bayesian approach for modelling jointly the anticipatory covariate and the event of interest, and allowing the effects of the anticipatory covariate to vary over time. The issues are illustrated with data on the effects of education attained by the survey-time on divorce risks among Swedish men. The overall results show that failure to adjust for the anticipatory nature of education leads to elevated relative risks of divorce across educational levels. The results are partially in accordance with previous findings based on analyses of the same data set. More importantly, our findings provide new insights in that the bias due to anticipatory covariates varies over marriage duration.
更多查看译文
关键词
Anticipatory covariates, Bayesian inference, current-date covariates, dynamic modelling, educational gradients in divorce-risks, observational studies, particle filter, retrospective surveys, Sweden
AI 理解论文
溯源树
样例
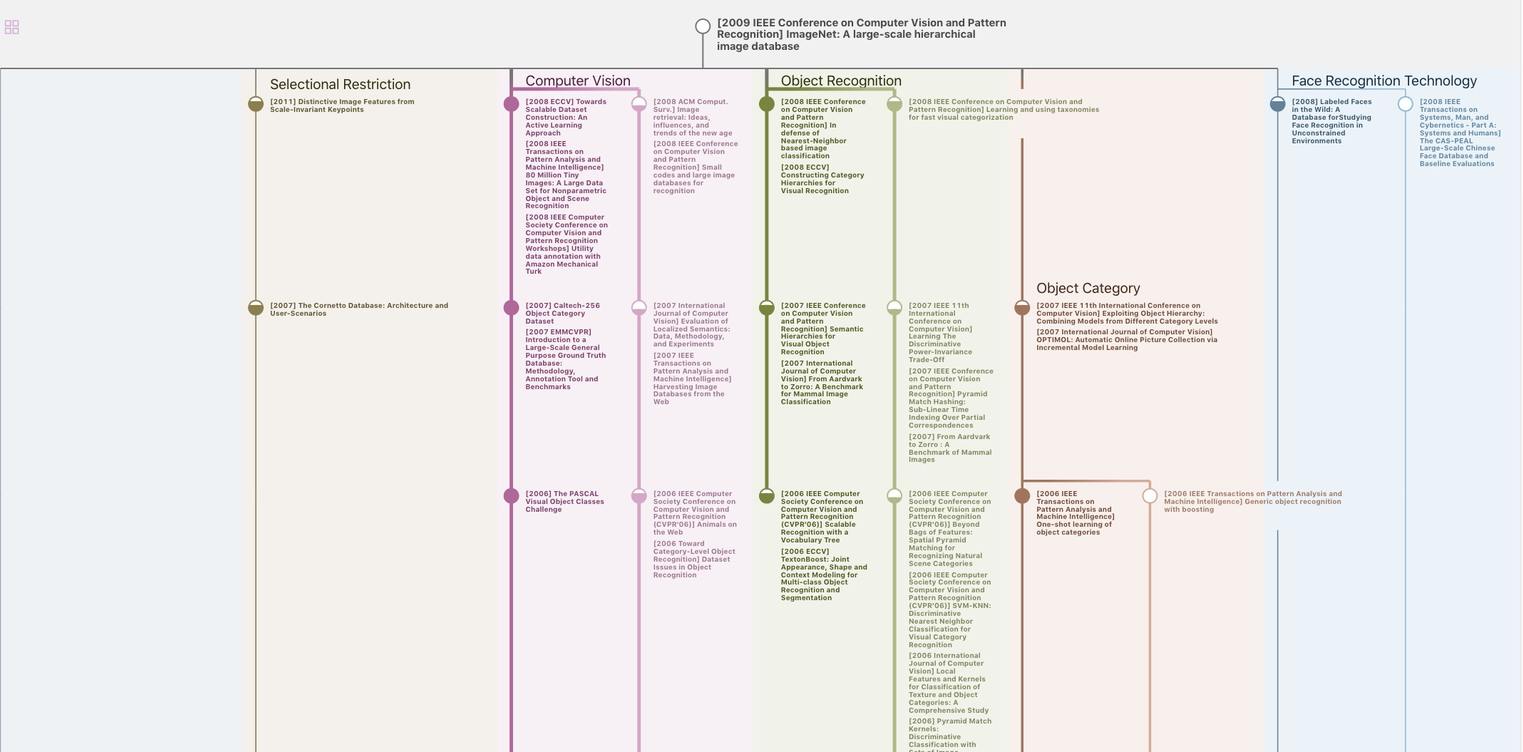
生成溯源树,研究论文发展脉络
Chat Paper
正在生成论文摘要