Applications Of Reinforcement Learning In Energy Systems
RENEWABLE & SUSTAINABLE ENERGY REVIEWS(2021)
Abstract
Energy systems undergo major transitions to facilitate the large-scale penetration of renewable energy technologies and improve efficiencies, leading to the integration of many sectors into the energy system domain. As the complexities in this domain increase, it becomes challenging to control energy flows using existing techniques based on physical models. Moreover, although data-driven models, such as reinforcement learning (RL), have gained considerable attention in many fields, a direct shift into RL is not feasible in the energy domain irrespective of the ongoing complexities. To this end, a top-down approach is used to understand this behavior by reviewing the current state of the art.We classified RL papers in the literature into seven categories based on their area of application. Subsequently, publications under each category were further examined relative to problem diversity, RL technique employed, performance improvement (compared with other white and gray box models), verification, and reproducibility; many of the articles reported a 10-20% performance improvement with the use of RL. In most studies, however, deep learning techniques and state-of-the-art actor-critic methods (e.g., twin delayed deep deterministic policy gradient and soft actor-critic) were not applied. This has remarkably hindered performance improvements and problems related to complex energy flows have not been considered. Approximately half of the publications reported the use of Q-learning. Furthermore, despite the availability of historical data in the energy system domain, batch RL algorithms have not been exploited. Emerging multi-agent RL applications may be considered as a positive development that can enable the management of complex interactions among multiple parties. Most studies lack proper benchmarking compared to model-based approaches or gray-box models, and a majority cover energy dispatch problems and building energy management. Although RL can adequately solve problems that are considerably integrated in several sectors, only a limited number of publications have discussed its broad application. The present study clearly demonstrates that even without the full utilization of RL capacity, this technique has a considerable potential in resolving the continuously increasing complexity within the energy system domain.
MoreTranslated text
Key words
Energy systems, Reinforcement learning, Renewable energy, Building energy, Machine learning
AI Read Science
Must-Reading Tree
Example
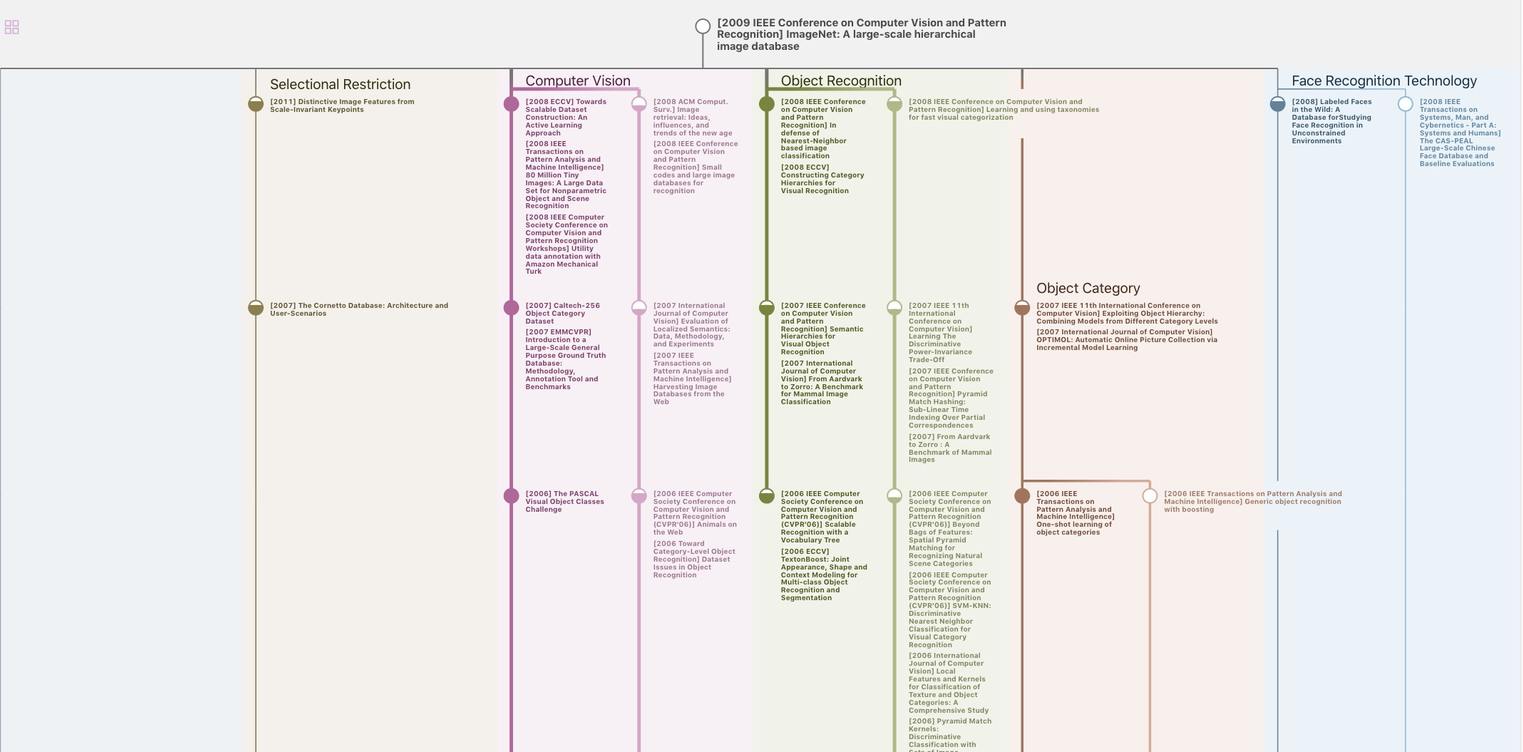
Generate MRT to find the research sequence of this paper
Chat Paper
Summary is being generated by the instructions you defined