Early Stuck Pipe Sign Detection With Depth-Domain 3d Convolutional Neural Network Using Actual Drilling Data
SPE JOURNAL(2021)
摘要
A real-time stuck pipe prediction using the deep-learning approach is studied in this paper. Early signs of stuck pipe, hereinafter called stuck, are assumed to show common patterns in the monitored data set, and designing a data clip that well captures these features is critical for efficient prediction. With the valuable input from drilling engineers, we propose a 3D-convolutional neural network (CNN) approach with depth-domain data clip. The clip illustrates depth-domain data in 2D-histogram images with unique abstraction of the time domain. Thirty field well data prepared in multivariate time series are used in this study-20 for training and 10 for validation. The validation data include six stuck incidents, and the 3D-CNN model has successfully detected early signs of stuck in three cases before the occurrence. The portion of the data clip contributing to anomaly detection is indicated by gradient-weighted class activation map (grad-CAM), providing physical explanation of the black box model. We consider such explanation inevitable for the drilling engineers to interpret the signs for rational decision-making.
更多查看译文
AI 理解论文
溯源树
样例
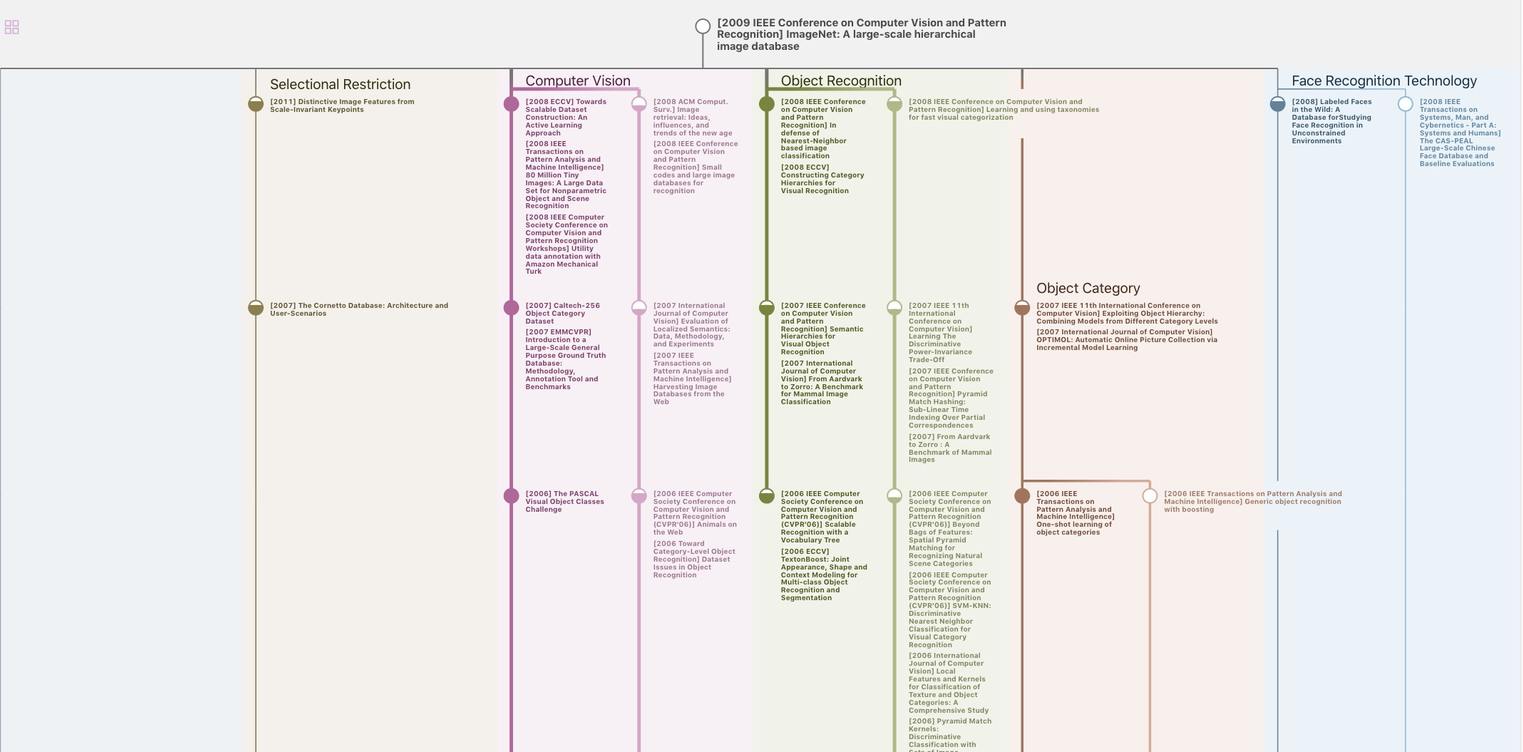
生成溯源树,研究论文发展脉络
Chat Paper
正在生成论文摘要