Data-driven Symbolic Regression for Identification of Nonlinear Dynamics in Power Systems
power and energy society general meeting(2020)
摘要
The paper describes a data-driven system identification method tailored to power systems and demonstrated on models of synchronous generators (SGs). In this work, we extend the recent sparse identification of nonlinear dynamics (SINDy) modeling procedure to include the effects of exogenous signals and nonlinear trigonometric terms in the library of elements. We show that the resulting framework requires fairly little in terms of data, and is computationally efficient and robust to noise, making it a viable candidate for online identification in response to rapid system changes. The proposed method also shows improved performance over linear data-driven modeling. While the proposed procedure is illustrated on a SG example in a multi-machine benchmark, it is directly applicable to the identification of other system components (e.g., dynamic loads) in large power systems.
更多查看译文
关键词
Power system, Dynamic model, System identification, Nonlinear dynamics, Sparse model
AI 理解论文
溯源树
样例
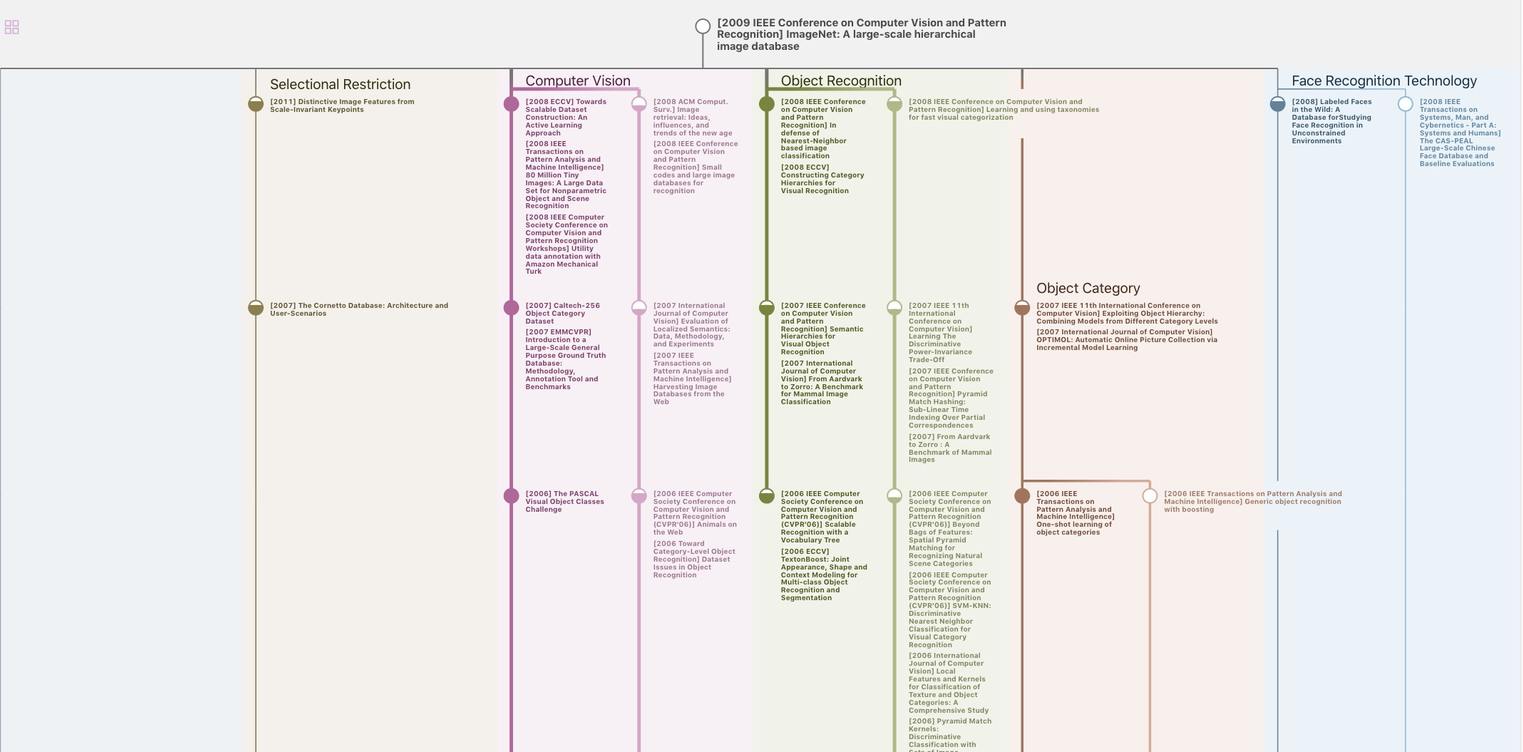
生成溯源树,研究论文发展脉络
Chat Paper
正在生成论文摘要