AGAMI: Scalable Visual Analytics over Multidimensional Data Streams.
2020 IEEE/ACM INTERNATIONAL CONFERENCE ON BIG DATA COMPUTING, APPLICATIONS AND TECHNOLOGIES (BDCAT 2020)(2020)
关键词
Streaming visualization,big data visualization,in-memory data sketches,online machine learning
AI 理解论文
溯源树
样例
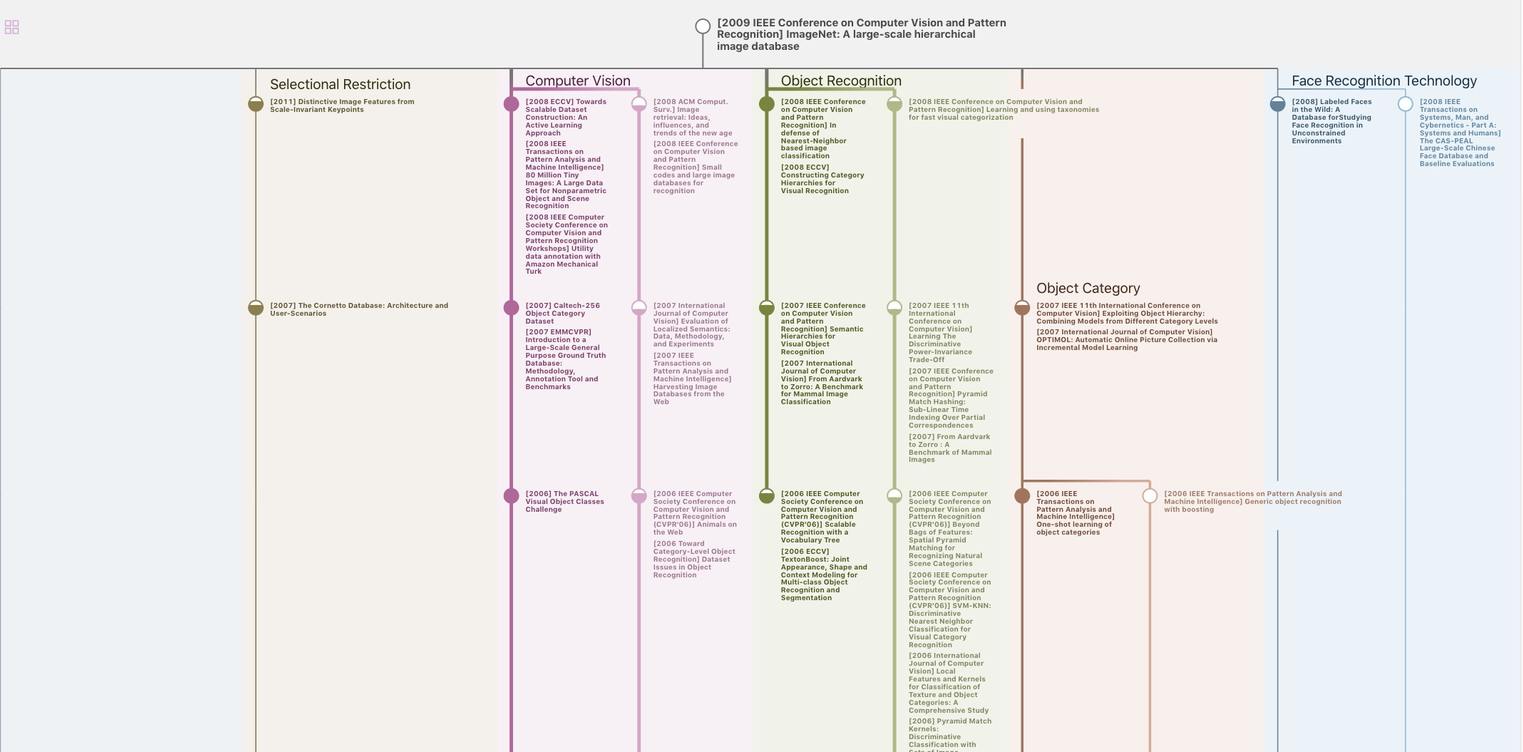
生成溯源树,研究论文发展脉络
Chat Paper
正在生成论文摘要