Machine Learning Method For Predicting The Influence Of Scanning Parameters On Random Measurement Error
MEASUREMENT SCIENCE AND TECHNOLOGY(2021)
Abstract
Measurements of technical objects can be done with contact and non-contact approaches. Contact methods are accurate but slow. On the other hand, non-contact methods deliver rapid point acquisition and are increasingly being used as their precision mounts. However, multiple scanning parameters such as the incident angle, object colour and scanning distance influence the measurement error and uncertainty when capturing the geometry of the object. With the aim of creating a generalised model that considers the influence of the aforementioned scanning parameters with satisfactory accuracy, a model for predicting the random measurement error based on machine learning (ML) is proposed in this study. Data acquired from measurements with varying scanning distances, incident angles and surface colours were used to train ML models. The tested ML methods included linear regression, support vector machine, neural network, k-nearest neighbour, AdaBoost and random forest. The best-performing trained model was the random forest, with a standard deviation of relative differences of 1.46% for the case of red surfaces, and 5.2% for the case of an arbitrarily coloured surface, which is comparable to results achieved with model-based methods. The trained models and the data are available online.
MoreTranslated text
Key words
scanning parameters, machine learning, random measurement error
AI Read Science
Must-Reading Tree
Example
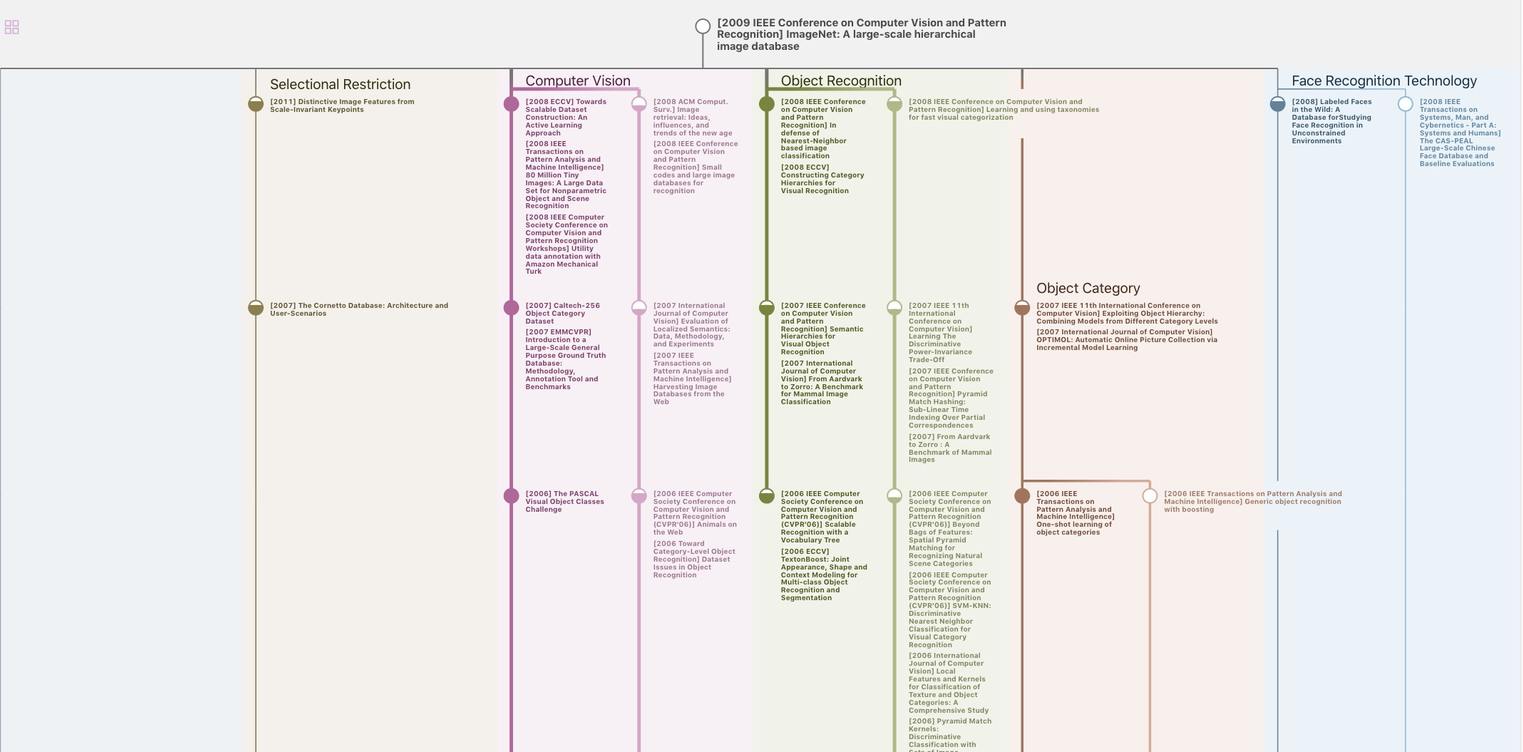
Generate MRT to find the research sequence of this paper
Chat Paper
Summary is being generated by the instructions you defined