Research On Change Detection Method Of High-Resolution Remote Sensing Images Based On Subpixel Convolution
IEEE JOURNAL OF SELECTED TOPICS IN APPLIED EARTH OBSERVATIONS AND REMOTE SENSING(2021)
Abstract
Remote sensing image change detection method plays a great role in land cover research, disaster assessment, medical diagnosis, video surveillance, and other fields, so it has attracted wide attention. Based on a small sample dataset from SZTAKI Air-Change Benchmark Set, in order to solve the problem that the deep learning network needs a large number of samples, this work first uses nongenerative sample augmentation method and generative sample augmentation method based on deep convolutional generative adversarial networks, and then, constructs a remote sensing image change detection model based on an improved DeepLabv3+ network. This model can realize end-to-end training and prediction of remote sensing image change detection with subpixel convolution. Finally, Landsat 8, Google Earth, and Onera satellite change detection datasets are used to verify the generalization performance of this network. The experimental results show that the improved network accuracy is 95.1% and the generalization performance is acceptable.
MoreTranslated text
Key words
Change detection, DeepLabv3+, deep convolutional generative adversarial networks (DCGAN), deep learning, subpixel convolution
AI Read Science
Must-Reading Tree
Example
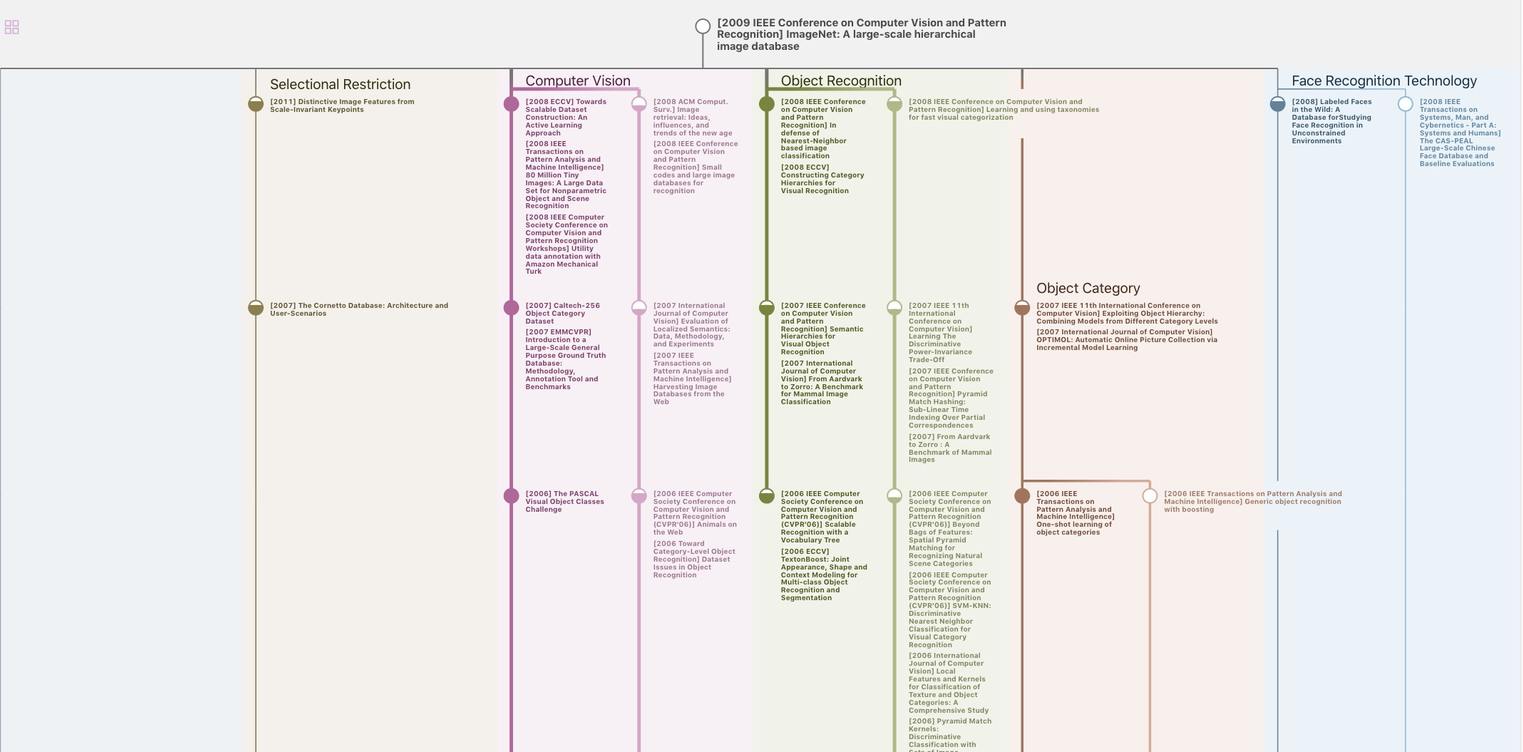
Generate MRT to find the research sequence of this paper
Chat Paper
Summary is being generated by the instructions you defined