Predicting Issue Types On Github
SCIENCE OF COMPUTER PROGRAMMING(2021)
摘要
Software maintenance and evolution involves critical activities for the success of software projects. To support such activities and keep code up-to-date and error-free, software communities make use of issue trackers, i.e., tools for signaling, handling, and addressing the issues occurring in software systems. However, in popular projects, tens or hundreds of issue reports are daily submitted. In this context, identifying the type of each submitted report (e.g., bug report, feature request, etc.) would facilitate the management and the prioritization of the issues to address. To support issue handling activities, in this paper, we propose TICKET TAGGER, a GitHub app analyzing the issue title and description through machine learning techniques to automatically recognize the types of reports submitted on GitHub and assign labels to each issue accordingly. We empirically evaluated the tool's prediction performance on about 30,000 GitHub issues. Our results show that the Ticket Tagger can identify the correct labels to assign to GitHub issues with reasonably high effectiveness. Considering these results and the fact that the tool is designed to be easily integrated in the GitHub issue management process, Ticket Tagger consists in a useful solution for developers. (C) 2020 Elsevier B.V. All rights reserved.
更多查看译文
关键词
Software maintenance and evolution, Issue reports management, Labeling unstructured data
AI 理解论文
溯源树
样例
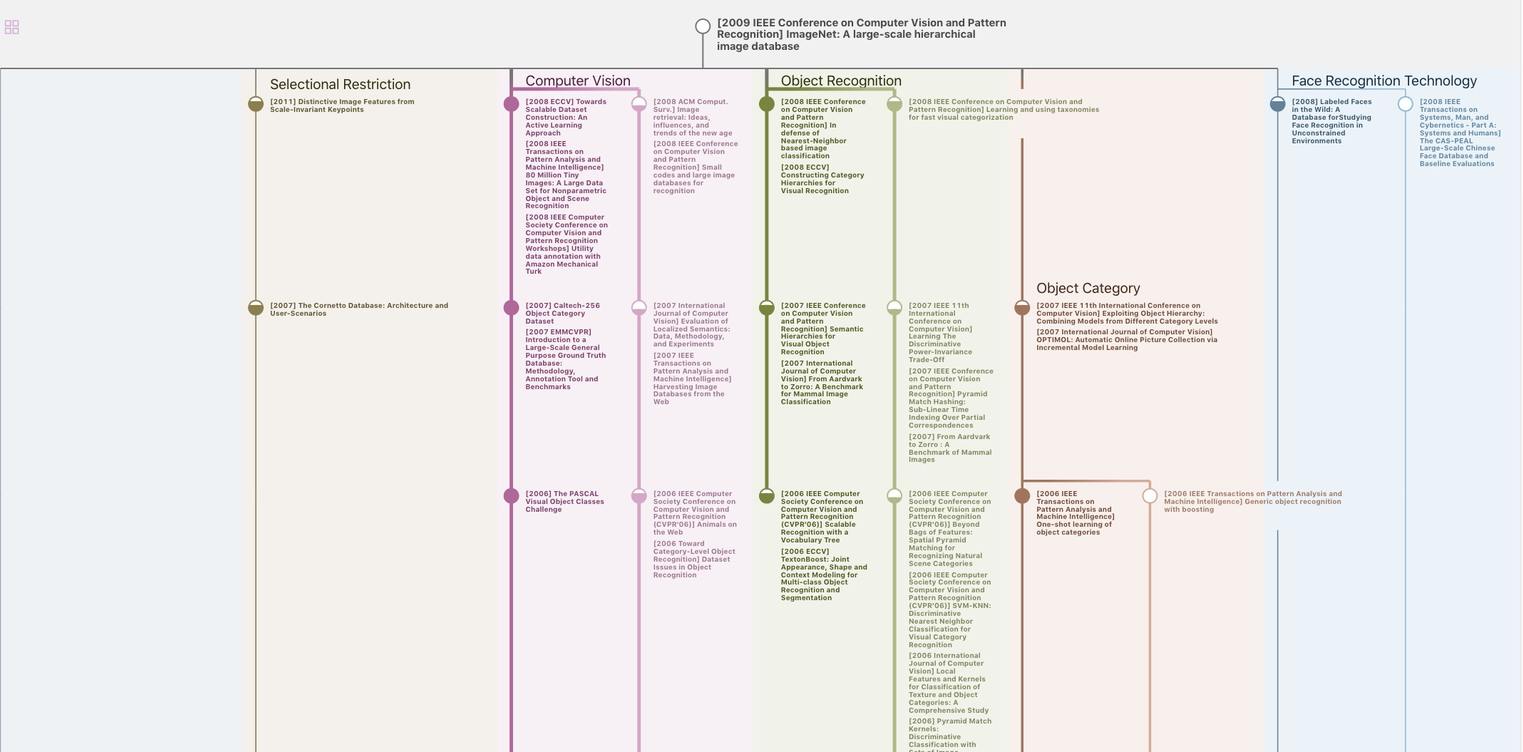
生成溯源树,研究论文发展脉络
Chat Paper
正在生成论文摘要