Capturing Spatio-Temporal Dependencies in the Probabilistic Forecasting of Distribution Locational Marginal Prices
IEEE Transactions on Smart Grid(2021)
Abstract
This article presents a new spatio-temporal framework for the day-ahead probabilistic forecasting of Distribution Locational Marginal Prices (DLMPs). The approach relies on a recurrent neural network, whose architecture is enriched by introducing a deep bidirectional variant designed to capture the complex time dynamics in multi-step forecasts. In order to account for nodal price differentiation (...
MoreTranslated text
Key words
Predictive models,Tools,Forecasting,Low voltage,Computer architecture,Data models,Correlation
AI Read Science
Must-Reading Tree
Example
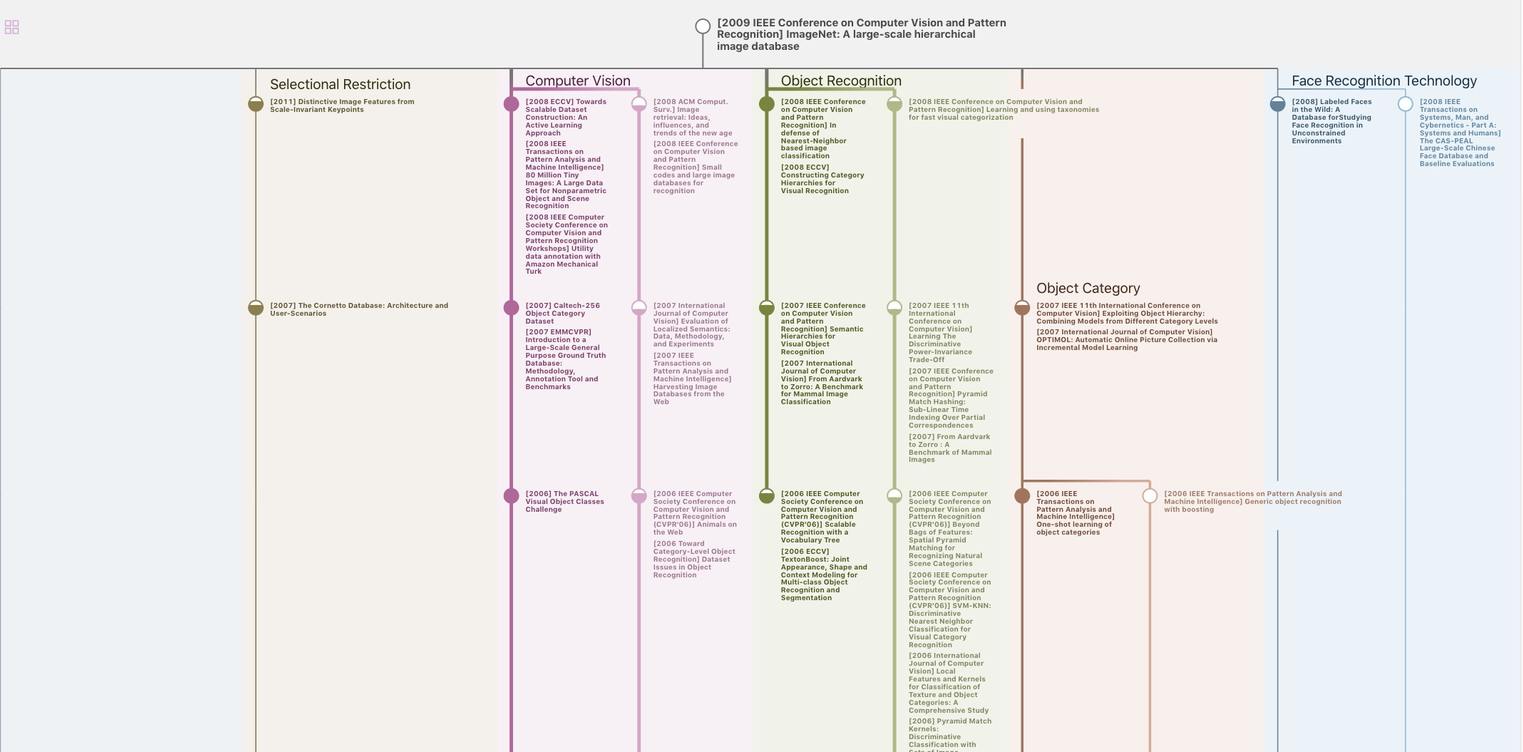
Generate MRT to find the research sequence of this paper
Chat Paper
Summary is being generated by the instructions you defined