MeSHProbeNet-P: Improving Large-scale MeSH Indexing with Personalizable MeSH Probes
ACM Transactions on Knowledge Discovery from Data(2021)
摘要
AbstractIndexing biomedical research articles with Medical Subject Headings (MeSH) can greatly facilitate biomedical research and information retrieval. Currently MeSH indexing is performed by human experts. To alleviate the time consumption and monetary cost caused by manual indexing, many automatic MeSH indexing models have been developed, such as MeSHProbeNet, DeepMeSH, and NLM’s official model Medical Text Indexer. In this article, we propose an end-to-end framework, MeSHProbeNet-P, which extends MeSHProbeNet with personalizable MeSH probes. In MeSHProbeNet-P, each MeSH probe carries certain aspects of biomedical knowledge and extracts related information from input articles. MeSHProbeNet-P is able to automatically personalize its MeSH probes for different input articles to ensure that the current MeSH probes best fit the current input article and the most informative features can be extracted from the article. We demonstrate the effectiveness of MeSHProbeNet-P in a real-world large-scale MeSH indexing challenge. MeSHProbeNet-P won the first place in the first batch of Task A in the 2019 BioASQ challenge. The result on the first test set of the challenge is reported in this article. We also provide ablation studies to show the advantages of personalizable MeSH probes.
更多查看译文
关键词
Large-scale, biomedical MeSH indexing, deep learning, attention mechanism
AI 理解论文
溯源树
样例
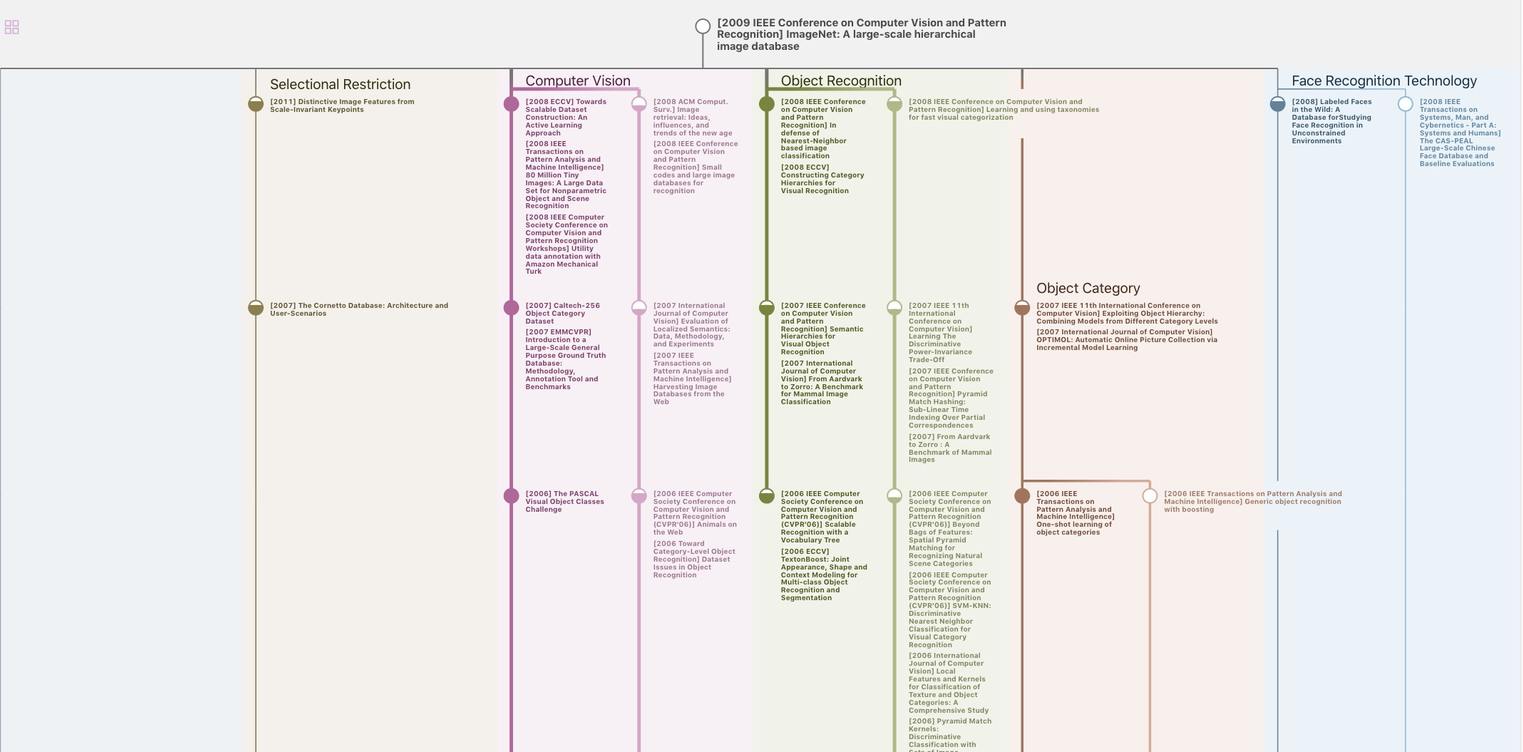
生成溯源树,研究论文发展脉络
Chat Paper
正在生成论文摘要