3D-CNN Autoencoder for Plenoptic Image Compression
2020 IEEE International Conference on Visual Communications and Image Processing (VCIP)(2020)
摘要
Recently, plenoptic image has attracted great attentions because of its applications in various scenarios. However, high resolution and special pixel distribution structure bring huge challenges to its storage and transmission. In order to adapt compression to the structural characteristic of plenoptic image, in this paper, we propose a Data Structure Adaptive 3D-convolutional(DSA-3D) autoencoder. The DSA-3D autoencoder enables up-sampling and down-samping the sub-aperture sequence along the angular resolution or spatial resolution, thereby avoiding the artifacts caused by directly compressing plenoptic image and achieving better compression efficiency. In addition, we propose a special and efficient Square rearrangement to generate sub-aperture sequence. We compare Square with Zigzag sub-aperture sequence rearrangements, and analyzed the compression efficiency of block image compression and whole image compression. Compared with traditional hybrid encoders HEVC, JPEG2000 and JPEG PLENO(WaSP), the proposed DSA-3D(Square) autoencoder achieves a superior performance in terms of PSNR metrics.
更多查看译文
关键词
Plenoptic image compression,Autoencoder network,3D-CNN
AI 理解论文
溯源树
样例
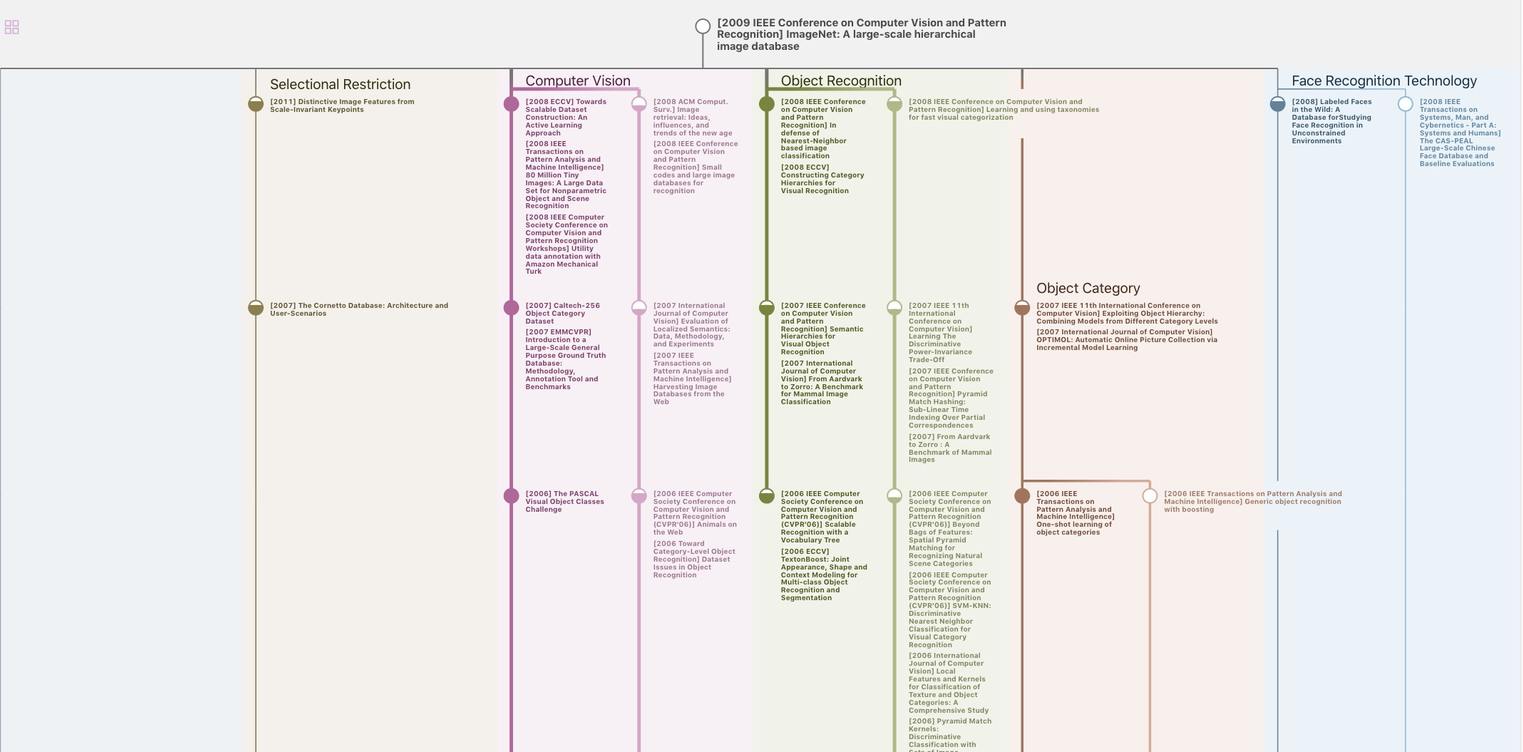
生成溯源树,研究论文发展脉络
Chat Paper
正在生成论文摘要