Deep Learning in Forest Structural Parameter Estimation Using Airborne LiDAR Data
IEEE JOURNAL OF SELECTED TOPICS IN APPLIED EARTH OBSERVATIONS AND REMOTE SENSING(2021)
摘要
Accurately estimating and mapping forest structural parameters are essential for monitoring forest resources and understanding ecological processes. The novel deep learning algorithm has the potential to be a promising approach to improve the estimation accuracy while combining with advanced remote sensing technology. Airborne light detection and ranging (LiDAR) has the preferable capability to characterize 3-D canopy structure and estimate forest structural parameters. In this study, we developed a deep learning-based algorithm (Deep-RBN) that combined the fully connected network (FCN) deep learning algorithm with the optimized radial basis neural network (RBN) algorithm for forest structural parameter estimation using airborne LiDAR data. The multiple iterations were used to constantly update the internal weights to achieve the optimized accuracy of model fitting, and the optimized RBN algorithm was developed for the limited training sets. We assessed the efficiency and capability of the Deep-RBN in the estimation of forest structural parameters in a subtropical planted forest of southern China, by comparing the traditional FCN algorithm and multiple linear regression. We found that Deep-RBN had the strongest capability in estimates of forest structural parameters (R
2
= 0.67-0.86, rRMSE = 6.95%-20.34%). The sensitivity analysis of the key hyperparameters of Deep-RBN algorithm showed that the learning rate is one of the most important parameters that influence the performance of predictive models, and while its value equal is to 0.001, the predictive models had the highest accuracy (mean DBH: RMSE = 1.01, mean height: RMSE = 1.45, volume: RMSE = 26.49, stem density: RMSE = 121.06). With the increase of training samples added in Deep-RBN model, the predictive models performed better; however, no significant improvements of accuracy were observed while the number of training set is larger than 80. This study demonstrates the benefits of jointly using the Deep-RBN algorithm and airborne LiDAR data to improve the accuracy of forest structural parameter estimation and mapping, which provides a promising methodology for sustainable forest resources monitoring.
更多查看译文
关键词
Deep learning, forest structural parameters, hyperparameter, LiDAR, volume distribution
AI 理解论文
溯源树
样例
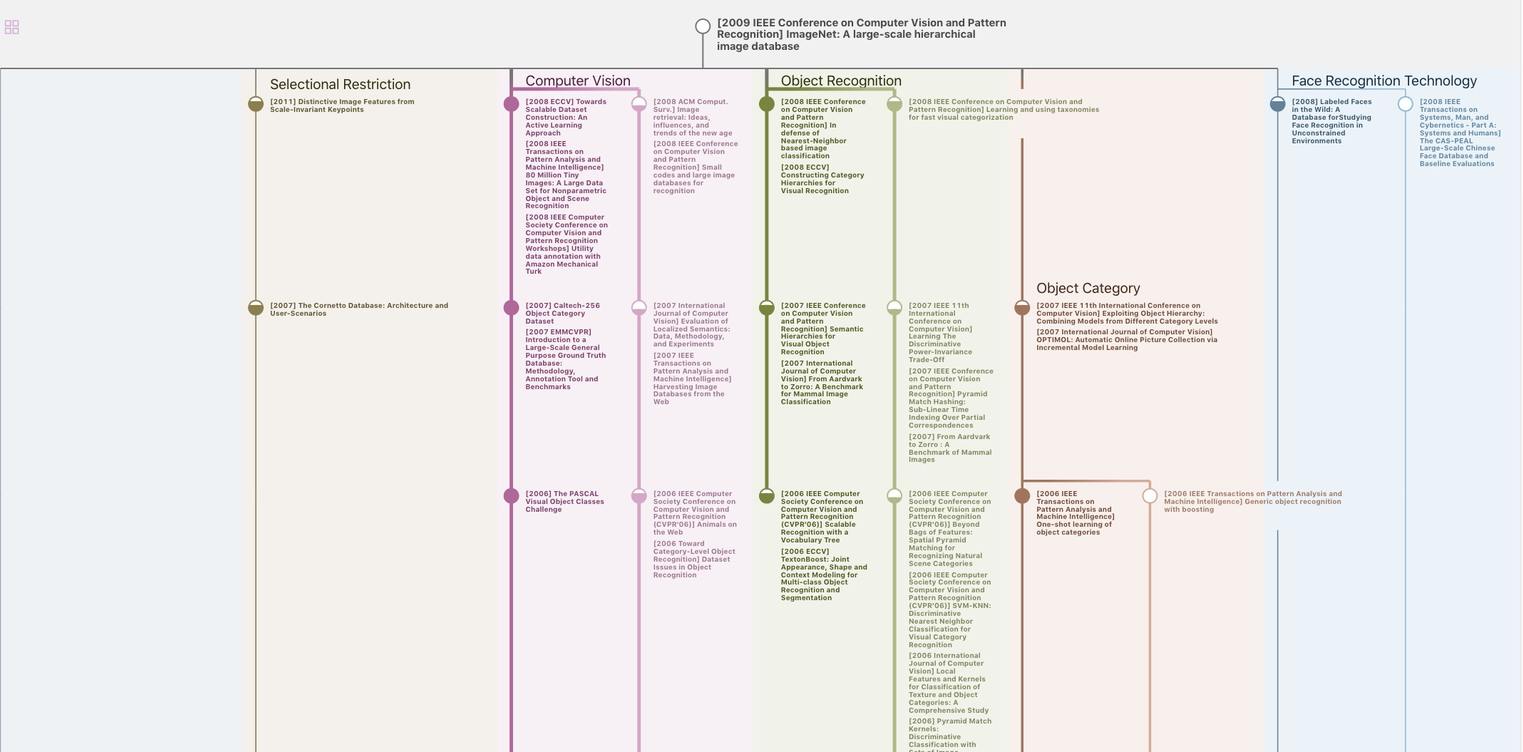
生成溯源树,研究论文发展脉络
Chat Paper
正在生成论文摘要