A Fast-Learning Sparse Antenna Array
2020 IEEE RADAR CONFERENCE (RADARCONF20)(2020)
摘要
Selecting a sparse subset of antennas to obtain high-resolution direction-of-arrival estimates while circumventing the complexity associated with using a large array is critical in many radar applications. Since this subset selection problem is combinatorial, deep learning has been recently proposed as a possible solution for efficiently solving it. However, the bottleneck in this approach is training data generation, which requires an exhaustive search over all possible subarrays. In this paper, we propose an efficient method for generating training data using ideas from submodular optimization. In particular, we use the log-determinant of the Cram ' er-Rao lower bound as our cost function due to its submodular structure. It is then minimized through a greedy optimization approach to determine the best subarray. We provide numerical simulations to validate the performance of the proposed array selection strategy. Our simulations show that the proposed approach is ten times faster in training than an exhaustive search method while providing comparable performance.
更多查看译文
关键词
Cramér-Rao lower bound,submodular optimization,training data generation,deep learning,subset selection problem,radar applications,high-resolution direction-of-arrival estimates,fast-learning sparse antenna array,exhaustive search method,greedy optimization approach
AI 理解论文
溯源树
样例
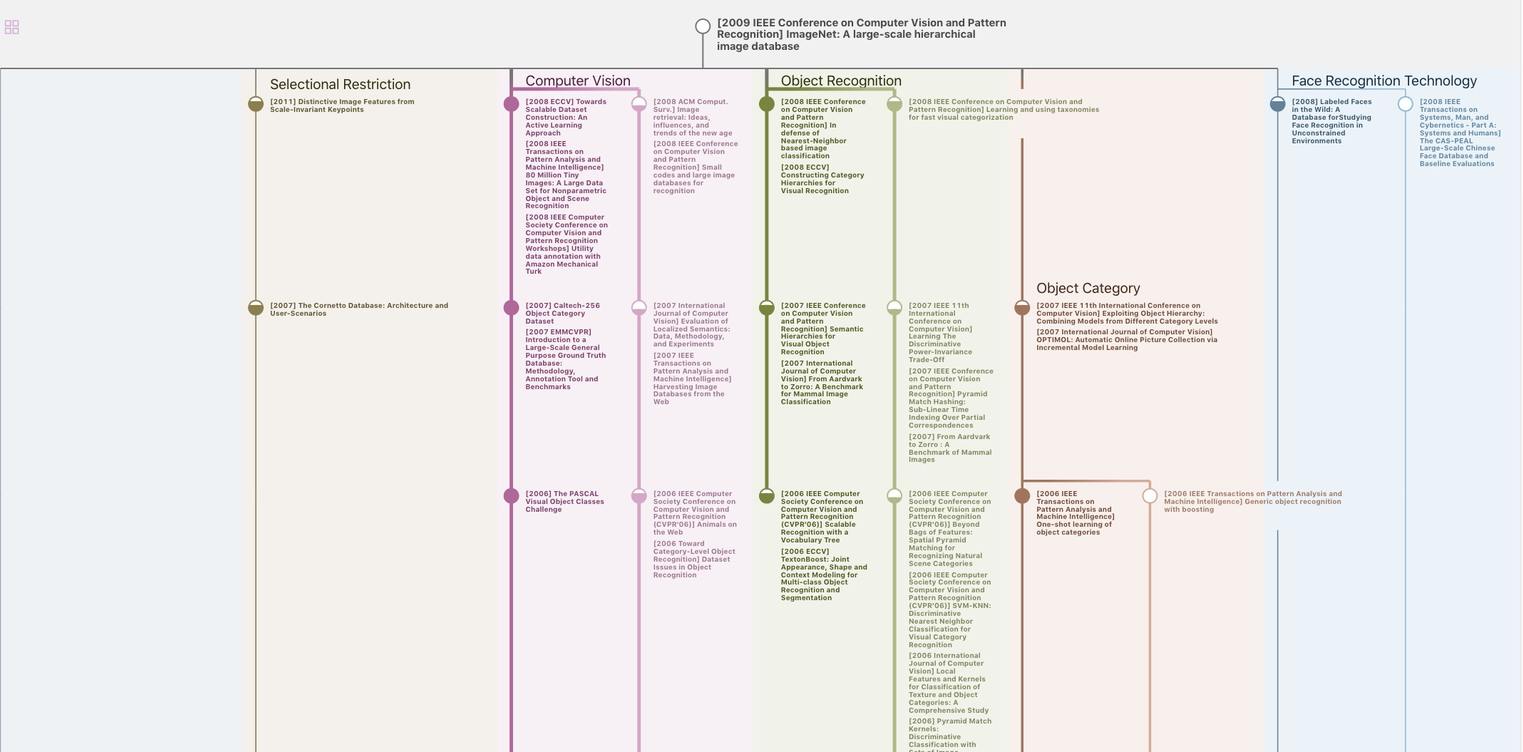
生成溯源树,研究论文发展脉络
Chat Paper
正在生成论文摘要