Combating Selection Biases in Recommender Systems with a Few Unbiased Ratings
WSDM(2021)
摘要
ABSTRACTRecommendation datasets are prone to selection biases due to self-selection behavior of users and item selection process of systems. This makes explicitly combating selection biases an essential problem in training recommender systems. Most previous studies assume no unbiased data available for training. We relax this assumption and assume that a small subset of training data is unbiased. Then, we propose a novel objective that utilizes the unbiased data to adaptively assign propensity weights to biased training ratings. This objective, combined with unbiased performance estimators, alleviates the effects of selection biases on the training of recommender systems. To optimize the objective, we propose an efficient algorithm that minimizes the variance of propensity estimates for better generalized recommender systems. Extensive experiments on two real-world datasets confirm the advantages of our approach in significantly reducing both the error of rating prediction and the variance of propensity estimation.
更多查看译文
关键词
recommender systems,selection biases
AI 理解论文
溯源树
样例
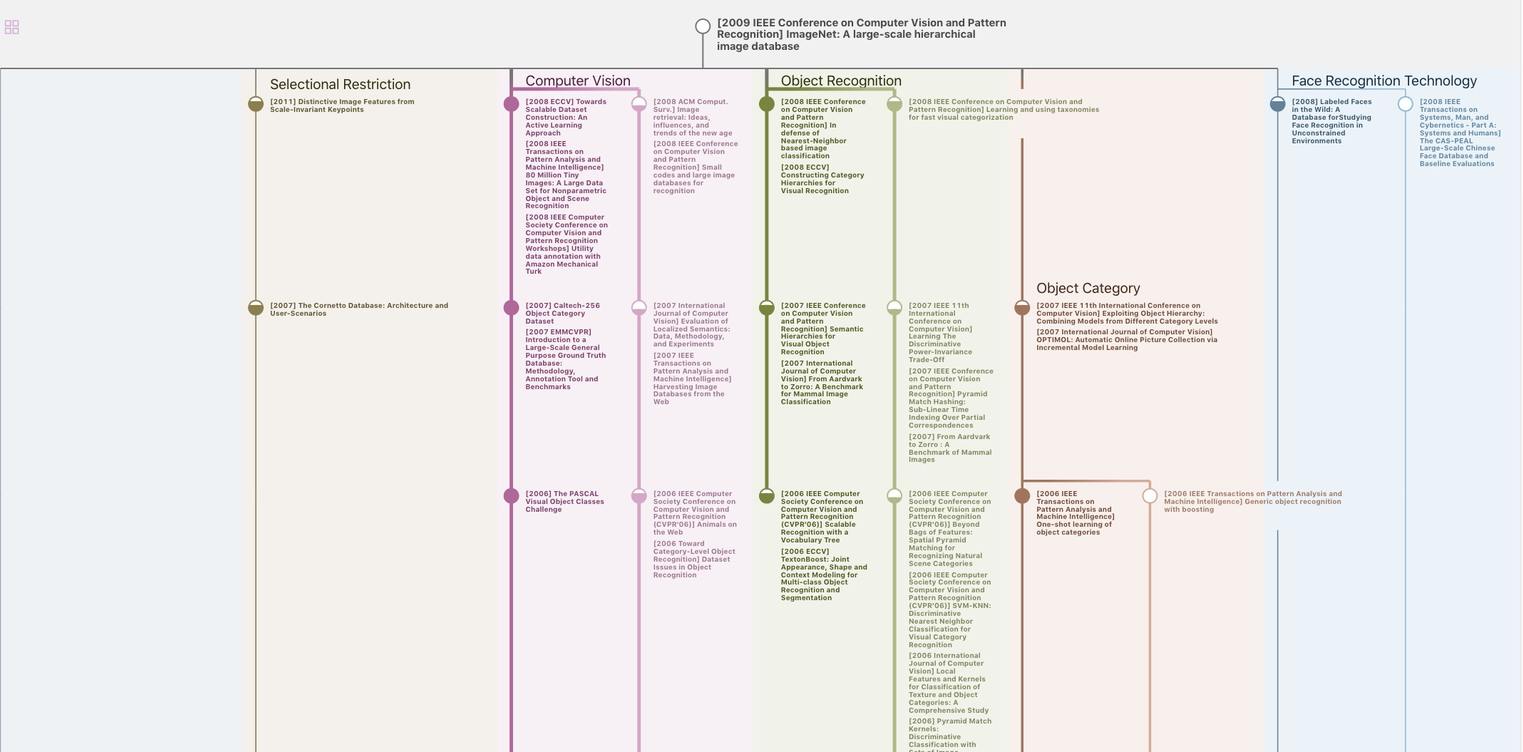
生成溯源树,研究论文发展脉络
Chat Paper
正在生成论文摘要