Sequence-To-Sequence Deep Neural Network With Spatio-Spectro And Temporal Features For Motor Imagery Classification
BIOCYBERNETICS AND BIOMEDICAL ENGINEERING(2021)
摘要
Electroencephalography (EEG) is a method of the brain-computer interface (BCI) that measures brain activities. EEG is a method of (non-)invasive recording of the electrical activity of the brain. This can be used to build BCIs. From the last decade, EEG has grasped researchers' attention to distinguish human activities. However, temporal information has rarely been retained to incorporate temporal information for multi-class (more than two classes) motor imagery classification. This research proposes a long-short-term-memory-based deep learning model to learn the hidden sequential patterns. Two types of features are used to feed the proposed model, including Fourier Transform Energy Maps (FTEMs) and Common Spatial Patterns (CSPs) filters. Multiple experiments have been conducted on a publicly available dataset. Extraction of spatial and spectro-temporal features using CSP filters and FTEM allow the sequence-to-sequence based proposed model to learn the hidden sequential features. The proposed method is trained, evaluated, and optimized for a publicly available benchmark data set and resulted in 0.81 mean kappa value. Obtained results depict the model robustness for the artifacts and suitable for real-life applications with comparable classification accuracy. The code and findings will be available at https://github.com/waseemabbaas/Motor-Imagery-Classification.git.(c) 2020 Published by Elsevier B.V. on behalf of Nalecz Institute of Biocybernetics and Biomedical Engineering of the Polish Academy of Sciences.
更多查看译文
关键词
Deep learning, Convolutional neural network, Long-short-term-memory, Electroencephalography, Motor imagery
AI 理解论文
溯源树
样例
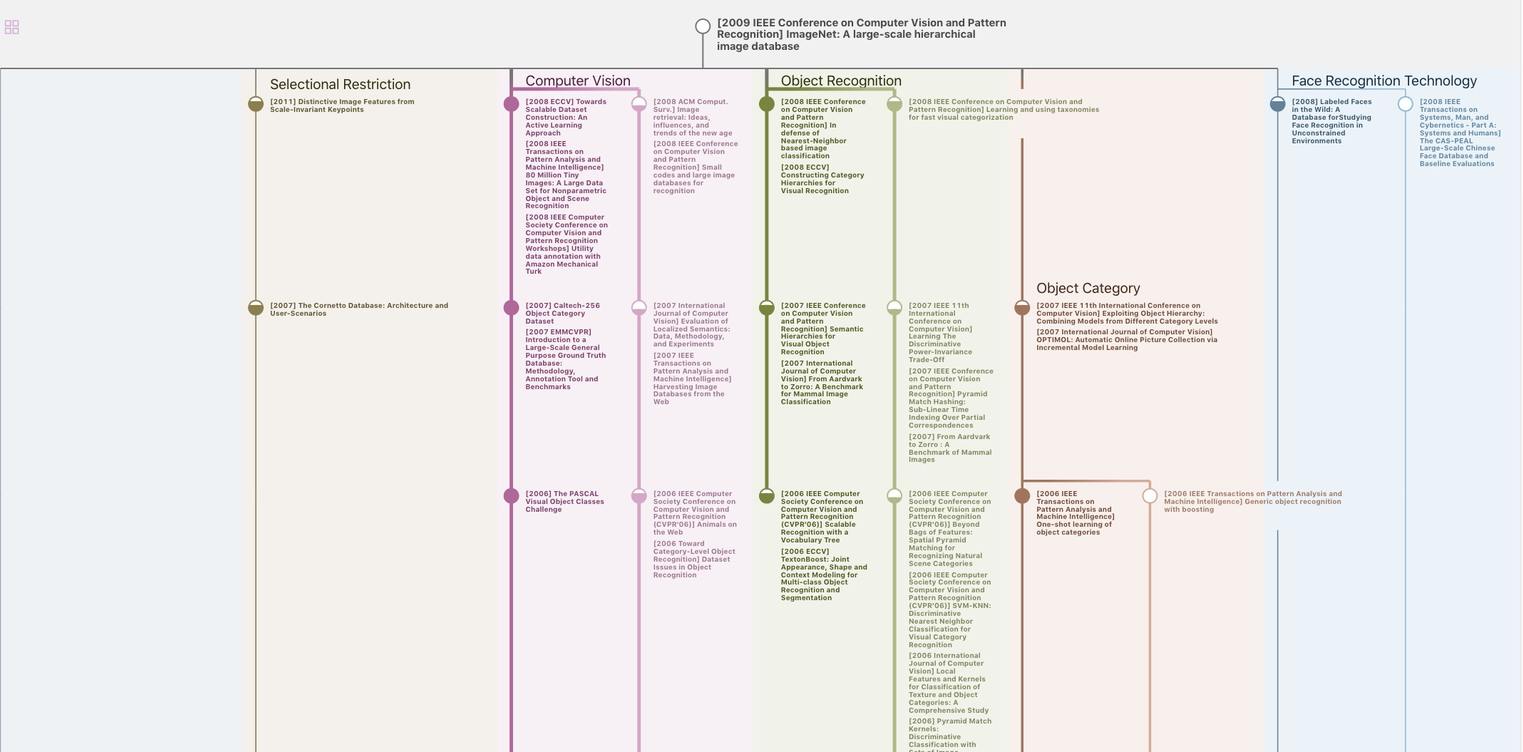
生成溯源树,研究论文发展脉络
Chat Paper
正在生成论文摘要