BBAS: Towards large scale effective ensemble adversarial attacks against deep neural network learning
Information Sciences(2021)
摘要
Recent decades have witnessed rapid development of deep neural networks (DNN). As DNN learning is becoming more and more important to numerous intelligent system, ranging from self driving car to video surveillance system, significant research efforts have been devoted to explore how to improve DNN model’s robustness and reliability against adversarial example attacks. Distinguish from previous study, we address the problem of adversarial training with ensemble based approach and propose a novel boosting based black-box attack scheme call BBAS to facilitate high diverse adversarial example generation. BBAS not only separates example generation from the settings of the trained model but also enhance the diversity of perturbation over class distribution through seamless integration of stratified sampling and ensemble adversarial training. This leads to reliable and effective training example selection. To validate and evaluate the scheme from different perspectives, a set of comprehensive tests have been carried out based on two large open data sets. Experimental results demonstrate the superiority of our method in terms of effectiveness.
更多查看译文
关键词
Black-box attack,Adversarial,Robustness,Boosting
AI 理解论文
溯源树
样例
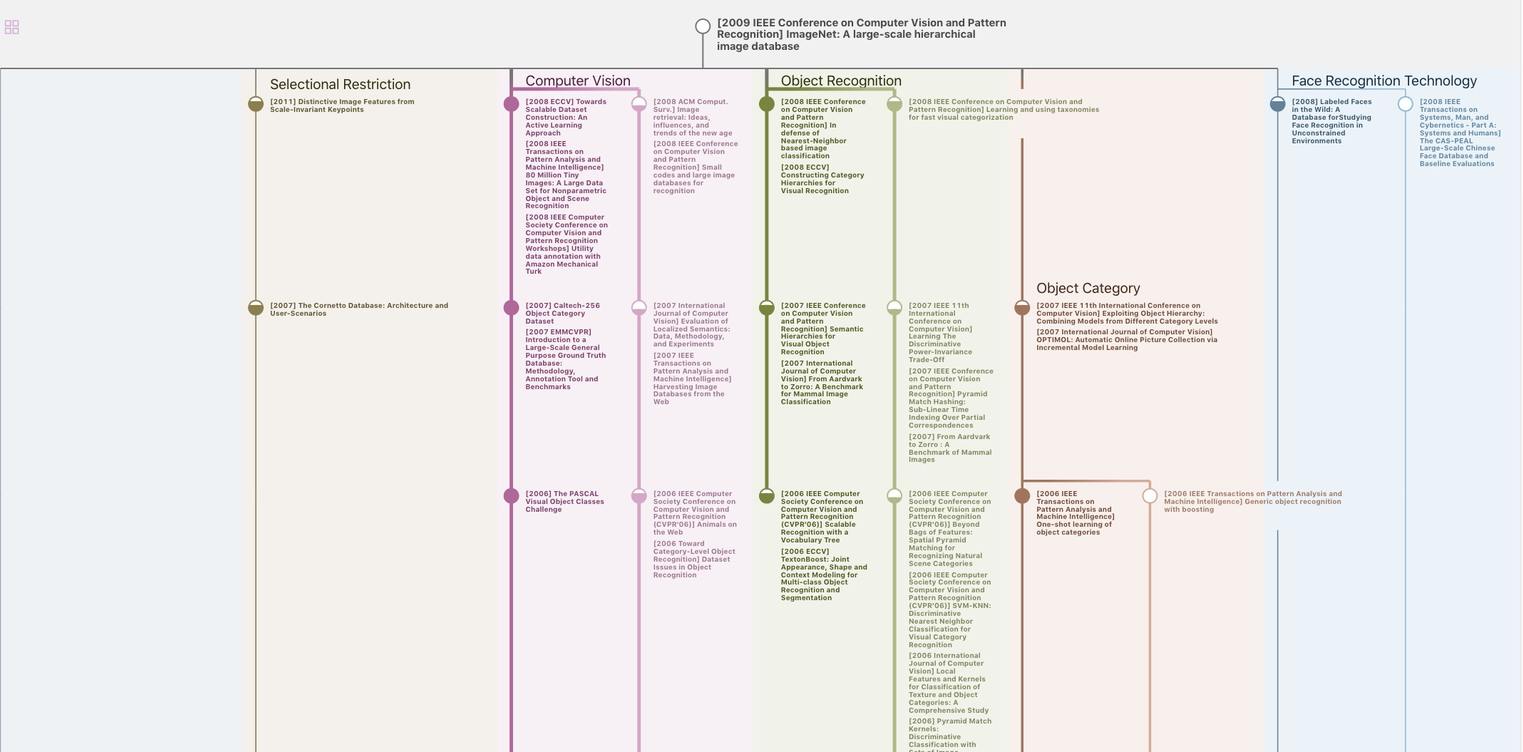
生成溯源树,研究论文发展脉络
Chat Paper
正在生成论文摘要