A Robust Stochastic Approach To Mineral Hyperspectral Analysis For Geometallurgy
MINERALS(2020)
Abstract
Most mining companies have registered important amounts of drill core composite spectra using different acquisition equipment and by following diverse protocols. These companies have used classic spectrography based on the detection of absorption features to perform semi-quantitative mineralogy. This methodology requires ideal laboratory conditions in order to obtain normalized spectra to compare. However, the inherent variability of spectral features-due to environmental conditions and geological context, among others-is unavoidable and needs to be managed. This work presents a novel methodology for geometallurgical sample characterization consisting of a heterogeneous, multi-pixel processing pipeline which addresses the effects of ambient conditions and geological context variability to estimate critical geological and geometallurgical variables. It relies on the assumptions that the acquisition of hyperspectral images is an inherently stochastic process and that ore sample information is deployed in the whole spectrum. The proposed framework is basically composed of: (a) a new hyperspectral image segmentation algorithm, (b) a preserving-information dimensionality reduction scheme and (c) a stochastic hierarchical regression model. A set of experiments considering white reference spectral characterization and geometallurgical variable estimation is presented to show promising results for the proposed approach.
MoreTranslated text
Key words
geometallurgical characterization, hyperspectral processing and analysis, hyperspectral clustering, stochastic modeling
AI Read Science
Must-Reading Tree
Example
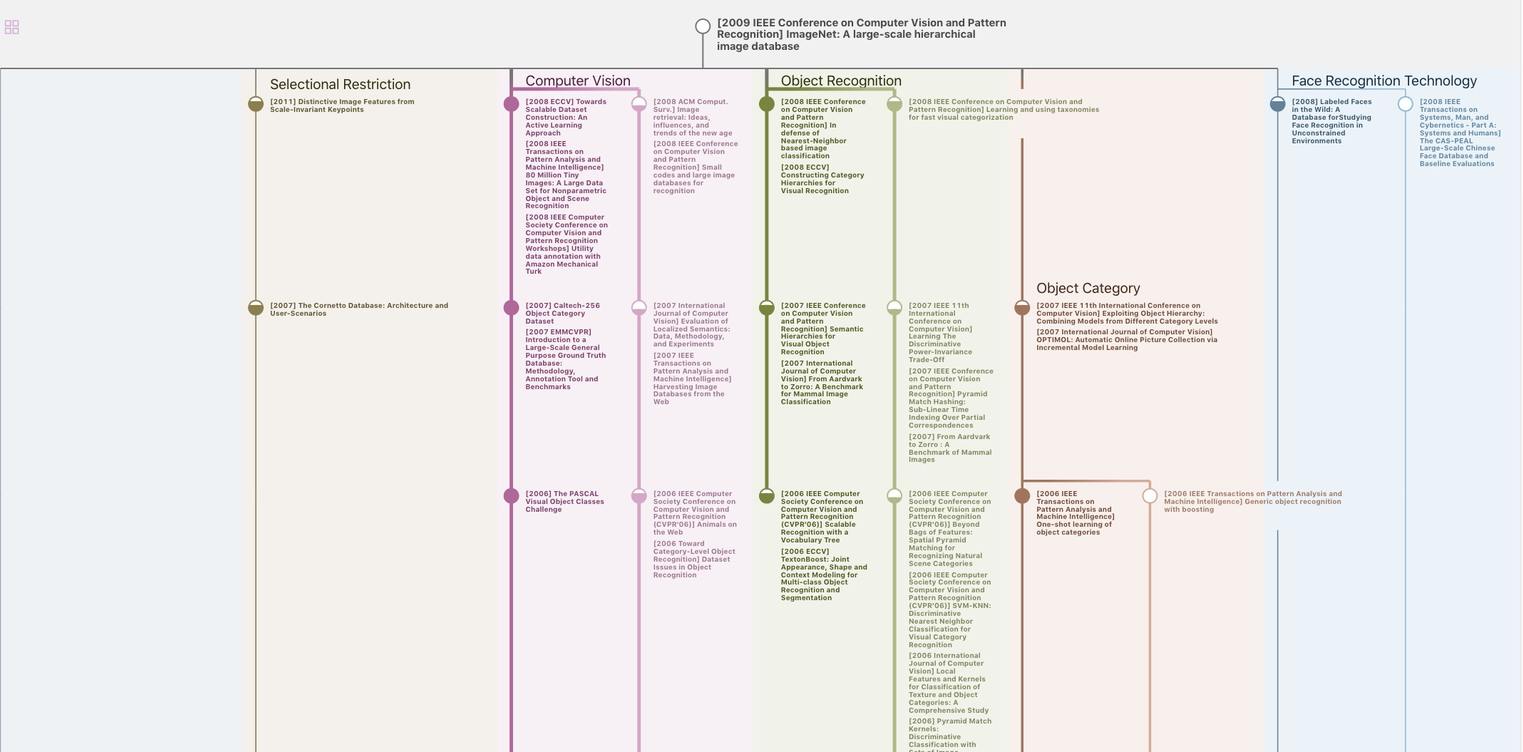
Generate MRT to find the research sequence of this paper
Chat Paper
Summary is being generated by the instructions you defined