Covariate Information Number for Feature Screening in Ultrahigh-Dimensional Supervised Problems
JOURNAL OF THE AMERICAN STATISTICAL ASSOCIATION(2022)
摘要
Contemporary high-throughput experimental and surveying techniques give rise to ultrahigh-dimensional supervised problems with sparse signals; that is, a limited number of observations (n), each with a very large number of covariates (p >> n), only a small share of which is truly associated with the response. In these settings, major concerns on computational burden, algorithmic stability, and statistical accuracy call for substantially reducing the feature space by eliminating redundant covariates before the use of any sophisticated statistical analysis. Along the lines of Pearson's correlation coefficient-based sure independence screening and other model- and correlation-based feature screening methods, we propose a model-free procedure called covariate information number-sure independence screening (CIS). CIS uses a marginal utility connected to the notion of the traditional Fisher information, possesses the sure screening property, and is applicable to any type of response (features) with continuous features (response). Simulations and an application to transcriptomic data on rats reveal the comparative strengths of CIS over some popular feature screening methods. for this article are available online.
更多查看译文
关键词
Affymetrix GeneChip Rat Genome 230 2.0 Array, Fisher information, Model-free, Supervised problems, Sure independence screening, Ultrahigh dimension
AI 理解论文
溯源树
样例
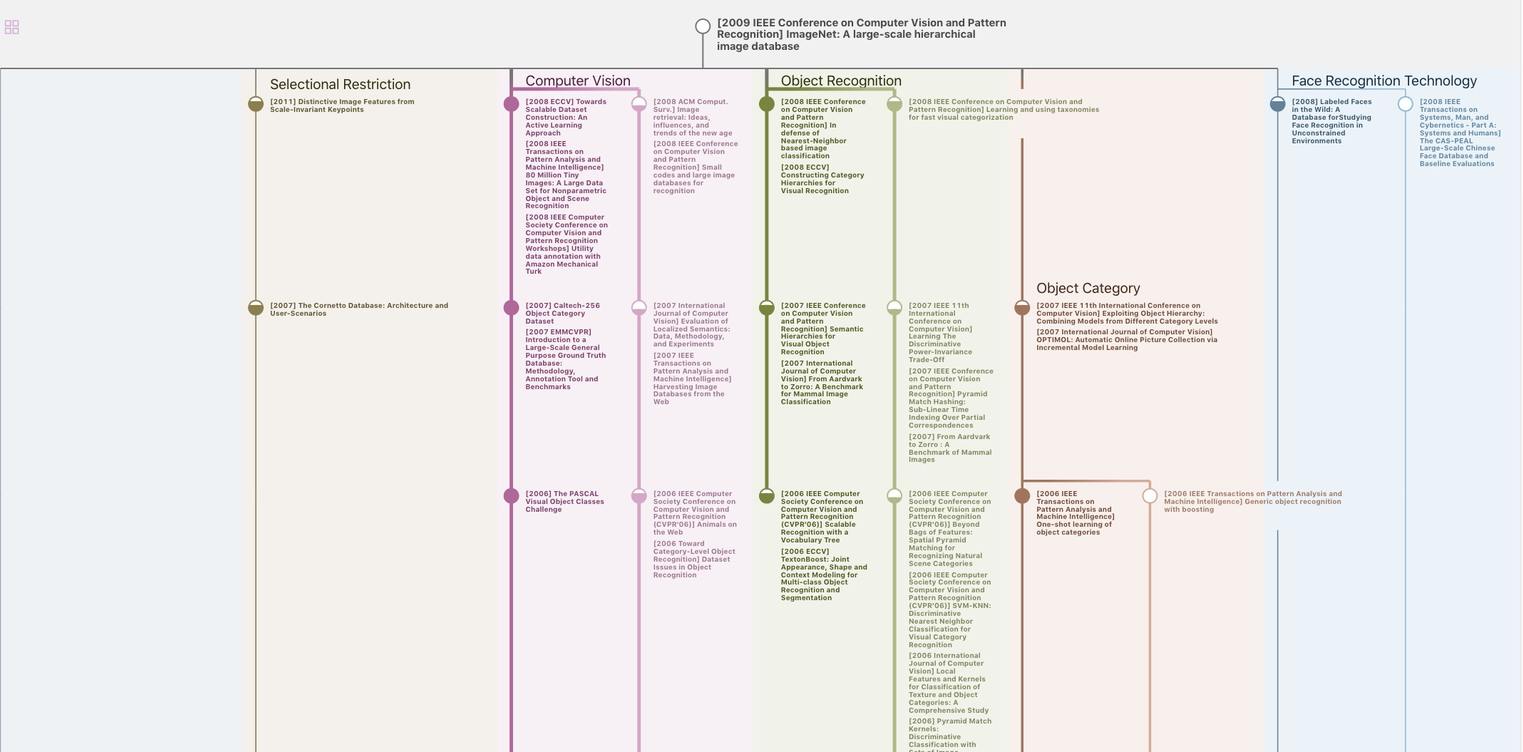
生成溯源树,研究论文发展脉络
Chat Paper
正在生成论文摘要