Learning a deep network with cross-hierarchy aggregation for crowd counting
KNOWLEDGE-BASED SYSTEMS(2021)
摘要
Crowd counting, a significant but challenging task in computer vision, aims at estimating the number of people in an image or video. Recent methods for crowd counting have obtained promising performance due to deep neural networks but most of them ignore the abundant conducive information in hierarchical features. In this paper, a novel Cross-Hierarchy Aggregation Network (CHANet) is proposed to exploit multi-hierarchy information in the crowd features from each hierarchy and aggregate cross-hierarchy features to generate reasonable density maps. Firstly, we propose a CHA module to fully extract local hierarchical features and capture maximum information of the crowd features. The CHA module combines residual and dense connections without over-assigning parameters for feature reuse. Then, we utilize the global hierarchical features from the shallow hierarchies to obtain a more powerful representation ability with a global residual connection. Experimental evaluations on four publicly available crowd counting datasets (ShanghaiTech, UCF-QNRF, WorldExpo'10, and Beijing BRT) demonstrate that the proposed CHANet achieves superior performance compared to other state-of-the-art methods. (C) 2020 Elsevier B.V. All rights reserved.
更多查看译文
关键词
Crowd counting,Cross-hierarchy aggregation,Density maps
AI 理解论文
溯源树
样例
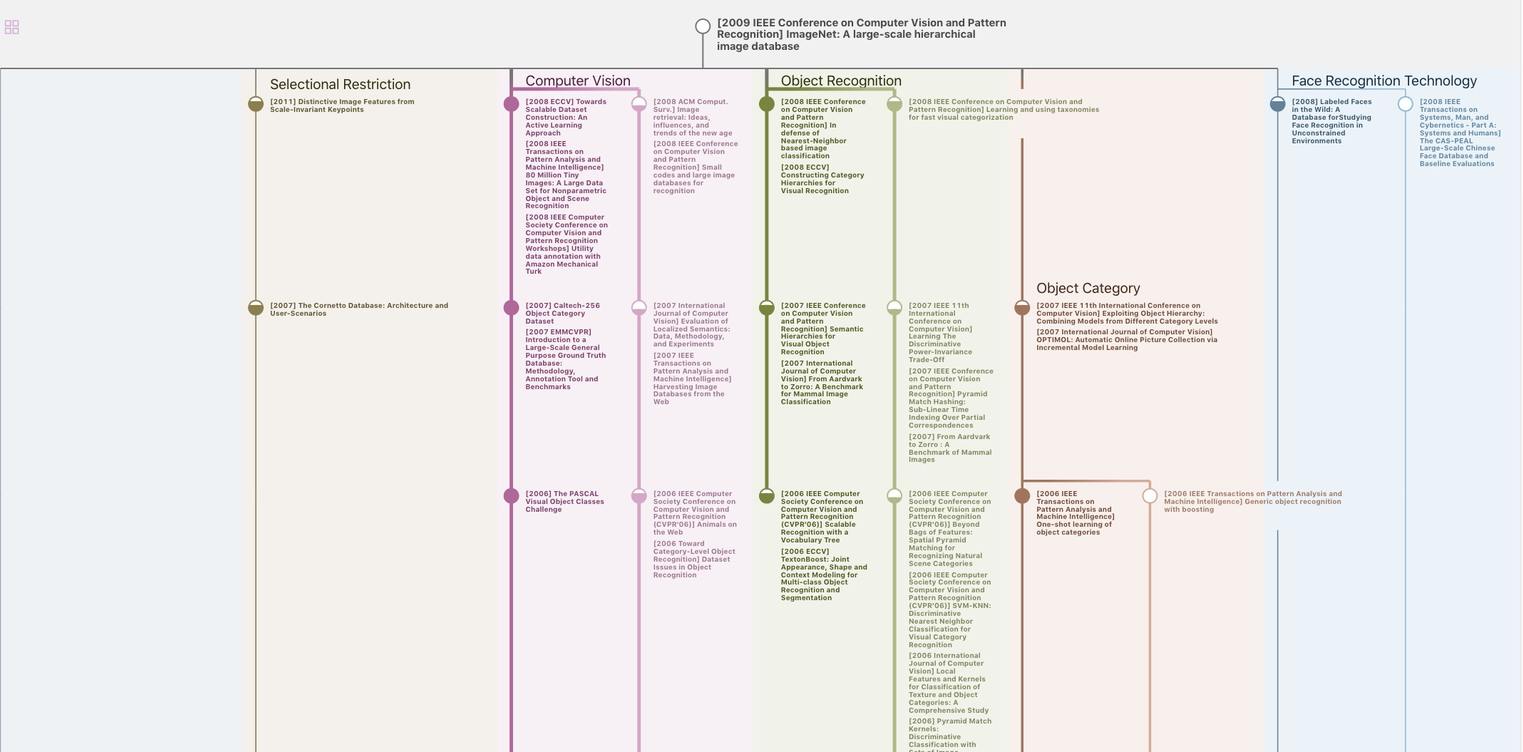
生成溯源树,研究论文发展脉络
Chat Paper
正在生成论文摘要