Optimal DNN Architecture Search Using Bayesian Optimization Hyperband for Arrhythmia Detection
IEEE Wireless Power Transfer Conference(2020)
关键词
electrocardiogram,deep neural network,arrhythmia,Bayesian optimization hyperband
AI 理解论文
溯源树
样例
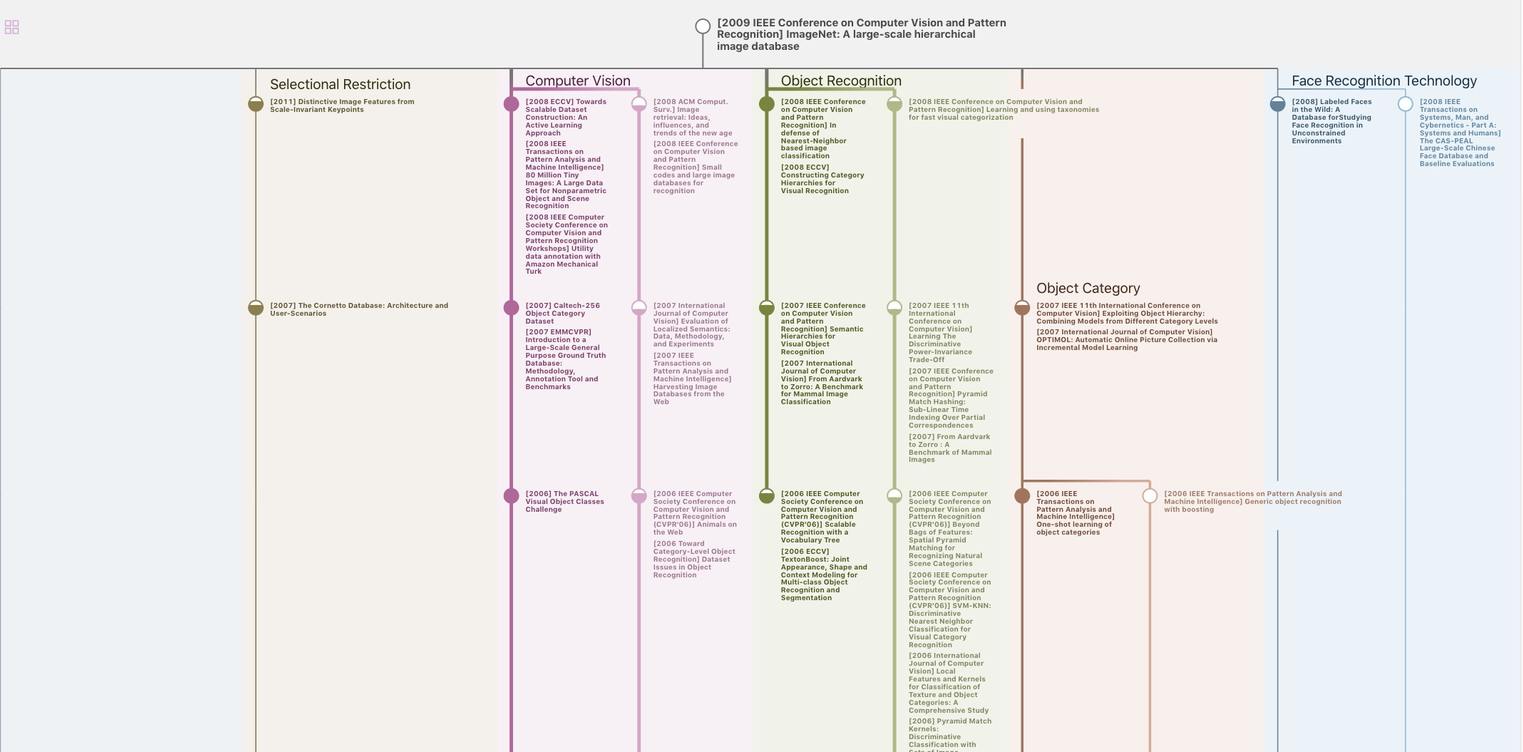
生成溯源树,研究论文发展脉络
Chat Paper
正在生成论文摘要