Preference-Aware Dynamic Bitrate Adaptation For Mobile Short-Form Video Feed Streaming
IEEE ACCESS(2020)
摘要
Short-form video feed becomes increasingly popular among younger generations, where mobile users watch videos of a few seconds one-by-one in a session or a pre-defined list. The common solution to improve the quality of experience (QoE) for short-video feed is to implement dynamic adaptive streaming strategies to decide bitrate for each video. However, legacy bitrate adaptation strategies fail to differentiate the weights of videos in the list according to the user preferences, leading to the bandwidth waste on downloading those video content skipped immediately by the users without being displayed. In this paper, we propose RecDASH, which consists of an attention-based user modeling module leveraging advanced recommendation algorithms and a reinforcement learning (RL) based bitrate adaptation module. Specifically, we use gated recurrent unit (GRU) network with attention mechanism to encode the session into a representation vector. Then, RL module combines the representation of session and other observations within playback, and yields the appropriate bitrate for the next short-form video for optimizing a given QoE objective. The low space and time complexity of the model enables it to be easily deployed on mobile devices. Trace-driven emulations verify the efficiency of RecDASH compared to several state-of-the-art streaming strategies with at least 5%-15% improvement on the video quality under various QoE objectives.
更多查看译文
关键词
Streaming media, Bit rate, Feeds, Quality of experience, Bandwidth, Adaptation models, Recommender systems, Bitrate adaptation, short-video feed, list-based recommendation, reinforcement learning
AI 理解论文
溯源树
样例
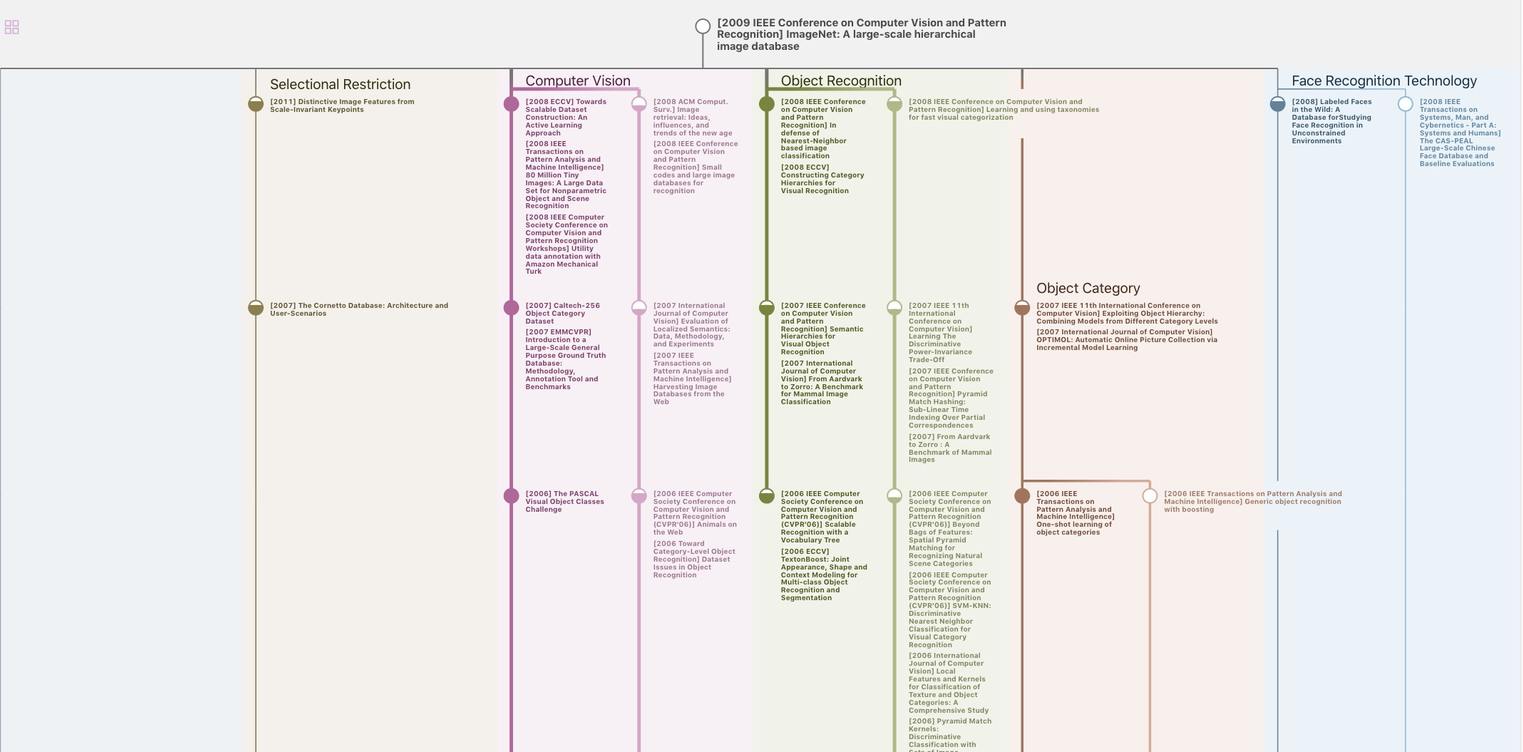
生成溯源树,研究论文发展脉络
Chat Paper
正在生成论文摘要