Assessment Of Urban Infrastructures Exposed To Flood Using Susceptibility Map And Google Earth Engine
IEEE JOURNAL OF SELECTED TOPICS IN APPLIED EARTH OBSERVATIONS AND REMOTE SENSING(2021)
摘要
Acute hydrological natural hazards, such as floods, not only affect the lives and properties of people but also cause severe damage to the critical infrastructures, which needs to be function even in substandard situations. Therefore, it is significant to predict the flood-prone areas for understanding how critical infrastructures are exposed to massive flooding. For this aim, flood-prone areas and their susceptibility are mapped using machine learning techniques including boosted regression tree (BRT) and generalized linear model (GLM) with the help of Sentinel 3 satellite images in Google Earth Engine. The BRT model achieved the highest precision (AUC = 81.10%) in comparison to GLM (AUC = 78.30%) and was utilized for evaluating the flood risk on critical infrastructures. In addition, the flood risk in four large and famous watersheds, namely Zohre, Dorodzan, Tashk-Bakhtegan, and Qareagaj located in Fars Province was analyzed, among which Zohre had the highest risk to flooding. The assessment of flood risk on critical infrastructures, such as hospitals, pharmacies, banks, fire stations, automated teller machines, fuel stations, speed cameras, and mosques located in Shiraz County (capital of Fars Province) disclosed that these structures had high and very high flood hazard. The evaluation of flood risk on schools situated in nine most populated cities of Fars Province was also carried out, which revealed that Shiraz had the maximum percentage (92.98%) and Marvdasht had the least percentage (60.75%) of schools that fall under very high risk. This article aids the decision makers in better planning for flood resilience and risk management.
更多查看译文
关键词
Boosted regression tree (BRT), floods, Google Earth Engine (GEE), urban infrastructures
AI 理解论文
溯源树
样例
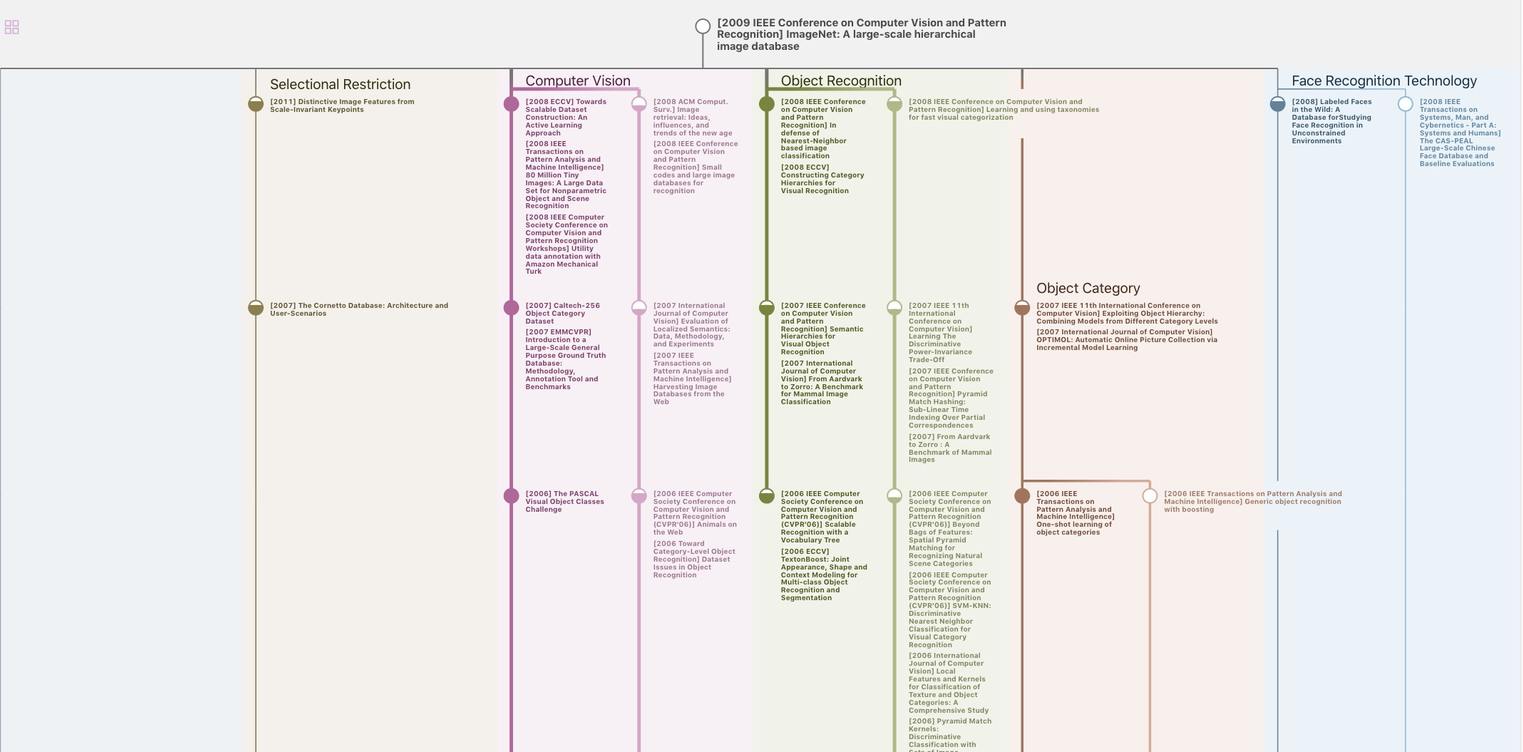
生成溯源树,研究论文发展脉络
Chat Paper
正在生成论文摘要