Learning Graph Topology Representation with Attention Networks
2020 IEEE International Conference on Visual Communications and Image Processing (VCIP)(2020)
Abstract
Contextualized neural language models have gained much attention in Information Retrieval (IR) with its ability to achieve better word understanding by capturing contextual structure on sentence level. However, to understand a document better, it is necessary to involve contextual structure from document level. Moreover, some words contributes more information to delivering the meaning of a document. Motivated by this, in this paper, we take the advantages of Graph Convolutional Networks (GCN) and Graph Attention Networks (GAN) to model global word-relation structure of a document with attention mechanism to improve context-aware document ranking. We propose to build a graph for a document to model the global contextual structure. The nodes and edges of the graph are constructed from contextual embeddings. We first apply graph convolution on the graph and then use attention networks to explore the influence of more informative words to obtain a new representation. This representation covers both local contextual and global structure information. The experimental results show that our method outperforms the state-of-the- art contextual language models, which demonstrate that incorporating contextual structure is useful for improving document ranking.
MoreTranslated text
Key words
Text Understanding,Contextualized Neural Language Models,Graph Convolution Networks,Graph Attention Networks
AI Read Science
Must-Reading Tree
Example
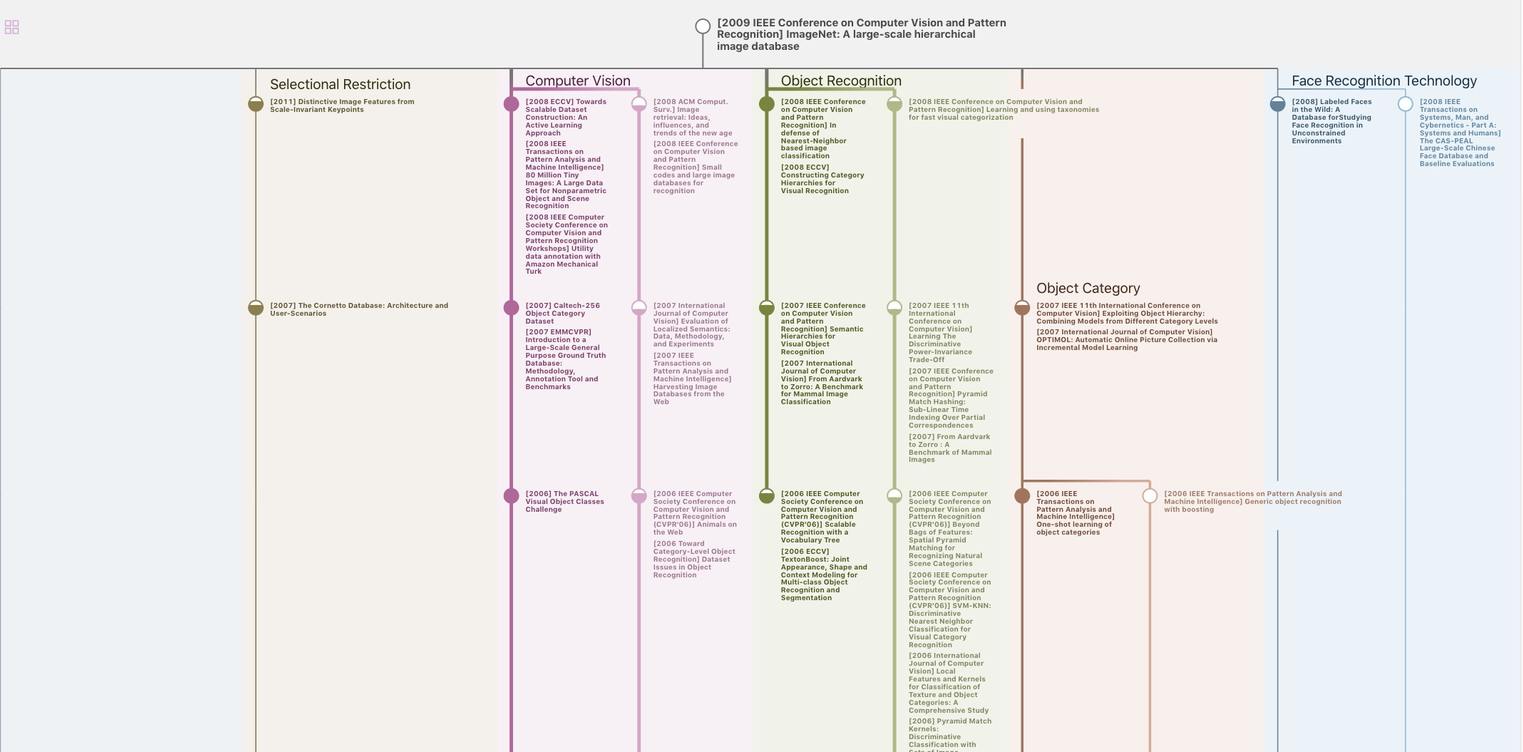
Generate MRT to find the research sequence of this paper
Chat Paper
Summary is being generated by the instructions you defined