TangleCV: A Distributed Ledger Technique for Secure Message Sharing in Connected Vehicles
ACM Transactions on Cyber-Physical Systems(2021)
Abstract
AbstractConnected vehicles are set to define the future of transportation; however, this upcoming technology continues to be plagued with serious security risks. If these risks are not addressed in a timely fashion, then they could threaten the adoption and success of this promising technology. This article deals with a specific class of attacks in connected vehicles, namely tampering attacks caused due to compromise of on-board sensors. Current centralized solutions that employ trusted infrastructure to protect against adversarial manipulation of information cannot validate the correctness of the shared data and do not scale well. To overcome these issues, decentralized protection mechanisms by means of blockchain technology have emerged as a promising research direction. However, current permission-less, linear blockchain-based solutions have low transaction performance and high computational cost, thereby making it difficult to adopt them for security in connected vehicles. In this article, we present TangleCV, a directed acyclic graph–based distributed ledger technique for connected vehicles to address data tampering threats in connected vehicular networks. We describe new validation steps, tip selection strategies, and cumulative weight definition for TangleCV that not only meets the timing constraints of the connected vehicular networks but also secures the network against threats due to tampering attacks. We describe how the reputation of the network is established in TangleCV using trust factors calculated on the basis of ability, integrity, and benevolence of the nodes in the network. We present numerical results that demonstrate that the average value of the time to first approval decreases by more than 70% as the network evolves from a low load to a high load in the case of the nearest neighbor strategy. We observe that more than 60% of the nodes are approved in a low-load network and this number increases to 80% in a high-load network for the nearest neighbor strategy. The standard deviation of error measurements for nodes experiencing tampering attack is around 60% higher as compared to nodes that do not experience such an attack.
MoreTranslated text
Key words
Tangle, blockchain, IOTA, connected vehicles, security, directed acyclic graph
AI Read Science
Must-Reading Tree
Example
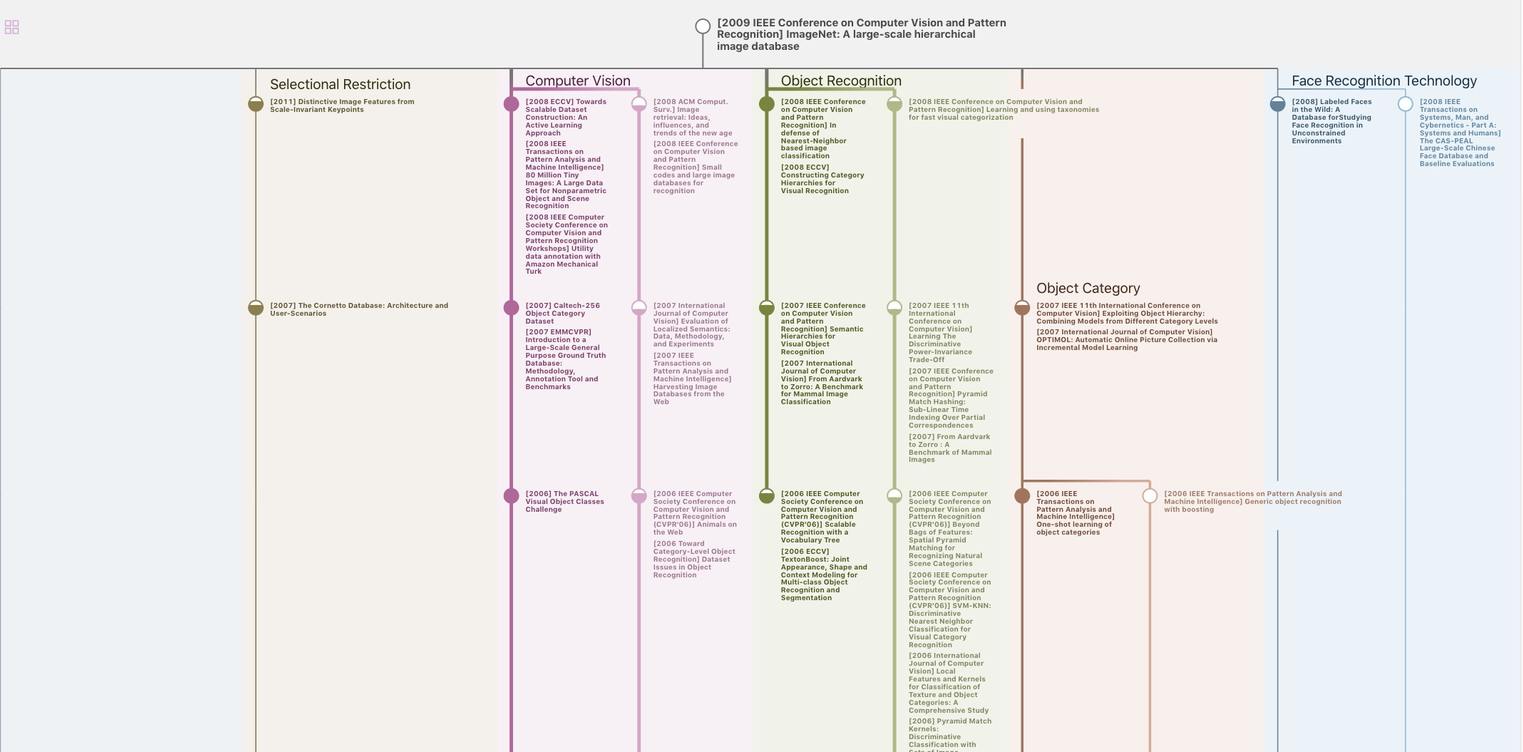
Generate MRT to find the research sequence of this paper
Chat Paper
Summary is being generated by the instructions you defined